Create a data product¶
Spatial signatures in Great Britain are released as a data product.
This notebook takes the generated results and turn them into clean outputs.
# we need the master version of dask geopandas that has sjoin and recent dask
!pip install git+https://github.com/geopandas/dask-geopandas.git
import pandas
import dask.dataframe
import dask
import geopandas
import dask_geopandas
dask_geopandas.__version__
'v0.1.0a4+16.gf18ae2c'
Signature geometry¶
We create an output with signature geometry and clear columns.
signatures = geopandas.read_parquet("../../urbangrammar_samba/spatial_signatures/signatures/signatures_combined_levels_orig.pq")
signatures.head()
kmeans10gb | geometry | level2 | signature_type | |
---|---|---|---|---|
0 | 0 | POLYGON Z ((62220.000 798500.000 0.000, 62110.... | 0.0 | 0_0 |
1 | 0 | POLYGON Z ((63507.682 796515.169 0.000, 63471.... | 0.0 | 0_0 |
2 | 0 | POLYGON Z ((65953.174 802246.172 0.000, 65950.... | 0.0 | 0_0 |
3 | 0 | POLYGON Z ((67297.740 803435.800 0.000, 67220.... | 0.0 | 0_0 |
4 | 0 | POLYGON Z ((75760.000 852670.000 0.000, 75700.... | 0.0 | 0_0 |
types = {
"0_0": "Countryside agriculture",
"1_0": "Accessible suburbia",
"3_0": "Open sprawl",
"4_0": "Wild countryside",
"5_0": "Warehouse/Park land",
"6_0": "Gridded residential quarters",
"7_0": "Urban buffer",
"8_0": "Disconnected suburbia",
"2_0": "Dense residential neighbourhoods",
"2_1": "Connected residential neighbourhoods",
"2_2": "Dense urban neighbourhoods",
"9_0": "Local urbanity",
"9_1": "Concentrated urbanity",
"9_2": "Regional urbanity",
"9_4": "Metropolitan urbanity",
"9_5": "Hyper concentrated urbanity",
"9_3": "outlier",
"9_6": "outlier",
"9_7": "outlier",
"9_8": "outlier",
}
signatures["type"] = signatures.signature_type.map(types)
signatures["id"] = range(len(signatures))
signatures.head()
kmeans10gb | geometry | level2 | signature_type | type | id | |
---|---|---|---|---|---|---|
0 | 0 | POLYGON Z ((62220.000 798500.000 0.000, 62110.... | 0.0 | 0_0 | Countryside agriculture | 0 |
1 | 0 | POLYGON Z ((63507.682 796515.169 0.000, 63471.... | 0.0 | 0_0 | Countryside agriculture | 1 |
2 | 0 | POLYGON Z ((65953.174 802246.172 0.000, 65950.... | 0.0 | 0_0 | Countryside agriculture | 2 |
3 | 0 | POLYGON Z ((67297.740 803435.800 0.000, 67220.... | 0.0 | 0_0 | Countryside agriculture | 3 |
4 | 0 | POLYGON Z ((75760.000 852670.000 0.000, 75700.... | 0.0 | 0_0 | Countryside agriculture | 4 |
signatures = signatures[["id", "type", "geometry"]]
signatures
id | type | geometry | |
---|---|---|---|
0 | 0 | Countryside agriculture | POLYGON Z ((62220.000 798500.000 0.000, 62110.... |
1 | 1 | Countryside agriculture | POLYGON Z ((63507.682 796515.169 0.000, 63471.... |
2 | 2 | Countryside agriculture | POLYGON Z ((65953.174 802246.172 0.000, 65950.... |
3 | 3 | Countryside agriculture | POLYGON Z ((67297.740 803435.800 0.000, 67220.... |
4 | 4 | Countryside agriculture | POLYGON Z ((75760.000 852670.000 0.000, 75700.... |
... | ... | ... | ... |
96699 | 96699 | outlier | POLYGON ((323321.005 463795.416, 323319.842 46... |
96700 | 96700 | outlier | POLYGON ((325929.840 1008792.061, 325927.377 1... |
96701 | 96701 | outlier | POLYGON ((337804.770 1013422.583, 337800.122 1... |
96702 | 96702 | outlier | POLYGON ((422304.270 1147826.990, 422296.000 1... |
96703 | 96703 | outlier | POLYGON ((525396.260 439215.480, 525360.920 43... |
96704 rows × 3 columns
Remove Z coordinates¶
Some polygons contain Z coordinates that are not needed.
import pygeos
signatures["geometry"] = pygeos.apply(signatures.geometry.values.data, lambda x: x, include_z=False)
signatures
id | type | geometry | |
---|---|---|---|
0 | 0 | Countryside agriculture | POLYGON ((62220.000 798500.000, 62110.000 7985... |
1 | 1 | Countryside agriculture | POLYGON ((63507.682 796515.169, 63471.097 7965... |
2 | 2 | Countryside agriculture | POLYGON ((65953.174 802246.172, 65950.620 8022... |
3 | 3 | Countryside agriculture | POLYGON ((67297.740 803435.800, 67220.289 8034... |
4 | 4 | Countryside agriculture | POLYGON ((75760.000 852670.000, 75700.000 8527... |
... | ... | ... | ... |
96699 | 96699 | outlier | POLYGON ((323321.005 463795.416, 323319.842 46... |
96700 | 96700 | outlier | POLYGON ((325929.840 1008792.061, 325927.377 1... |
96701 | 96701 | outlier | POLYGON ((337804.770 1013422.583, 337800.122 1... |
96702 | 96702 | outlier | POLYGON ((422304.270 1147826.990, 422296.000 1... |
96703 | 96703 | outlier | POLYGON ((525396.260 439215.480, 525360.920 43... |
96704 rows × 3 columns
Cleanup sliver geometries¶
Remove artifacts caused by floating point imprecision in dissolving of ET cells into signature geometries.
from shapely.geometry import Polygon
signatures_ddf = dask_geopandas.from_geopandas(signatures, npartitions=4)
def fix(gdf):
new = []
for poly in gdf.geometry:
new.append(Polygon(shell=poly.exterior, holes=[i for i in poly.interiors if Polygon(i).area > 1]))
return geopandas.GeoSeries(new, index=gdf.index, crs=gdf.crs)
signatures_ddf["geometry"] = signatures_ddf.map_partitions(fix, meta=geopandas.GeoSeries())
signatures.compute().to_file("spatial_signatures_GB.gpkg", driver="GPKG")
Link data¶
We also need CSV summarising geometries, but since we want to use the original characters before contextualisation and standardisation, we need to join them to geometry.
Harmonize Corine data.¶
import pyarrow.parquet as pq
import pandas as pd
columns = set()
for i in range(103):
schema = pq.read_schema(f"../../urbangrammar_samba/spatial_signatures/functional/corine/corine_{i}.pq")
for c in schema.names:
columns.add(c)
for i in range(103):
df = pd.read_parquet(f"../../urbangrammar_samba/spatial_signatures/functional/corine/corine_{i}.pq")
missing = [c for c in columns if c not in df.columns]
df[missing] = 0
df.to_parquet(f"../../urbangrammar_samba/spatial_signatures/functional/corine/corine_{i}.pq")
Join data¶
from dask.distributed import Client, LocalCluster
client = Client(LocalCluster(n_workers=8))
client
Client
Client-b5f9b711-0a6c-11ec-85f9-0242ac110002
Connection method: Cluster object | Cluster type: distributed.LocalCluster |
Dashboard: http://127.0.0.1:8787/status |
Cluster Info
LocalCluster
c364f511
Dashboard: http://127.0.0.1:8787/status | Workers: 8 |
Total threads: 16 | Total memory: 125.55 GiB |
Status: running | Using processes: True |
Scheduler Info
Scheduler
Scheduler-7f95868f-38c0-41cc-bef2-91e05d88ddb8
Comm: tcp://127.0.0.1:37701 | Workers: 8 |
Dashboard: http://127.0.0.1:8787/status | Total threads: 16 |
Started: Just now | Total memory: 125.55 GiB |
Workers
Worker: 0
Comm: tcp://127.0.0.1:36115 | Total threads: 2 |
Dashboard: http://127.0.0.1:37581/status | Memory: 15.69 GiB |
Nanny: tcp://127.0.0.1:36133 | |
Local directory: /home/jovyan/work/spatial_signatures/data_product/dask-worker-space/worker-w6mvf4ot |
Worker: 1
Comm: tcp://127.0.0.1:34095 | Total threads: 2 |
Dashboard: http://127.0.0.1:41073/status | Memory: 15.69 GiB |
Nanny: tcp://127.0.0.1:42657 | |
Local directory: /home/jovyan/work/spatial_signatures/data_product/dask-worker-space/worker-_dgk8ggr |
Worker: 2
Comm: tcp://127.0.0.1:36553 | Total threads: 2 |
Dashboard: http://127.0.0.1:46527/status | Memory: 15.69 GiB |
Nanny: tcp://127.0.0.1:40465 | |
Local directory: /home/jovyan/work/spatial_signatures/data_product/dask-worker-space/worker-w5tfmvxj |
Worker: 3
Comm: tcp://127.0.0.1:37429 | Total threads: 2 |
Dashboard: http://127.0.0.1:46297/status | Memory: 15.69 GiB |
Nanny: tcp://127.0.0.1:43827 | |
Local directory: /home/jovyan/work/spatial_signatures/data_product/dask-worker-space/worker-qq8ve98m |
Worker: 4
Comm: tcp://127.0.0.1:40931 | Total threads: 2 |
Dashboard: http://127.0.0.1:36109/status | Memory: 15.69 GiB |
Nanny: tcp://127.0.0.1:41977 | |
Local directory: /home/jovyan/work/spatial_signatures/data_product/dask-worker-space/worker-o4ibcabn |
Worker: 5
Comm: tcp://127.0.0.1:38313 | Total threads: 2 |
Dashboard: http://127.0.0.1:42449/status | Memory: 15.69 GiB |
Nanny: tcp://127.0.0.1:44105 | |
Local directory: /home/jovyan/work/spatial_signatures/data_product/dask-worker-space/worker-erb1jj_t |
Worker: 6
Comm: tcp://127.0.0.1:39061 | Total threads: 2 |
Dashboard: http://127.0.0.1:43121/status | Memory: 15.69 GiB |
Nanny: tcp://127.0.0.1:38433 | |
Local directory: /home/jovyan/work/spatial_signatures/data_product/dask-worker-space/worker-0uhi0o89 |
Worker: 7
Comm: tcp://127.0.0.1:37331 | Total threads: 2 |
Dashboard: http://127.0.0.1:35769/status | Memory: 15.69 GiB |
Nanny: tcp://127.0.0.1:39703 | |
Local directory: /home/jovyan/work/spatial_signatures/data_product/dask-worker-space/worker-plm8h9tl |
tessellation = dask_geopandas.read_parquet("../../urbangrammar_samba/spatial_signatures/tessellation/tess_*.pq")
/opt/conda/lib/python3.8/site-packages/dask_geopandas/io/parquet.py:83: FutureWarning: Assigning CRS to a GeoDataFrame without a geometry column is now deprecated and will not be supported in the future.
meta = geopandas.GeoDataFrame(meta, crs=crs)
tessellation = dask_geopandas.from_dask_dataframe(tessellation[["hindex", "tessellation"]]).set_geometry("tessellation").explode()
tessellation["point"] = tessellation.representative_point()
tessellation = tessellation.set_geometry("point")
joined = dask_geopandas.sjoin(tessellation, signatures, op="within")
/opt/conda/lib/python3.8/site-packages/dask_geopandas/sjoin.py:50: UserWarning: CRS mismatch between the CRS of left geometries and the CRS of right geometries.
Use `to_crs()` to reproject one of the input geometries to match the CRS of the other.
Left CRS: None
Right CRS: EPSG:27700
meta = geopandas.sjoin(left._meta, right._meta, how=how, op=op)
%%time
joined[["hindex", "id", "type"]].to_parquet("../../urbangrammar_samba/spatial_signatures/signatures/hindex_to_type")
CPU times: user 17.1 s, sys: 3.16 s, total: 20.3 s
Wall time: 1min 33s
Second bit.
joined = dask.dataframe.read_parquet("../../urbangrammar_samba/spatial_signatures/signatures/hindex_to_type")
pop = dask.dataframe.read_parquet(f"../../urbangrammar_samba/spatial_signatures/functional/population/pop_*")
nl = dask.dataframe.read_parquet(f"../../urbangrammar_samba/spatial_signatures/functional/night_lights/nl_*")
workplace = dask.dataframe.read_parquet(f"../../urbangrammar_samba/spatial_signatures/functional/workplace/pop_*")
corine = dask.dataframe.read_parquet(f"../../urbangrammar_samba/spatial_signatures/functional/corine/corine_*.pq")
ndvi = dask.dataframe.read_parquet(f"../../urbangrammar_samba/functional_data/ndvi/ndvi_tess_*.pq")
pois = dask.dataframe.read_parquet(f"../../urbangrammar_samba/spatial_signatures/functional/accessibility/access_*.pq")
water = dask.dataframe.read_parquet(f'../../urbangrammar_samba/spatial_signatures/functional/water/water_*.pq')
retail_centres = dask.dataframe.read_parquet(f'../../urbangrammar_samba/spatial_signatures/functional/retail_centre/retail_*.pq')
function = dask.dataframe.multi.concat([pop, nl.drop(columns="hindex"), workplace.drop(columns="hindex"), corine.drop(columns="hindex"), ndvi.reset_index(drop=True), pois.drop(columns="hindex"), water.drop(columns="hindex"), retail_centres.drop(columns="hindex")], axis=1)
/opt/conda/lib/python3.8/site-packages/dask/dataframe/multi.py:1212: UserWarning: Concatenating dataframes with unknown divisions.
We're assuming that the indices of each dataframes are
aligned. This assumption is not generally safe.
warnings.warn(
%%time
function.to_parquet("../../urbangrammar_samba/spatial_signatures/functional/combined_raw")
Another bit
function = dask.dataframe.read_parquet("../../urbangrammar_samba/spatial_signatures/functional/combined_raw")
function
hindex | population | night_lights | A, B, D, E. Agriculture, energy and water | C. Manufacturing | F. Construction | G, I. Distribution, hotels and restaurants | H, J. Transport and communication | K, L, M, N. Financial, real estate, professional and administrative activities | O,P,Q. Public administration, education and health | R, S, T, U. Other | Code_18_124 | Code_18_211 | Code_18_121 | Code_18_421 | Code_18_522 | Code_18_142 | Code_18_141 | Code_18_112 | Code_18_231 | Code_18_311 | Code_18_131 | Code_18_123 | Code_18_122 | Code_18_512 | Code_18_243 | Code_18_313 | Code_18_412 | Code_18_321 | Code_18_322 | Code_18_324 | Code_18_111 | Code_18_423 | Code_18_523 | Code_18_133 | Code_18_334 | Code_18_132 | Code_18_242 | Code_18_411 | Code_18_511 | Code_18_312 | Code_18_332 | Code_18_521 | Code_18_331 | Code_18_244 | Code_18_333 | Code_18_222 | mean | supermarkets_nearest | supermarkets_counts | listed_nearest | listed_counts | fhrs_nearest | fhrs_counts | culture_nearest | culture_counts | nearest_water | nearest_retail_centre | |
---|---|---|---|---|---|---|---|---|---|---|---|---|---|---|---|---|---|---|---|---|---|---|---|---|---|---|---|---|---|---|---|---|---|---|---|---|---|---|---|---|---|---|---|---|---|---|---|---|---|---|---|---|---|---|---|---|---|---|
npartitions=103 | ||||||||||||||||||||||||||||||||||||||||||||||||||||||||||
object | float64 | float64 | float64 | float64 | float64 | float64 | float64 | float64 | float64 | float64 | float64 | float64 | float64 | float64 | float64 | float64 | float64 | float64 | float64 | float64 | float64 | float64 | float64 | float64 | float64 | float64 | float64 | float64 | float64 | float64 | float64 | float64 | float64 | int64 | int64 | int64 | int64 | int64 | int64 | int64 | int64 | int64 | int64 | int64 | int64 | int64 | float64 | float64 | int64 | float64 | int64 | float64 | int64 | float64 | int64 | float64 | float64 | |
... | ... | ... | ... | ... | ... | ... | ... | ... | ... | ... | ... | ... | ... | ... | ... | ... | ... | ... | ... | ... | ... | ... | ... | ... | ... | ... | ... | ... | ... | ... | ... | ... | ... | ... | ... | ... | ... | ... | ... | ... | ... | ... | ... | ... | ... | ... | ... | ... | ... | ... | ... | ... | ... | ... | ... | ... | ... | |
... | ... | ... | ... | ... | ... | ... | ... | ... | ... | ... | ... | ... | ... | ... | ... | ... | ... | ... | ... | ... | ... | ... | ... | ... | ... | ... | ... | ... | ... | ... | ... | ... | ... | ... | ... | ... | ... | ... | ... | ... | ... | ... | ... | ... | ... | ... | ... | ... | ... | ... | ... | ... | ... | ... | ... | ... | ... | ... |
... | ... | ... | ... | ... | ... | ... | ... | ... | ... | ... | ... | ... | ... | ... | ... | ... | ... | ... | ... | ... | ... | ... | ... | ... | ... | ... | ... | ... | ... | ... | ... | ... | ... | ... | ... | ... | ... | ... | ... | ... | ... | ... | ... | ... | ... | ... | ... | ... | ... | ... | ... | ... | ... | ... | ... | ... | ... | |
... | ... | ... | ... | ... | ... | ... | ... | ... | ... | ... | ... | ... | ... | ... | ... | ... | ... | ... | ... | ... | ... | ... | ... | ... | ... | ... | ... | ... | ... | ... | ... | ... | ... | ... | ... | ... | ... | ... | ... | ... | ... | ... | ... | ... | ... | ... | ... | ... | ... | ... | ... | ... | ... | ... | ... | ... | ... |
form = dask.dataframe.read_parquet("../../urbangrammar_samba/spatial_signatures/morphometrics/cells/cells_*.pq")
form
hindex | tessellation | buildings | sdbAre | sdbPer | sdbCoA | ssbCCo | ssbCor | ssbSqu | ssbERI | ssbElo | ssbCCM | ssbCCD | stbOri | sdcLAL | sdcAre | sscCCo | sscERI | stcOri | sicCAR | stbCeA | mtbAli | mtbNDi | mtcWNe | mdcAre | ltcWRE | ltbIBD | nodeID | sdsSPW | sdsSWD | sdsSPO | sdsLen | sssLin | ldsMSL | mtdDeg | lcdMes | linP3W | linP4W | linPDE | lcnClo | ldsCDL | xcnSCl | mtdMDi | lddNDe | linWID | edgeID_keys | edgeID_values | edgeID_primary | stbSAl | sddAre | sdsAre | sisBpM | misCel | mdsAre | lisCel | ldsAre | ltcRea | ltcAre | ldeAre | ldePer | lseCCo | lseERI | lseCWA | lteOri | lteWNB | lieWCe | |
---|---|---|---|---|---|---|---|---|---|---|---|---|---|---|---|---|---|---|---|---|---|---|---|---|---|---|---|---|---|---|---|---|---|---|---|---|---|---|---|---|---|---|---|---|---|---|---|---|---|---|---|---|---|---|---|---|---|---|---|---|---|---|---|---|---|---|
npartitions=103 | ||||||||||||||||||||||||||||||||||||||||||||||||||||||||||||||||||
object | object | object | float64 | float64 | float64 | float64 | float64 | float64 | float64 | float64 | float64 | float64 | float64 | float64 | float64 | float64 | float64 | float64 | float64 | float64 | float64 | float64 | float64 | float64 | float64 | float64 | int64 | float64 | float64 | float64 | float64 | float64 | float64 | int64 | float64 | float64 | float64 | float64 | float64 | float64 | float64 | float64 | float64 | float64 | object | object | float64 | float64 | float64 | float64 | float64 | float64 | float64 | float64 | float64 | int64 | float64 | float64 | float64 | float64 | float64 | float64 | float64 | float64 | float64 | |
... | ... | ... | ... | ... | ... | ... | ... | ... | ... | ... | ... | ... | ... | ... | ... | ... | ... | ... | ... | ... | ... | ... | ... | ... | ... | ... | ... | ... | ... | ... | ... | ... | ... | ... | ... | ... | ... | ... | ... | ... | ... | ... | ... | ... | ... | ... | ... | ... | ... | ... | ... | ... | ... | ... | ... | ... | ... | ... | ... | ... | ... | ... | ... | ... | ... | |
... | ... | ... | ... | ... | ... | ... | ... | ... | ... | ... | ... | ... | ... | ... | ... | ... | ... | ... | ... | ... | ... | ... | ... | ... | ... | ... | ... | ... | ... | ... | ... | ... | ... | ... | ... | ... | ... | ... | ... | ... | ... | ... | ... | ... | ... | ... | ... | ... | ... | ... | ... | ... | ... | ... | ... | ... | ... | ... | ... | ... | ... | ... | ... | ... | ... | ... |
... | ... | ... | ... | ... | ... | ... | ... | ... | ... | ... | ... | ... | ... | ... | ... | ... | ... | ... | ... | ... | ... | ... | ... | ... | ... | ... | ... | ... | ... | ... | ... | ... | ... | ... | ... | ... | ... | ... | ... | ... | ... | ... | ... | ... | ... | ... | ... | ... | ... | ... | ... | ... | ... | ... | ... | ... | ... | ... | ... | ... | ... | ... | ... | ... | ... | |
... | ... | ... | ... | ... | ... | ... | ... | ... | ... | ... | ... | ... | ... | ... | ... | ... | ... | ... | ... | ... | ... | ... | ... | ... | ... | ... | ... | ... | ... | ... | ... | ... | ... | ... | ... | ... | ... | ... | ... | ... | ... | ... | ... | ... | ... | ... | ... | ... | ... | ... | ... | ... | ... | ... | ... | ... | ... | ... | ... | ... | ... | ... | ... | ... | ... |
form = form.drop(columns=["tessellation", "buildings"])
data = form.merge(function, on="hindex", how="left")
%%time
data.to_parquet("../../urbangrammar_samba/spatial_signatures/data_combined_raw")
distributed.nanny - WARNING - Worker process still alive after 3 seconds, killing
CPU times: user 7min 38s, sys: 44.6 s, total: 8min 23s
Wall time: 37min 11s
data = dask.dataframe.read_parquet("../../urbangrammar_samba/spatial_signatures/data_combined_raw")
merged = joined.merge(data, on="hindex", how="left")
With all data merged together, we can create summaries using groupby.
per_geometry = merged.drop(columns=["hindex", "type"]).groupby("id").mean()
per_type = merged.drop(columns=["hindex", "id"]).groupby("type").mean()
%%time
per_type, per_geometry = dask.compute(per_type, per_geometry)
CPU times: user 1min 1s, sys: 5.53 s, total: 1min 7s
Wall time: 3min 42s
per_type
sdbAre | sdbPer | sdbCoA | ssbCCo | ssbCor | ssbSqu | ssbERI | ssbElo | ssbCCM | ssbCCD | ... | supermarkets_nearest | supermarkets_counts | listed_nearest | listed_counts | fhrs_nearest | fhrs_counts | culture_nearest | culture_counts | nearest_water | nearest_retail_centre | |
---|---|---|---|---|---|---|---|---|---|---|---|---|---|---|---|---|---|---|---|---|---|
type | |||||||||||||||||||||
Accessible suburbia | 176.949135 | 53.902697 | 0.476838 | 0.534856 | 4.253845 | 0.775272 | 0.987707 | 0.642271 | 9.604873 | 0.364329 | ... | 828.822261 | 1.890519 | 744.223994 | 11.273578 | 218.460768 | 334.434991 | 5384.638746 | 0.059113 | 542.613661 | 849.447871 |
Connected residential neighbourhoods | 272.522220 | 69.119967 | 1.069872 | 0.480941 | 4.445515 | 1.466426 | 0.979415 | 0.555468 | 12.406840 | 0.707494 | ... | 679.958940 | 2.855980 | 596.613654 | 24.279146 | 152.479922 | 692.664050 | 3946.052517 | 0.129197 | 555.964942 | 536.469155 |
Countryside agriculture | 204.100400 | 56.048984 | 0.505831 | 0.506275 | 4.371156 | 0.809087 | 0.977789 | 0.600065 | 9.791869 | 0.556915 | ... | 4751.226429 | 0.089042 | 557.936276 | 11.223066 | 725.690643 | 44.465529 | 13156.203765 | 0.003804 | 304.488181 | 4943.972107 |
Dense residential neighbourhoods | 375.598290 | 80.558473 | 2.127340 | 0.472554 | 4.694714 | 1.863620 | 0.969912 | 0.557054 | 13.961420 | 1.070784 | ... | 661.765594 | 3.127412 | 506.614715 | 37.474705 | 144.021617 | 860.927102 | 3497.511181 | 0.259194 | 483.124139 | 421.093530 |
Dense urban neighbourhoods | 588.359559 | 107.362849 | 5.034084 | 0.435714 | 5.208242 | 3.278419 | 0.950431 | 0.519821 | 17.999101 | 1.877968 | ... | 587.276810 | 4.436632 | 350.890339 | 62.783422 | 106.084358 | 1568.443597 | 2287.432199 | 0.476118 | 528.850109 | 224.326713 |
Disconnected suburbia | 212.713958 | 61.631662 | 0.519372 | 0.491588 | 4.347900 | 1.019293 | 0.981779 | 0.573786 | 11.114826 | 0.539407 | ... | 761.858373 | 2.067315 | 729.608517 | 24.181257 | 217.945624 | 342.081722 | 5831.523433 | 0.077958 | 523.050240 | 725.573382 |
Distilled urbanity | 3713.379427 | 376.300388 | 159.087608 | 0.425186 | 12.484667 | 18.589482 | 0.776950 | 0.594314 | 35.927794 | 9.034682 | ... | 229.903096 | 22.510563 | 31.732228 | 685.156338 | 16.219050 | 6297.607746 | 702.750880 | 10.392254 | 565.248203 | 29.803442 |
Gridded residential quarters | 283.892607 | 69.667245 | 0.749026 | 0.491754 | 4.506685 | 1.663151 | 0.976842 | 0.584548 | 12.411805 | 0.799002 | ... | 577.683974 | 3.409903 | 516.197376 | 31.771826 | 129.244204 | 1081.376510 | 4094.915253 | 0.240503 | 522.087434 | 445.518972 |
Hyper distilled urbanity | 3358.099586 | 330.818576 | 90.819544 | 0.446292 | 9.273438 | 22.513954 | 0.802892 | 0.617669 | 37.220199 | 7.696910 | ... | 324.416312 | 18.791045 | 69.749236 | 1142.567164 | 14.101269 | 9213.145522 | 351.325110 | 34.197761 | 759.604208 | 32.544669 |
Local urbanity | 823.354285 | 135.543919 | 12.666729 | 0.409310 | 6.014239 | 5.071848 | 0.919085 | 0.506415 | 20.711500 | 2.976781 | ... | 483.017862 | 6.848327 | 216.858599 | 140.031073 | 82.468961 | 2167.909991 | 1273.226897 | 1.129622 | 507.706457 | 161.854989 |
Metropolitan urbanity | 2413.938716 | 283.935149 | 118.946949 | 0.395862 | 9.717504 | 12.406894 | 0.820142 | 0.527758 | 29.677259 | 6.780030 | ... | 299.930496 | 17.271958 | 51.874364 | 456.529630 | 40.063982 | 4490.949206 | 644.531259 | 4.446825 | 467.713631 | 66.318054 |
Open sprawl | 226.715098 | 59.639780 | 0.900478 | 0.518531 | 4.371939 | 0.985729 | 0.982452 | 0.622301 | 10.488328 | 0.547552 | ... | 948.025914 | 1.469023 | 760.257254 | 18.171441 | 267.238602 | 253.875226 | 6309.753341 | 0.061560 | 378.356187 | 1002.663064 |
Regional urbanity | 1480.260902 | 195.978660 | 43.190998 | 0.393035 | 7.776863 | 8.844805 | 0.869514 | 0.512959 | 25.254583 | 4.980199 | ... | 331.067781 | 12.531123 | 115.004786 | 324.500752 | 56.865240 | 3163.829678 | 850.254487 | 2.233070 | 461.415859 | 90.874497 |
Urban buffer | 209.421858 | 55.941702 | 0.743395 | 0.523632 | 4.340540 | 0.859973 | 0.982904 | 0.630125 | 9.808575 | 0.492953 | ... | 1752.873479 | 0.654018 | 673.931399 | 16.138833 | 379.169823 | 132.661846 | 8939.647136 | 0.024052 | 345.790747 | 2102.455609 |
Warehouse land | 393.216097 | 75.680270 | 3.263039 | 0.469466 | 4.564890 | 1.352536 | 0.976102 | 0.542256 | 13.200176 | 0.876237 | ... | 1043.835409 | 1.427596 | 934.001152 | 10.570084 | 256.220805 | 271.092537 | 5121.469150 | 0.058667 | 417.431851 | 898.174463 |
Wild countryside | 209.855382 | 57.122052 | 0.223542 | 0.501939 | 4.381272 | 0.706130 | 0.976522 | 0.589908 | 9.947378 | 0.601808 | ... | 9854.123937 | 0.028975 | 1324.025044 | 4.212720 | 1699.169649 | 33.073293 | 20695.290665 | 0.001680 | 236.730324 | 11041.324478 |
outlier | 500.238760 | 64.310418 | 2.870285 | 0.775186 | 11.563107 | 44.142680 | 1.057249 | 0.822132 | 10.632181 | 0.282117 | ... | 3337.054736 | 0.017857 | 1795.228633 | 1.285714 | 2303.176980 | 7.848214 | 7388.413122 | 0.000000 | 10.618568 | 2876.467504 |
17 rows × 118 columns
per_geometry
sdbAre | sdbPer | sdbCoA | ssbCCo | ssbCor | ssbSqu | ssbERI | ssbElo | ssbCCM | ssbCCD | ... | supermarkets_nearest | supermarkets_counts | listed_nearest | listed_counts | fhrs_nearest | fhrs_counts | culture_nearest | culture_counts | nearest_water | nearest_retail_centre | |
---|---|---|---|---|---|---|---|---|---|---|---|---|---|---|---|---|---|---|---|---|---|
id | |||||||||||||||||||||
0 | 129.933434 | 45.146514 | 0.202279 | 0.540968 | 4.172237 | 0.524401 | 0.993543 | 0.622563 | 8.027774 | 0.234288 | ... | 1444.935973 | 0.454774 | 890.196235 | 3.572864 | 341.152792 | 77.364322 | NaN | 0.0 | 136.483329 | 93403.761607 |
4 | 258.461742 | 65.650654 | 5.278706 | 0.500917 | 4.376384 | 0.558092 | 0.975591 | 0.571528 | 11.094158 | 0.675784 | ... | 9034.336389 | 0.000000 | 736.182262 | 1.718412 | 642.509775 | 126.115523 | NaN | 0.0 | 139.419780 | 58846.096367 |
9 | 122.655396 | 46.888304 | 0.000000 | 0.509570 | 4.336957 | 0.606492 | 0.981817 | 0.578105 | 8.168939 | 0.342824 | ... | NaN | 0.000000 | 112.232624 | 4.935484 | 309.115193 | 61.000000 | NaN | 0.0 | 80.087762 | 4762.653237 |
11 | 190.308946 | 59.022824 | 0.764543 | 0.494519 | 4.541985 | 0.997673 | 0.967397 | 0.598092 | 10.134862 | 0.665047 | ... | NaN | 0.000000 | 281.289369 | 8.043478 | 198.296841 | 4.326087 | NaN | 0.0 | 74.377682 | 4378.879114 |
14 | 146.477816 | 50.308964 | 0.229756 | 0.509439 | 4.382090 | 0.769455 | 0.974591 | 0.607979 | 8.836870 | 0.504590 | ... | 2296.657968 | 0.023599 | 519.019717 | 7.519174 | 281.737308 | 24.020649 | NaN | 0.0 | 199.544381 | 1698.769041 |
... | ... | ... | ... | ... | ... | ... | ... | ... | ... | ... | ... | ... | ... | ... | ... | ... | ... | ... | ... | ... | ... |
94815 | NaN | NaN | NaN | NaN | NaN | NaN | NaN | NaN | NaN | NaN | ... | 967.354980 | 1.000000 | 733.508972 | 18.000000 | 294.217987 | 62.000000 | 4342.378906 | 0.0 | 0.000000 | 731.905179 |
94853 | 542.697500 | 98.334230 | 0.000000 | 0.518628 | 4.000000 | 0.078285 | 0.999855 | 0.516309 | 18.248347 | 0.009636 | ... | 547.687012 | 3.000000 | 97.226997 | 77.000000 | 112.362999 | 1346.000000 | 1062.750000 | 1.0 | 160.527776 | 213.305247 |
94892 | NaN | NaN | NaN | NaN | NaN | NaN | NaN | NaN | NaN | NaN | ... | 457.170990 | 5.000000 | 0.000000 | 515.000000 | 38.470001 | 2364.000000 | 401.411011 | 2.0 | 410.039048 | 0.000000 |
96021 | 180.378400 | 57.278130 | 0.000000 | 0.499708 | 4.000000 | 0.025284 | 1.000037 | 0.484826 | 10.717266 | 0.001857 | ... | 327.584015 | 4.000000 | 251.115005 | 3.000000 | 153.737000 | 301.000000 | 2968.321045 | 0.0 | 710.912280 | 317.166726 |
96702 | 54.747100 | 29.614573 | 0.000000 | 0.634613 | 4.000000 | 0.048043 | 1.000000 | 0.932525 | 5.238355 | 0.002229 | ... | 41921.593750 | 0.000000 | 0.000000 | 4.000000 | 1227.026001 | 0.000000 | NaN | 0.0 | 0.000000 | 25185.139279 |
96704 rows × 118 columns
Rename columns¶
coded = {
'population': 'func_population',
'night_lights': 'func_night_lights',
'A, B, D, E. Agriculture, energy and water': 'func_workplace_abde',
'C. Manufacturing': 'func_workplace_c',
'F. Construction': 'func_workplace_f',
'G, I. Distribution, hotels and restaurants': 'func_workplace_gi',
'H, J. Transport and communication': 'func_workplace_hj',
'K, L, M, N. Financial, real estate, professional and administrative activities': 'func_workplace_klmn',
'O,P,Q. Public administration, education and health': 'func_workplace_opq',
'R, S, T, U. Other': 'func_workplace_rstu',
'Code_18_124': 'func_corine_124',
'Code_18_211': 'func_corine_211',
'Code_18_121': 'func_corine_121',
'Code_18_421': 'func_corine_421',
'Code_18_522': 'func_corine_522',
'Code_18_142': 'func_corine_142',
'Code_18_141': 'func_corine_141',
'Code_18_112': 'func_corine_112',
'Code_18_231': 'func_corine_231',
'Code_18_311': 'func_corine_311',
'Code_18_131': 'func_corine_131',
'Code_18_123': 'func_corine_123',
'Code_18_122': 'func_corine_122',
'Code_18_512': 'func_corine_512',
'Code_18_243': 'func_corine_243',
'Code_18_313': 'func_corine_313',
'Code_18_412': 'func_corine_412',
'Code_18_321': 'func_corine_321',
'Code_18_322': 'func_corine_322',
'Code_18_324': 'func_corine_324',
'Code_18_111': 'func_corine_111',
'Code_18_423': 'func_corine_423',
'Code_18_523': 'func_corine_523',
'Code_18_312': 'func_corine_312',
'Code_18_133': 'func_corine_133',
'Code_18_333': 'func_corine_333',
'Code_18_332': 'func_corine_332',
'Code_18_411': 'func_corine_411',
'Code_18_132': 'func_corine_132',
'Code_18_222': 'func_corine_222',
'Code_18_242': 'func_corine_242',
'Code_18_331': 'func_corine_331',
'Code_18_511': 'func_corine_511',
'Code_18_334': 'func_corine_334',
'Code_18_244': 'func_corine_244',
'Code_18_521': 'func_corine_521',
'mean': 'ndvi',
'supermarkets_nearest': 'func_supermarkets_nearest',
'supermarkets_counts': 'func_supermarkets_counts',
'listed_nearest': 'func_listed_nearest',
'listed_counts': 'func_listed_counts',
'fhrs_nearest': 'func_fhrs_nearest',
'fhrs_counts': 'func_fhrs_counts',
'culture_nearest': 'func_culture_nearest',
'culture_counts': 'func_culture_counts',
'nearest_water': 'func_water_nearest',
'nearest_retail_centre': 'func_retail_centrenearest',
'sdbAre': 'form_sdbAre',
'sdbPer': 'form_sdbPer',
'sdbCoA': 'form_sdbCoA',
'ssbCCo': 'form_ssbCCo',
'ssbCor': 'form_ssbCor',
'ssbSqu': 'form_ssbSqu',
'ssbERI': 'form_ssbERI',
'ssbElo': 'form_ssbElo',
'ssbCCM': 'form_ssbCCM',
'ssbCCD': 'form_ssbCCD',
'stbOri': 'form_stbOri',
'sdcLAL': 'form_sdcLAL',
'sdcAre': 'form_sdcAre',
'sscCCo': 'form_sscCCo',
'sscERI': 'form_sscERI',
'stcOri': 'form_stcOri',
'sicCAR': 'form_sicCAR',
'stbCeA': 'form_stbCeA',
'mtbAli': 'form_mtbAli',
'mtbNDi': 'form_mtbNDi',
'mtcWNe': 'form_mtcWNe',
'mdcAre': 'form_mdcAre',
'ltcWRE': 'form_ltcWRE',
'ltbIBD': 'form_ltbIBD',
'sdsSPW': 'form_sdsSPW',
'sdsSWD': 'form_sdsSWD',
'sdsSPO': 'form_sdsSPO',
'sdsLen': 'form_sdsLen',
'sssLin': 'form_sssLin',
'ldsMSL': 'form_ldsMSL',
'mtdDeg': 'form_mtdDeg',
'lcdMes': 'form_lcdMes',
'linP3W': 'form_linP3W',
'linP4W': 'form_linP4W',
'linPDE': 'form_linPDE',
'lcnClo': 'form_lcnClo',
'ldsCDL': 'form_ldsCDL',
'xcnSCl': 'form_xcnSCl',
'mtdMDi': 'form_mtdMDi',
'lddNDe': 'form_lddNDe',
'linWID': 'form_linWID',
'stbSAl': 'form_stbSAl',
'sddAre': 'form_sddAre',
'sdsAre': 'form_sdsAre',
'sisBpM': 'form_sisBpM',
'misCel': 'form_misCel',
'mdsAre': 'form_mdsAre',
'lisCel': 'form_lisCel',
'ldsAre': 'form_ldsAre',
'ltcRea': 'form_ltcRea',
'ltcAre': 'form_ltcAre',
'ldeAre': 'form_ldeAre',
'ldePer': 'form_ldePer',
'lseCCo': 'form_lseCCo',
'lseERI': 'form_lseERI',
'lseCWA': 'form_lseCWA',
'lteOri': 'form_lteOri',
'lteWNB': 'form_lteWNB',
'lieWCe': 'form_lieWCe',
}
key = {
'func_population': 'Population',
'func_night_lights': 'Night lights',
'func_workplace_abde': 'Workplace population [Agriculture, energy and water]',
'func_workplace_c': 'Workplace population [Manufacturing]',
'func_workplace_f': 'Workplace population [Construction]',
'func_workplace_gi': 'Workplace population [Distribution, hotels and restaurants]',
'func_workplace_hj': 'Workplace population [Transport and communication]',
'func_workplace_klmn': 'Workplace population [Financial, real estate, professional and administrative activities]',
'func_workplace_opq': 'Workplace population [Public administration, education and health]',
'func_workplace_rstu': 'Workplace population [Other]',
'func_corine_124': 'Land cover [Airports]',
'func_corine_211': 'Land cover [Non-irrigated arable land]',
'func_corine_121': 'Land cover [Industrial or commercial units]',
'func_corine_421': 'Land cover [Salt marshes]',
'func_corine_522': 'Land cover [Estuaries]',
'func_corine_142': 'Land cover [Sport and leisure facilities]',
'func_corine_141': 'Land cover [Green urban areas]',
'func_corine_112': 'Land cover [Discontinuous urban fabric]',
'func_corine_231': 'Land cover [Pastures]',
'func_corine_311': 'Land cover [Broad-leaved forest]',
'func_corine_131': 'Land cover [Mineral extraction sites]',
'func_corine_123': 'Land cover [Port areas]',
'func_corine_122': 'Land cover [Road and rail networks and associated land]',
'func_corine_512': 'Land cover [Water bodies]',
'func_corine_243': 'Land cover [Land principally occupied by agriculture, with significant areas of natural vegetation]',
'func_corine_313': 'Land cover [Mixed forest]',
'func_corine_412': 'Land cover [Peat bogs]',
'func_corine_321': 'Land cover [Natural grasslands]',
'func_corine_322': 'Land cover [Moors and heathland]',
'func_corine_324': 'Land cover [Transitional woodland-shrub]',
'func_corine_111': 'Land cover [Continuous urban fabric]',
'func_corine_423': 'Land cover [Intertidal flats]',
'func_corine_523': 'Land cover [Sea and ocean]',
'func_corine_312': 'Land cover [Coniferous forest]',
'func_corine_133': 'Land cover [Construction sites]',
'func_corine_333': 'Land cover [Sparsely vegetated areas]',
'func_corine_332': 'Land cover [Bare rocks]',
'func_corine_411': 'Land cover [Inland marshes]',
'func_corine_132': 'Land cover [Dump sites]',
'func_corine_222': 'Land cover [Fruit trees and berry plantations]',
'func_corine_242': 'Land cover [Complex cultivation patterns]',
'func_corine_331': 'Land cover [Beaches, dunes, sands]',
'func_corine_511': 'Land cover [Water courses]',
'func_corine_334': 'Land cover [Burnt areas]',
'func_corine_244': 'Land cover [Agro-forestry areas]',
'func_corine_521': 'Land cover [Coastal lagoons]',
'ndvi': 'NDVI',
'func_supermarkets_nearest': 'Supermarkets [distance to nearest]',
'func_supermarkets_counts': 'Supermarkets [counts within 1200m]',
'func_listed_nearest': 'Listed buildings [distance to nearest]',
'func_listed_counts': 'Listed buildings [counts within 1200m]',
'func_fhrs_nearest': 'FHRS points [distance to nearest]',
'func_fhrs_counts': 'FHRS points [counts within 1200m]',
'func_culture_nearest': 'Cultural venues [distance to nearest]',
'func_culture_counts': 'Cultural venues [counts within 1200m]',
'func_water_nearest': 'Water bodies [distance to nearest]',
'func_retail_centrenearest': 'Retail centres [distance to nearest]',
'form_sdbAre': 'area of building',
'form_sdbPer': 'perimeter of building',
'form_sdbCoA': 'courtyard area of building',
'form_ssbCCo': 'circular compactness of building',
'form_ssbCor': 'corners of building',
'form_ssbSqu': 'squareness of building',
'form_ssbERI': 'equivalent rectangular index of building',
'form_ssbElo': 'elongation of building',
'form_ssbCCM': 'centroid - corner mean distance of building',
'form_ssbCCD': 'centroid - corner distance deviation of building',
'form_stbOri': 'orientation of building',
'form_sdcLAL': 'longest axis length of ETC',
'form_sdcAre': 'area of ETC',
'form_sscCCo': 'circular compactness of ETC',
'form_sscERI': 'equivalent rectangular index of ETC',
'form_stcOri': 'orientation of ETC',
'form_sicCAR': 'covered area ratio of ETC',
'form_stbCeA': 'cell alignment of building',
'form_mtbAli': 'alignment of neighbouring buildings',
'form_mtbNDi': 'mean distance between neighbouring buildings',
'form_mtcWNe': 'perimeter-weighted neighbours of ETC',
'form_mdcAre': 'area covered by neighbouring cells',
'form_ltcWRE': 'weighted reached enclosures of ETC',
'form_ltbIBD': 'mean inter-building distance',
'form_sdsSPW': 'width of street profile',
'form_sdsSWD': 'width deviation of street profile',
'form_sdsSPO': 'openness of street profile',
'form_sdsLen': 'length of street segment',
'form_sssLin': 'linearity of street segment',
'form_ldsMSL': 'mean segment length within 3 steps',
'form_mtdDeg': 'node degree of junction',
'form_lcdMes': 'local meshedness of street network',
'form_linP3W': 'local proportion of 3-way intersections of street network',
'form_linP4W': 'local proportion of 4-way intersections of street network',
'form_linPDE': 'local proportion of cul-de-sacs of street network',
'form_lcnClo': 'local closeness of street network',
'form_ldsCDL': 'local cul-de-sac length of street network',
'form_xcnSCl': 'square clustering of street network',
'form_mtdMDi': 'mean distance to neighbouring nodes of street network',
'form_lddNDe': 'local node density of street network',
'form_linWID': 'local degree weighted node density of street network',
'form_stbSAl': 'street alignment of building',
'form_sddAre': 'area covered by node-attached ETCs',
'form_sdsAre': 'area covered by edge-attached ETCs',
'form_sisBpM': 'buildings per meter of street segment',
'form_misCel': 'reached ETCs by neighbouring segments',
'form_mdsAre': 'reached area by neighbouring segments',
'form_lisCel': 'reached ETCs by local street network',
'form_ldsAre': 'reached area by local street network',
'form_ltcRea': 'reached ETCs by tessellation contiguity',
'form_ltcAre': 'reached area by tessellation contiguity',
'form_ldeAre': 'area of enclosure',
'form_ldePer': 'perimeter of enclosure',
'form_lseCCo': 'circular compactness of enclosure',
'form_lseERI': 'equivalent rectangular index of enclosure',
'form_lseCWA': 'compactness-weighted axis of enclosure',
'form_lteOri': 'orientation of enclosure',
'form_lteWNB': 'perimeter-weighted neighbours of enclosure',
'form_lieWCe': 'area-weighted ETCs of enclosure',
}
Assing new column names
per_type = per_type.rename(columns=coded)
per_type.drop("outlier").to_csv("per_type.csv")
per_geometry = per_geometry.rename(columns=coded)
per_geometry.to_csv("per_geometry.csv")
pandas.Series(key).to_csv("key.csv")
Pen portraits JSON¶
import json
portraits ={
"Wild countryside": "In “Wild countryside”, human influence is the least intensive. This signature covers large open spaces in the countryside where no urbanisation happens apart from occasional roads, cottages, and pastures. You can find it across the Scottish Highlands, numerous national parks such as Lake District, or in the majority of Wales.",
"Countryside agriculture": "“Countryside agriculture” features much of the English countryside and displays a high degree of agriculture including both fields and pastures. There are a few buildings scattered across the area but, for the most part, it is green space.",
"Urban buffer": "“Urban buffer” can be characterised as a green belt around cities. This signature includes mostly agricultural land in the immediate adjacency of towns and cities, often including edge development. It still feels more like countryside than urban, but these signatures are much smaller compared to other countryside types.",
"Open sprawl": "“Open sprawl” represents the transition between countryside and urbanised land. It is located in the outskirts of cities or around smaller towns and is typically made up of large open space areas intertwined with different kinds of human development, from highways to smaller neighbourhoods.",
"Disconnected suburbia": "“Disconnected suburbia” includes residential developments in the outskirts of cities or even towns and villages with convoluted, disconnected street networks, low built-up and population densities, and lack of jobs and services. This signature type is entirely car-dependent.",
"Accessible suburbia": "“Accessible suburbia” covers residential development on the urban periphery with a relatively legible and connected street network, albeit less so than other more urban signature types. Areas in this signature feature low density, both in terms of population and built-up area, lack of jobs and services. For these reasons, “accessible suburbia” largely acts as dormitories.",
"Warehouse/Park land": "“Warehouse/Park land” covers predominantly industrial areas and other work-related developments made of box-like buildings with large footprints. It contains many jobs of manual nature such as manufacturing or construction, and very little population live here compared to the rest of urban areas. Occasionally this type also covers areas of parks with large scale green open areas.",
"Gridded residential quarters": "“Gridded residential quarters” are areas with street networks forming a well-connected grid-like (high density of 4-way intersections) pattern, resulting in places with smaller blocks and higher granularity. This signature is mostly residential but includes some services and jobs, and it tends to be located away from city centres.",
"Connected residential neighbourhoods": "“Connected residential neighbourhoods” are relatively dense urban areas, both in terms of population and built-up area, that tend to be formed around well-connected street networks. They have access to services and some jobs but may be further away from city centres leading to higher dependency on cars and public transport for their residents.",
"Dense residential neighbourhoods": "A “dense residential neighbourhood” is an abundant signature often covering large parts of cities outside of their centres. It has primarily residential purpose and high population density, varied street network patterns, and some services and jobs but not in high intensity.",
"Dense urban neighbourhoods": "“Dense urban neighbourhoods” are areas of inner-city with high population and built-up density of a predominantly residential nature but with direct access to jobs and services. This signature type tends to be relatively walkable and, in the case of some towns, may even form their centres.",
"Local urbanity": "“Local urbanity” reflects town centres, outer parts of city centres or even district centres. In all cases, this signature is very much urban in essence, combining high population and built-up density, access to amenities and jobs. Yet, it is on the lower end of the hierarchy of signature types denoting urban centres with only a local significance.",
"Regional urbanity": "“Regional urbanity” captures centres of mid-size cities with regional importance such as Liverpool, Plymouth or Newcastle upon Tyne. It is often encircled by “Local urbanity” signatures and can form outer rings of city centres in large cities. It features high population density, as well as a high number of jobs and amenities within walkable distance.",
"Metropolitan urbanity": "Signature type “Metropolitan urbanity” captures the centre of the largest cities in Great Britain such as Glasgow, Birmingham or Manchester. It is characterised by a very high number of jobs in the area, high built-up density and often high population density. This type serves as the core centre of the entire metropolitan areas.",
"Concentrated urbanity": "Concentrated urbanity” is a signature type found in the city centre of London and nowhere else in Great Britain. It reflects the uniqueness of London in the British context with an extremely high number of jobs and amenities located nearby, as well as high built-up and population densities. Buildings in this signature are large and tightly packed, forming complex shapes with courtyards and little green space.",
"Hyper concentrated urbanity": "The epitome of urbanity in the British context. “Hyper concentrated urbanity” is a signature type present only in the centre of London, around the Soho district, and covering Oxford and Regent streets. This signature is the result of centuries of urban primacy, with a multitude of historical layers interwoven, very high built-up and population density, and extreme abundance of amenities, services and jobs.",
}
with open("pen_portraits.json", "w") as outfile:
json.dump(portraits, outfile, indent=4)
Use string IDs¶
Change integer ID for string.
signatures = geopandas.read_file("../../urbangrammar_samba/spatial_signatures/data_product/spatial_signatures_GB.gpkg")
/opt/conda/lib/python3.8/site-packages/geopandas/geodataframe.py:577: RuntimeWarning: Sequential read of iterator was interrupted. Resetting iterator. This can negatively impact the performance.
for feature in features_lst:
signatures
id | type | geometry | |
---|---|---|---|
0 | 0 | Countryside agriculture | POLYGON ((62220.000 798500.000, 62110.000 7985... |
1 | 1 | Countryside agriculture | POLYGON ((63507.682 796515.169, 63471.097 7965... |
2 | 2 | Countryside agriculture | POLYGON ((65953.174 802246.172, 65950.620 8022... |
3 | 3 | Countryside agriculture | POLYGON ((67297.740 803435.800, 67220.289 8034... |
4 | 4 | Countryside agriculture | POLYGON ((75760.000 852670.000, 75700.000 8527... |
... | ... | ... | ... |
96699 | 96699 | outlier | POLYGON ((323321.005 463795.416, 323319.842 46... |
96700 | 96700 | outlier | POLYGON ((325929.840 1008792.061, 325927.377 1... |
96701 | 96701 | outlier | POLYGON ((337804.770 1013422.583, 337800.122 1... |
96702 | 96702 | outlier | POLYGON ((422304.270 1147826.990, 422296.000 1... |
96703 | 96703 | outlier | POLYGON ((525396.260 439215.480, 525360.920 43... |
96704 rows × 3 columns
signatures["type"].unique()
array(['Countryside agriculture', 'Accessible suburbia', 'Open sprawl',
'Wild countryside', 'Warehouse land',
'Gridded residential quarters', 'Urban buffer',
'Disconnected suburbia', 'Dense residential neighbourhoods',
'Connected residential neighbourhoods',
'Dense urban neighbourhoods', 'Local urbanity',
'Distilled urbanity', 'Regional urbanity', 'outlier',
'Metropolitan urbanity', 'Hyper distilled urbanity'], dtype=object)
type_ids = {
'Countryside agriculture': "COA",
'Accessible suburbia': "ACS",
'Open sprawl': "OPS",
'Wild countryside': "WIC",
'Warehouse land': "WAL",
'Gridded residential quarters': "GRQ",
'Urban buffer': "URB",
'Disconnected suburbia': "DIS",
'Dense residential neighbourhoods': "DRN",
'Connected residential neighbourhoods': "CRN",
'Dense urban neighbourhoods': "DUN",
'Local urbanity': "LOU",
'Distilled urbanity': "DIU",
'Regional urbanity': "REU",
'Metropolitan urbanity': "MEU",
'Hyper distilled urbanity': "HDU",
'outlier': "OUT",
}
string_id = signatures["id"].astype(str) + "_" + signatures["type"].map(type_ids)
signatures["id"] = string_id
signatures["code"] = signatures["type"].map(type_ids)
signatures[["id", "code", "type", "geometry"]].to_file("spatial_signatures_GB.gpkg", driver="GPKG")
per_type = pandas.read_csv("../../urbangrammar_samba/spatial_signatures/data_product/per_type.csv")
per_type
type | form_sdbAre | form_sdbPer | form_sdbCoA | form_ssbCCo | form_ssbCor | form_ssbSqu | form_ssbERI | form_ssbElo | form_ssbCCM | ... | func_supermarkets_nearest | func_supermarkets_counts | func_listed_nearest | func_listed_counts | func_fhrs_nearest | func_fhrs_counts | func_culture_nearest | func_culture_counts | func_water_nearest | func_retail_centrenearest | |
---|---|---|---|---|---|---|---|---|---|---|---|---|---|---|---|---|---|---|---|---|---|
0 | Accessible suburbia | 176.949135 | 53.902697 | 0.476838 | 0.534856 | 4.253845 | 0.775272 | 0.987707 | 0.642271 | 9.604873 | ... | 828.822261 | 1.890519 | 744.223994 | 11.273578 | 218.460768 | 334.434991 | 5384.638746 | 0.059113 | 542.613661 | 849.447871 |
1 | Connected residential neighbourhoods | 272.522220 | 69.119967 | 1.069872 | 0.480941 | 4.445515 | 1.466426 | 0.979415 | 0.555468 | 12.406840 | ... | 679.958940 | 2.855980 | 596.613654 | 24.279146 | 152.479922 | 692.664050 | 3946.052517 | 0.129197 | 555.964942 | 536.469155 |
2 | Countryside agriculture | 204.100400 | 56.048984 | 0.505831 | 0.506275 | 4.371156 | 0.809087 | 0.977789 | 0.600065 | 9.791869 | ... | 4751.226429 | 0.089042 | 557.936276 | 11.223066 | 725.690643 | 44.465529 | 13156.203765 | 0.003804 | 304.488181 | 4943.972107 |
3 | Dense residential neighbourhoods | 375.598290 | 80.558473 | 2.127340 | 0.472554 | 4.694714 | 1.863620 | 0.969912 | 0.557054 | 13.961420 | ... | 661.765594 | 3.127412 | 506.614715 | 37.474705 | 144.021617 | 860.927102 | 3497.511181 | 0.259194 | 483.124139 | 421.093530 |
4 | Dense urban neighbourhoods | 588.359559 | 107.362849 | 5.034084 | 0.435714 | 5.208242 | 3.278419 | 0.950431 | 0.519821 | 17.999101 | ... | 587.276810 | 4.436632 | 350.890339 | 62.783422 | 106.084358 | 1568.443597 | 2287.432199 | 0.476118 | 528.850109 | 224.326713 |
5 | Disconnected suburbia | 212.713958 | 61.631662 | 0.519372 | 0.491588 | 4.347900 | 1.019293 | 0.981779 | 0.573786 | 11.114826 | ... | 761.858373 | 2.067315 | 729.608517 | 24.181257 | 217.945624 | 342.081722 | 5831.523433 | 0.077958 | 523.050240 | 725.573382 |
6 | Distilled urbanity | 3713.379427 | 376.300388 | 159.087608 | 0.425186 | 12.484667 | 18.589482 | 0.776950 | 0.594314 | 35.927794 | ... | 229.903096 | 22.510563 | 31.732228 | 685.156338 | 16.219050 | 6297.607746 | 702.750880 | 10.392254 | 565.248203 | 29.803442 |
7 | Gridded residential quarters | 283.892607 | 69.667245 | 0.749026 | 0.491754 | 4.506685 | 1.663151 | 0.976842 | 0.584548 | 12.411805 | ... | 577.683974 | 3.409903 | 516.197376 | 31.771826 | 129.244204 | 1081.376510 | 4094.915253 | 0.240503 | 522.087434 | 445.518972 |
8 | Hyper distilled urbanity | 3358.099586 | 330.818576 | 90.819544 | 0.446292 | 9.273438 | 22.513954 | 0.802892 | 0.617669 | 37.220199 | ... | 324.416312 | 18.791045 | 69.749236 | 1142.567164 | 14.101269 | 9213.145522 | 351.325110 | 34.197761 | 759.604208 | 32.544669 |
9 | Local urbanity | 823.354285 | 135.543919 | 12.666729 | 0.409310 | 6.014239 | 5.071848 | 0.919085 | 0.506415 | 20.711500 | ... | 483.017862 | 6.848327 | 216.858599 | 140.031073 | 82.468961 | 2167.909991 | 1273.226897 | 1.129622 | 507.706457 | 161.854989 |
10 | Metropolitan urbanity | 2413.938716 | 283.935149 | 118.946949 | 0.395862 | 9.717504 | 12.406894 | 0.820142 | 0.527758 | 29.677259 | ... | 299.930496 | 17.271958 | 51.874364 | 456.529630 | 40.063982 | 4490.949206 | 644.531259 | 4.446825 | 467.713631 | 66.318054 |
11 | Open sprawl | 226.715098 | 59.639780 | 0.900478 | 0.518531 | 4.371939 | 0.985729 | 0.982452 | 0.622301 | 10.488328 | ... | 948.025914 | 1.469023 | 760.257254 | 18.171441 | 267.238602 | 253.875226 | 6309.753341 | 0.061560 | 378.356187 | 1002.663064 |
12 | Regional urbanity | 1480.260902 | 195.978660 | 43.190998 | 0.393035 | 7.776863 | 8.844805 | 0.869514 | 0.512959 | 25.254583 | ... | 331.067781 | 12.531123 | 115.004786 | 324.500752 | 56.865240 | 3163.829678 | 850.254487 | 2.233070 | 461.415859 | 90.874497 |
13 | Urban buffer | 209.421858 | 55.941702 | 0.743395 | 0.523632 | 4.340540 | 0.859973 | 0.982904 | 0.630125 | 9.808575 | ... | 1752.873479 | 0.654018 | 673.931399 | 16.138833 | 379.169823 | 132.661846 | 8939.647136 | 0.024052 | 345.790747 | 2102.455609 |
14 | Warehouse land | 393.216097 | 75.680270 | 3.263039 | 0.469466 | 4.564890 | 1.352536 | 0.976102 | 0.542256 | 13.200176 | ... | 1043.835409 | 1.427596 | 934.001152 | 10.570084 | 256.220805 | 271.092537 | 5121.469150 | 0.058667 | 417.431851 | 898.174463 |
15 | Wild countryside | 209.855382 | 57.122052 | 0.223542 | 0.501939 | 4.381272 | 0.706130 | 0.976522 | 0.589908 | 9.947378 | ... | 9854.123937 | 0.028975 | 1324.025044 | 4.212720 | 1699.169649 | 33.073293 | 20695.290665 | 0.001680 | 236.730324 | 11041.324478 |
16 rows × 119 columns
per_type["code"] = per_type["type"].map(type_ids)
columns = ["code"] + list(per_type.columns[:-1])
columns.remove("nodeID")
columns.remove("edgeID_primary")
per_type[columns]
code | type | form_sdbAre | form_sdbPer | form_sdbCoA | form_ssbCCo | form_ssbCor | form_ssbSqu | form_ssbERI | form_ssbElo | ... | func_supermarkets_nearest | func_supermarkets_counts | func_listed_nearest | func_listed_counts | func_fhrs_nearest | func_fhrs_counts | func_culture_nearest | func_culture_counts | func_water_nearest | func_retail_centrenearest | |
---|---|---|---|---|---|---|---|---|---|---|---|---|---|---|---|---|---|---|---|---|---|
0 | ACS | Accessible suburbia | 176.949135 | 53.902697 | 0.476838 | 0.534856 | 4.253845 | 0.775272 | 0.987707 | 0.642271 | ... | 828.822261 | 1.890519 | 744.223994 | 11.273578 | 218.460768 | 334.434991 | 5384.638746 | 0.059113 | 542.613661 | 849.447871 |
1 | CRN | Connected residential neighbourhoods | 272.522220 | 69.119967 | 1.069872 | 0.480941 | 4.445515 | 1.466426 | 0.979415 | 0.555468 | ... | 679.958940 | 2.855980 | 596.613654 | 24.279146 | 152.479922 | 692.664050 | 3946.052517 | 0.129197 | 555.964942 | 536.469155 |
2 | COA | Countryside agriculture | 204.100400 | 56.048984 | 0.505831 | 0.506275 | 4.371156 | 0.809087 | 0.977789 | 0.600065 | ... | 4751.226429 | 0.089042 | 557.936276 | 11.223066 | 725.690643 | 44.465529 | 13156.203765 | 0.003804 | 304.488181 | 4943.972107 |
3 | DRN | Dense residential neighbourhoods | 375.598290 | 80.558473 | 2.127340 | 0.472554 | 4.694714 | 1.863620 | 0.969912 | 0.557054 | ... | 661.765594 | 3.127412 | 506.614715 | 37.474705 | 144.021617 | 860.927102 | 3497.511181 | 0.259194 | 483.124139 | 421.093530 |
4 | DUN | Dense urban neighbourhoods | 588.359559 | 107.362849 | 5.034084 | 0.435714 | 5.208242 | 3.278419 | 0.950431 | 0.519821 | ... | 587.276810 | 4.436632 | 350.890339 | 62.783422 | 106.084358 | 1568.443597 | 2287.432199 | 0.476118 | 528.850109 | 224.326713 |
5 | DIS | Disconnected suburbia | 212.713958 | 61.631662 | 0.519372 | 0.491588 | 4.347900 | 1.019293 | 0.981779 | 0.573786 | ... | 761.858373 | 2.067315 | 729.608517 | 24.181257 | 217.945624 | 342.081722 | 5831.523433 | 0.077958 | 523.050240 | 725.573382 |
6 | DIU | Distilled urbanity | 3713.379427 | 376.300388 | 159.087608 | 0.425186 | 12.484667 | 18.589482 | 0.776950 | 0.594314 | ... | 229.903096 | 22.510563 | 31.732228 | 685.156338 | 16.219050 | 6297.607746 | 702.750880 | 10.392254 | 565.248203 | 29.803442 |
7 | GRQ | Gridded residential quarters | 283.892607 | 69.667245 | 0.749026 | 0.491754 | 4.506685 | 1.663151 | 0.976842 | 0.584548 | ... | 577.683974 | 3.409903 | 516.197376 | 31.771826 | 129.244204 | 1081.376510 | 4094.915253 | 0.240503 | 522.087434 | 445.518972 |
8 | HDU | Hyper distilled urbanity | 3358.099586 | 330.818576 | 90.819544 | 0.446292 | 9.273438 | 22.513954 | 0.802892 | 0.617669 | ... | 324.416312 | 18.791045 | 69.749236 | 1142.567164 | 14.101269 | 9213.145522 | 351.325110 | 34.197761 | 759.604208 | 32.544669 |
9 | LOU | Local urbanity | 823.354285 | 135.543919 | 12.666729 | 0.409310 | 6.014239 | 5.071848 | 0.919085 | 0.506415 | ... | 483.017862 | 6.848327 | 216.858599 | 140.031073 | 82.468961 | 2167.909991 | 1273.226897 | 1.129622 | 507.706457 | 161.854989 |
10 | MEU | Metropolitan urbanity | 2413.938716 | 283.935149 | 118.946949 | 0.395862 | 9.717504 | 12.406894 | 0.820142 | 0.527758 | ... | 299.930496 | 17.271958 | 51.874364 | 456.529630 | 40.063982 | 4490.949206 | 644.531259 | 4.446825 | 467.713631 | 66.318054 |
11 | OPS | Open sprawl | 226.715098 | 59.639780 | 0.900478 | 0.518531 | 4.371939 | 0.985729 | 0.982452 | 0.622301 | ... | 948.025914 | 1.469023 | 760.257254 | 18.171441 | 267.238602 | 253.875226 | 6309.753341 | 0.061560 | 378.356187 | 1002.663064 |
12 | REU | Regional urbanity | 1480.260902 | 195.978660 | 43.190998 | 0.393035 | 7.776863 | 8.844805 | 0.869514 | 0.512959 | ... | 331.067781 | 12.531123 | 115.004786 | 324.500752 | 56.865240 | 3163.829678 | 850.254487 | 2.233070 | 461.415859 | 90.874497 |
13 | URB | Urban buffer | 209.421858 | 55.941702 | 0.743395 | 0.523632 | 4.340540 | 0.859973 | 0.982904 | 0.630125 | ... | 1752.873479 | 0.654018 | 673.931399 | 16.138833 | 379.169823 | 132.661846 | 8939.647136 | 0.024052 | 345.790747 | 2102.455609 |
14 | WAL | Warehouse land | 393.216097 | 75.680270 | 3.263039 | 0.469466 | 4.564890 | 1.352536 | 0.976102 | 0.542256 | ... | 1043.835409 | 1.427596 | 934.001152 | 10.570084 | 256.220805 | 271.092537 | 5121.469150 | 0.058667 | 417.431851 | 898.174463 |
15 | WIC | Wild countryside | 209.855382 | 57.122052 | 0.223542 | 0.501939 | 4.381272 | 0.706130 | 0.976522 | 0.589908 | ... | 9854.123937 | 0.028975 | 1324.025044 | 4.212720 | 1699.169649 | 33.073293 | 20695.290665 | 0.001680 | 236.730324 | 11041.324478 |
16 rows × 118 columns
per_type[columns].to_csv("../../urbangrammar_samba/spatial_signatures/data_product/per_type.csv", index=False)
per_geometry = pandas.read_csv("../../urbangrammar_samba/spatial_signatures/data_product/per_geometry.csv")
per_geometry
id | form_sdbAre | form_sdbPer | form_sdbCoA | form_ssbCCo | form_ssbCor | form_ssbSqu | form_ssbERI | form_ssbElo | form_ssbCCM | ... | func_supermarkets_nearest | func_supermarkets_counts | func_listed_nearest | func_listed_counts | func_fhrs_nearest | func_fhrs_counts | func_culture_nearest | func_culture_counts | func_water_nearest | func_retail_centrenearest | |
---|---|---|---|---|---|---|---|---|---|---|---|---|---|---|---|---|---|---|---|---|---|
0 | 0 | 129.933434 | 45.146514 | 0.202279 | 0.540968 | 4.172237 | 0.524401 | 0.993543 | 0.622563 | 8.027774 | ... | 1444.935973 | 0.454774 | 890.196235 | 3.572864 | 341.152792 | 77.364322 | NaN | 0.0 | 136.483329 | 93403.761607 |
1 | 4 | 258.461742 | 65.650654 | 5.278706 | 0.500917 | 4.376384 | 0.558092 | 0.975591 | 0.571528 | 11.094158 | ... | 9034.336389 | 0.000000 | 736.182262 | 1.718412 | 642.509775 | 126.115523 | NaN | 0.0 | 139.419780 | 58846.096367 |
2 | 9 | 122.655396 | 46.888304 | 0.000000 | 0.509570 | 4.336957 | 0.606492 | 0.981817 | 0.578105 | 8.168939 | ... | NaN | 0.000000 | 112.232624 | 4.935484 | 309.115193 | 61.000000 | NaN | 0.0 | 80.087762 | 4762.653237 |
3 | 11 | 190.308946 | 59.022824 | 0.764543 | 0.494519 | 4.541985 | 0.997673 | 0.967397 | 0.598092 | 10.134862 | ... | NaN | 0.000000 | 281.289369 | 8.043478 | 198.296841 | 4.326087 | NaN | 0.0 | 74.377682 | 4378.879114 |
4 | 14 | 146.477816 | 50.308964 | 0.229756 | 0.509439 | 4.382090 | 0.769455 | 0.974591 | 0.607979 | 8.836870 | ... | 2296.657968 | 0.023599 | 519.019717 | 7.519174 | 281.737308 | 24.020649 | NaN | 0.0 | 199.544381 | 1698.769041 |
... | ... | ... | ... | ... | ... | ... | ... | ... | ... | ... | ... | ... | ... | ... | ... | ... | ... | ... | ... | ... | ... |
96699 | 94815 | NaN | NaN | NaN | NaN | NaN | NaN | NaN | NaN | NaN | ... | 967.354980 | 1.000000 | 733.508972 | 18.000000 | 294.217987 | 62.000000 | 4342.378906 | 0.0 | 0.000000 | 731.905179 |
96700 | 94853 | 542.697500 | 98.334230 | 0.000000 | 0.518628 | 4.000000 | 0.078285 | 0.999855 | 0.516309 | 18.248347 | ... | 547.687012 | 3.000000 | 97.226997 | 77.000000 | 112.362999 | 1346.000000 | 1062.750000 | 1.0 | 160.527776 | 213.305247 |
96701 | 94892 | NaN | NaN | NaN | NaN | NaN | NaN | NaN | NaN | NaN | ... | 457.170990 | 5.000000 | 0.000000 | 515.000000 | 38.470001 | 2364.000000 | 401.411011 | 2.0 | 410.039048 | 0.000000 |
96702 | 96021 | 180.378400 | 57.278130 | 0.000000 | 0.499708 | 4.000000 | 0.025284 | 1.000037 | 0.484826 | 10.717266 | ... | 327.584015 | 4.000000 | 251.115005 | 3.000000 | 153.737000 | 301.000000 | 2968.321045 | 0.0 | 710.912280 | 317.166726 |
96703 | 96702 | 54.747100 | 29.614573 | 0.000000 | 0.634613 | 4.000000 | 0.048043 | 1.000000 | 0.932525 | 5.238355 | ... | 41921.593750 | 0.000000 | 0.000000 | 4.000000 | 1227.026001 | 0.000000 | NaN | 0.0 | 0.000000 | 25185.139279 |
96704 rows × 119 columns
signatures["intid"] = signatures["id"].str[:-4].astype(int)
merge = dict(zip(signatures["intid"], signatures["id"]))
per_geometry["id"] = per_geometry["id"].map(merge)
per_geometry.drop(columns=["nodeID", "edgeID_primary"]).to_csv("../../urbangrammar_samba/spatial_signatures/data_product/per_geometry.csv", index=False)
pandas.Series(type_ids, name="type_code").to_csv("../../urbangrammar_samba/spatial_signatures/data_product/type_code.csv")
lsoa = pandas.read_csv("../../urbangrammar_samba/spatial_signatures/data_product/lsoa_estimates.csv", index_col=0)
lsoa.columns = lsoa.columns[:2].append(lsoa.columns.map(type_ids)[2:])
lsoa["primary_code"] = lsoa["primary_type"].map(type_ids)
lsoa[["LSOA11CD", "primary_code"] + list(lsoa.columns[1:-1])].to_csv("../../urbangrammar_samba/spatial_signatures/data_product/lsoa_estimates.csv", index=False)
oa = pandas.read_csv("../../urbangrammar_samba/spatial_signatures/data_product/output_area_estimates.csv", index_col=0)
oa.columns = oa.columns[:2].append(oa.columns.map(type_ids)[2:])
oa["primary_code"] = oa["primary_type"].map(type_ids)
oa
OA11CD | primary_type | NaN | NaN | NaN | NaN | NaN | NaN | NaN | NaN | ... | NaN | NaN | NaN | NaN | NaN | NaN | NaN | NaN | NaN | primary_code | |
---|---|---|---|---|---|---|---|---|---|---|---|---|---|---|---|---|---|---|---|---|---|
0 | E00000001 | Distilled urbanity | 0.000000 | 0.0 | 0.000000 | 0.000000 | 0.0 | 0.0 | 0.000000 | 0.0 | ... | 0.0 | 1.0 | 0.0 | 0.0 | 0.0 | 0.0 | DIU | DIU | DIU | DIU |
1 | E00000003 | Distilled urbanity | 0.000000 | 0.0 | 0.000000 | 0.000000 | 0.0 | 0.0 | 0.000000 | 0.0 | ... | 0.0 | 1.0 | 0.0 | 0.0 | 0.0 | 0.0 | DIU | DIU | DIU | DIU |
2 | E00000005 | Distilled urbanity | 0.000000 | 0.0 | 0.000000 | 0.000000 | 0.0 | 0.0 | 0.000000 | 0.0 | ... | 0.0 | 1.0 | 0.0 | 0.0 | 0.0 | 0.0 | DIU | DIU | DIU | DIU |
3 | E00000007 | Distilled urbanity | 0.000000 | 0.0 | 0.000000 | 0.000000 | 0.0 | 0.0 | 0.000000 | 0.0 | ... | 0.0 | 1.0 | 0.0 | 0.0 | 0.0 | 0.0 | DIU | DIU | DIU | DIU |
4 | E00000010 | Distilled urbanity | 0.000000 | 0.0 | 0.000000 | 0.000000 | 0.0 | 0.0 | 0.000000 | 0.0 | ... | 0.0 | 1.0 | 0.0 | 0.0 | 0.0 | 0.0 | DIU | DIU | DIU | DIU |
... | ... | ... | ... | ... | ... | ... | ... | ... | ... | ... | ... | ... | ... | ... | ... | ... | ... | ... | ... | ... | ... |
227754 | S00094726 | Wild countryside | 0.000000 | 0.0 | 0.000000 | 0.965738 | 0.0 | 0.0 | 0.000000 | 0.0 | ... | 0.0 | 0.0 | 0.0 | 0.0 | 0.0 | 0.0 | WIC | WIC | WIC | WIC |
227755 | S00102583 | Urban buffer | 0.000000 | 0.0 | 0.076019 | 0.000000 | 0.0 | 0.0 | 0.923981 | 0.0 | ... | 0.0 | 0.0 | 0.0 | 0.0 | 0.0 | 0.0 | URB | URB | URB | URB |
227756 | S00119179 | Wild countryside | 0.000000 | 0.0 | 0.000000 | 0.995102 | 0.0 | 0.0 | 0.000594 | 0.0 | ... | 0.0 | 0.0 | 0.0 | 0.0 | 0.0 | 0.0 | WIC | WIC | WIC | WIC |
227757 | S00119262 | Wild countryside | 0.000000 | 0.0 | 0.000000 | 0.982881 | 0.0 | 0.0 | 0.000000 | 0.0 | ... | 0.0 | 0.0 | 0.0 | 0.0 | 0.0 | 0.0 | WIC | WIC | WIC | WIC |
227758 | S00126169 | Countryside agriculture | 0.999624 | 0.0 | 0.000000 | 0.000377 | 0.0 | 0.0 | 0.000000 | 0.0 | ... | 0.0 | 0.0 | 0.0 | 0.0 | 0.0 | 0.0 | COA | COA | COA | COA |
227759 rows × 23 columns
oa = oa[list(oa.columns[:19]) + ["primary_code"]]
oa.columns = list(oa.columns[:2]) + list(lsoa.columns[2:])
oa[["OA11CD", "primary_code"] + list(oa.columns[1:-1])].to_csv("../../urbangrammar_samba/spatial_signatures/data_product/output_area_estimates.csv", index=False)
Not needed in this notebook but it is good to have them all at one place.
key_for_all = {
'population_q1': 'Population (Q1)',
'population_q2': 'Population (Q2)',
'population_q3': 'Population (Q3)',
'night_lights_q1': 'Night lights (Q1)',
'night_lights_q2': 'Night lights (Q2)',
'night_lights_q3': 'Night lights (Q3)',
'A, B, D, E. Agriculture, energy and water_q1': 'Workplace population [Agriculture, energy and water] (Q1)',
'A, B, D, E. Agriculture, energy and water_q2': 'Workplace population [Agriculture, energy and water] (Q2)',
'A, B, D, E. Agriculture, energy and water_q3': 'Workplace population [Agriculture, energy and water] (Q3)',
'C. Manufacturing_q1': 'Workplace population [Manufacturing] (Q1)',
'C. Manufacturing_q2': 'Workplace population [Manufacturing] (Q2)',
'C. Manufacturing_q3': 'Workplace population [Manufacturing] (Q3)',
'F. Construction_q1': 'Workplace population [Construction] (Q1)',
'F. Construction_q2': 'Workplace population [Construction] (Q2)',
'F. Construction_q3': 'Workplace population [Construction] (Q3)',
'G, I. Distribution, hotels and restaurants_q1': 'Workplace population [Distribution, hotels and restaurants] (Q1)',
'G, I. Distribution, hotels and restaurants_q2': 'Workplace population [Distribution, hotels and restaurants] (Q2)',
'G, I. Distribution, hotels and restaurants_q3': 'Workplace population [Distribution, hotels and restaurants] (Q3)',
'H, J. Transport and communication_q1': 'Workplace population [Transport and communication] (Q1)',
'H, J. Transport and communication_q2': 'Workplace population [Transport and communication] (Q2)',
'H, J. Transport and communication_q3': 'Workplace population [Transport and communication] (Q3)',
'K, L, M, N. Financial, real estate, professional and administrative activities_q1': 'Workplace population [Financial, real estate, professional and administrative activities] (Q1)',
'K, L, M, N. Financial, real estate, professional and administrative activities_q2': 'Workplace population [Financial, real estate, professional and administrative activities] (Q2)',
'K, L, M, N. Financial, real estate, professional and administrative activities_q3': 'Workplace population [Financial, real estate, professional and administrative activities] (Q3)',
'O,P,Q. Public administration, education and health_q1': 'Workplace population [Public administration, education and health] (Q1)',
'O,P,Q. Public administration, education and health_q2': 'Workplace population [Public administration, education and health] (Q2)',
'O,P,Q. Public administration, education and health_q3': 'Workplace population [Public administration, education and health] (Q3)',
'R, S, T, U. Other_q1': 'Workplace population [Other] (Q1)',
'R, S, T, U. Other_q2': 'Workplace population [Other] (Q2)',
'R, S, T, U. Other_q3': 'Workplace population [Other] (Q3)',
'Code_18_124_q1': 'Land cover [Airports] (Q1)',
'Code_18_124_q2': 'Land cover [Airports] (Q2)',
'Code_18_124_q3': 'Land cover [Airports] (Q3)',
'Code_18_211_q1': 'Land cover [Non-irrigated arable land] (Q1)',
'Code_18_211_q2': 'Land cover [Non-irrigated arable land] (Q2)',
'Code_18_211_q3': 'Land cover [Non-irrigated arable land] (Q3)',
'Code_18_121_q1': 'Land cover [Industrial or commercial units] (Q1)',
'Code_18_121_q2': 'Land cover [Industrial or commercial units] (Q2)',
'Code_18_121_q3': 'Land cover [Industrial or commercial units] (Q3)',
'Code_18_421_q1': 'Land cover [Salt marshes] (Q1)',
'Code_18_421_q2': 'Land cover [Salt marshes] (Q2)',
'Code_18_421_q3': 'Land cover [Salt marshes] (Q3)',
'Code_18_522_q1': 'Land cover [Estuaries] (Q1)',
'Code_18_522_q2': 'Land cover [Estuaries] (Q2)',
'Code_18_522_q3': 'Land cover [Estuaries] (Q3)',
'Code_18_142_q1': 'Land cover [Sport and leisure facilities] (Q1)',
'Code_18_142_q2': 'Land cover [Sport and leisure facilities] (Q2)',
'Code_18_142_q3': 'Land cover [Sport and leisure facilities] (Q3)',
'Code_18_141_q1': 'Land cover [Green urban areas] (Q1)',
'Code_18_141_q2': 'Land cover [Green urban areas] (Q2)',
'Code_18_141_q3': 'Land cover [Green urban areas] (Q3)',
'Code_18_112_q1': 'Land cover [Discontinuous urban fabric] (Q1)',
'Code_18_112_q2': 'Land cover [Discontinuous urban fabric] (Q2)',
'Code_18_112_q3': 'Land cover [Discontinuous urban fabric] (Q3)',
'Code_18_231_q1': 'Land cover [Pastures] (Q1)',
'Code_18_231_q2': 'Land cover [Pastures] (Q2)',
'Code_18_231_q3': 'Land cover [Pastures] (Q3)',
'Code_18_311_q1': 'Land cover [Broad-leaved forest] (Q1)',
'Code_18_311_q2': 'Land cover [Broad-leaved forest] (Q2)',
'Code_18_311_q3': 'Land cover [Broad-leaved forest] (Q3)',
'Code_18_131_q1': 'Land cover [Mineral extraction sites] (Q1)',
'Code_18_131_q2': 'Land cover [Mineral extraction sites] (Q2)',
'Code_18_131_q3': 'Land cover [Mineral extraction sites] (Q3)',
'Code_18_123_q1': 'Land cover [Port areas] (Q1)',
'Code_18_123_q2': 'Land cover [Port areas] (Q2)',
'Code_18_123_q3': 'Land cover [Port areas] (Q3)',
'Code_18_122_q1': 'Land cover [Road and rail networks and associated land] (Q1)',
'Code_18_122_q2': 'Land cover [Road and rail networks and associated land] (Q2)',
'Code_18_122_q3': 'Land cover [Road and rail networks and associated land] (Q3)',
'Code_18_512_q1': 'Land cover [Water bodies] (Q1)',
'Code_18_512_q2': 'Land cover [Water bodies] (Q2)',
'Code_18_512_q3': 'Land cover [Water bodies] (Q3)',
'Code_18_243_q1': 'Land cover [Land principally occupied by agriculture, with significant areas of natural vegetation] (Q1)',
'Code_18_243_q2': 'Land cover [Land principally occupied by agriculture, with significant areas of natural vegetation] (Q2)',
'Code_18_243_q3': 'Land cover [Land principally occupied by agriculture, with significant areas of natural vegetation] (Q3)',
'Code_18_313_q1': 'Land cover [Mixed forest] (Q1)',
'Code_18_313_q2': 'Land cover [Mixed forest] (Q2)',
'Code_18_313_q3': 'Land cover [Mixed forest] (Q3)',
'Code_18_412_q1': 'Land cover [Peat bogs] (Q1)',
'Code_18_412_q2': 'Land cover [Peat bogs] (Q2)',
'Code_18_412_q3': 'Land cover [Peat bogs] (Q3)',
'Code_18_321_q1': 'Land cover [Natural grasslands] (Q1)',
'Code_18_321_q2': 'Land cover [Natural grasslands] (Q2)',
'Code_18_321_q3': 'Land cover [Natural grasslands] (Q3)',
'Code_18_322_q1': 'Land cover [Moors and heathland] (Q1)',
'Code_18_322_q2': 'Land cover [Moors and heathland] (Q2)',
'Code_18_322_q3': 'Land cover [Moors and heathland] (Q3)',
'Code_18_324_q1': 'Land cover [Transitional woodland-shrub] (Q1)',
'Code_18_324_q2': 'Land cover [Transitional woodland-shrub] (Q2)',
'Code_18_324_q3': 'Land cover [Transitional woodland-shrub] (Q3)',
'Code_18_111_q1': 'Land cover [Continuous urban fabric] (Q1)',
'Code_18_111_q2': 'Land cover [Continuous urban fabric] (Q2)',
'Code_18_111_q3': 'Land cover [Continuous urban fabric] (Q3)',
'Code_18_423_q1': 'Land cover [Intertidal flats] (Q1)',
'Code_18_423_q2': 'Land cover [Intertidal flats] (Q2)',
'Code_18_423_q3': 'Land cover [Intertidal flats] (Q3)',
'Code_18_523_q1': 'Land cover [Sea and ocean] (Q1)',
'Code_18_523_q2': 'Land cover [Sea and ocean] (Q2)',
'Code_18_523_q3': 'Land cover [Sea and ocean] (Q3)',
'Code_18_312_q1': 'Land cover [Coniferous forest] (Q1)',
'Code_18_312_q2': 'Land cover [Coniferous forest] (Q2)',
'Code_18_312_q3': 'Land cover [Coniferous forest] (Q3)',
'Code_18_133_q1': 'Land cover [Construction sites] (Q1)',
'Code_18_133_q2': 'Land cover [Construction sites] (Q2)',
'Code_18_133_q3': 'Land cover [Construction sites] (Q3)',
'Code_18_333_q1': 'Land cover [Sparsely vegetated areas] (Q1)',
'Code_18_333_q2': 'Land cover [Sparsely vegetated areas] (Q2)',
'Code_18_333_q3': 'Land cover [Sparsely vegetated areas] (Q3)',
'Code_18_332_q1': 'Land cover [Bare rocks] (Q1)',
'Code_18_332_q2': 'Land cover [Bare rocks] (Q2)',
'Code_18_332_q3': 'Land cover [Bare rocks] (Q3)',
'Code_18_411_q1': 'Land cover [Inland marshes] (Q1)',
'Code_18_411_q2': 'Land cover [Inland marshes] (Q2)',
'Code_18_411_q3': 'Land cover [Inland marshes] (Q3)',
'Code_18_132_q1': 'Land cover [Dump sites] (Q1)',
'Code_18_331_q2': 'Land cover [Beaches, dunes, sands] (Q2)',
'Code_18_222_q1': 'Land cover [Fruit trees and berry plantations] (Q1)',
'Code_18_511_q3': 'Land cover [Water courses] (Q3)',
'Code_18_242_q1': 'Land cover [Complex cultivation patterns] (Q1)',
'Code_18_511_q2': 'Land cover [Water courses] (Q2)',
'Code_18_242_q3': 'Land cover [Complex cultivation patterns] (Q3)',
'Code_18_331_q1': 'Land cover [Beaches, dunes, sands] (Q1)',
'Code_18_334_q2': 'Land cover [Burnt areas] (Q2)',
'Code_18_511_q1': 'Land cover [Water courses] (Q1)',
'Code_18_334_q1': 'Land cover [Burnt areas] (Q1)',
'Code_18_222_q3': 'Land cover [Fruit trees and berry plantations] (Q3)',
'Code_18_242_q2': 'Land cover [Complex cultivation patterns] (Q2)',
'Code_18_244_q3': 'Land cover [Agro-forestry areas] (Q3)',
'Code_18_521_q2': 'Land cover [Coastal lagoons] (Q2)',
'Code_18_334_q3': 'Land cover [Burnt areas] (Q3)',
'Code_18_244_q1': 'Land cover [Agro-forestry areas] (Q1)',
'Code_18_244_q2': 'Land cover [Agro-forestry areas] (Q2)',
'Code_18_331_q3': 'Land cover [Beaches, dunes, sands] (Q3)',
'Code_18_132_q2': 'Land cover [Dump sites] (Q2)',
'Code_18_132_q3': 'Land cover [Dump sites] (Q3)',
'Code_18_521_q1': 'Land cover [Coastal lagoons] (Q1)',
'Code_18_222_q2': 'Land cover [Fruit trees and berry plantations] (Q2)',
'Code_18_521_q3': 'Land cover [Coastal lagoons] (Q3)',
'mean_q1': 'NDVI (Q1)',
'mean_q2': 'NDVI (Q2)',
'mean_q3': 'NDVI (Q3)',
'supermarkets_nearest': 'Supermarkets [distance to nearest]',
'supermarkets_counts': 'Supermarkets [counts within 1200m]',
'listed_nearest': 'Listed buildings [distance to nearest]',
'listed_counts': 'Listed buildings [counts within 1200m]',
'fhrs_nearest': 'FHRS points [distance to nearest]',
'fhrs_counts': 'FHRS points [counts within 1200m]',
'culture_nearest': 'Cultural venues [distance to nearest]',
'culture_counts': 'Cultural venues [counts within 1200m]',
'nearest_water': 'Water bodies [distance to nearest]',
'nearest_retail_centre': 'Retail centres [distance to nearest]',
'sdbAre_q1': 'area of building (Q1)',
'sdbAre_q2': 'area of building (Q2)',
'sdbAre_q3': 'area of building (Q3)',
'sdbPer_q1': 'perimeter of building (Q1)',
'sdbPer_q2': 'perimeter of building (Q2)',
'sdbPer_q3': 'perimeter of building (Q3)',
'sdbCoA_q1': 'courtyard area of building (Q1)',
'sdbCoA_q2': 'courtyard area of building (Q2)',
'sdbCoA_q3': 'courtyard area of building (Q3)',
'ssbCCo_q1': 'circular compactness of building (Q1)',
'ssbCCo_q2': 'circular compactness of building (Q2)',
'ssbCCo_q3': 'circular compactness of building (Q3)',
'ssbCor_q1': 'corners of building (Q1)',
'ssbCor_q2': 'corners of building (Q2)',
'ssbCor_q3': 'corners of building (Q3)',
'ssbSqu_q1': 'squareness of building (Q1)',
'ssbSqu_q2': 'squareness of building (Q2)',
'ssbSqu_q3': 'squareness of building (Q3)',
'ssbERI_q1': 'equivalent rectangular index of building (Q1)',
'ssbERI_q2': 'equivalent rectangular index of building (Q2)',
'ssbERI_q3': 'equivalent rectangular index of building (Q3)',
'ssbElo_q1': 'elongation of building (Q1)',
'ssbElo_q2': 'elongation of building (Q2)',
'ssbElo_q3': 'elongation of building (Q3)',
'ssbCCM_q1': 'centroid - corner mean distance of building (Q1)',
'ssbCCM_q2': 'centroid - corner mean distance of building (Q2)',
'ssbCCM_q3': 'centroid - corner mean distance of building (Q3)',
'ssbCCD_q1': 'centroid - corner distance deviation of building (Q1)',
'ssbCCD_q2': 'centroid - corner distance deviation of building (Q2)',
'ssbCCD_q3': 'centroid - corner distance deviation of building (Q3)',
'stbOri_q1': 'orientation of building (Q1)',
'stbOri_q2': 'orientation of building (Q2)',
'stbOri_q3': 'orientation of building (Q3)',
'sdcLAL_q1': 'longest axis length of ETC (Q1)',
'sdcLAL_q2': 'longest axis length of ETC (Q2)',
'sdcLAL_q3': 'longest axis length of ETC (Q3)',
'sdcAre_q1': 'area of ETC (Q1)',
'sdcAre_q2': 'area of ETC (Q2)',
'sdcAre_q3': 'area of ETC (Q3)',
'sscCCo_q1': 'circular compactness of ETC (Q1)',
'sscCCo_q2': 'circular compactness of ETC (Q2)',
'sscCCo_q3': 'circular compactness of ETC (Q3)',
'sscERI_q1': 'equivalent rectangular index of ETC (Q1)',
'sscERI_q2': 'equivalent rectangular index of ETC (Q2)',
'sscERI_q3': 'equivalent rectangular index of ETC (Q3)',
'stcOri_q1': 'orientation of ETC (Q1)',
'stcOri_q2': 'orientation of ETC (Q2)',
'stcOri_q3': 'orientation of ETC (Q3)',
'sicCAR_q1': 'covered area ratio of ETC (Q1)',
'sicCAR_q2': 'covered area ratio of ETC (Q2)',
'sicCAR_q3': 'covered area ratio of ETC (Q3)',
'stbCeA_q1': 'cell alignment of building (Q1)',
'stbCeA_q2': 'cell alignment of building (Q2)',
'stbCeA_q3': 'cell alignment of building (Q3)',
'mtbAli_q1': 'alignment of neighbouring buildings (Q1)',
'mtbAli_q2': 'alignment of neighbouring buildings (Q2)',
'mtbAli_q3': 'alignment of neighbouring buildings (Q3)',
'mtbNDi_q1': 'mean distance between neighbouring buildings (Q1)',
'mtbNDi_q2': 'mean distance between neighbouring buildings (Q2)',
'mtbNDi_q3': 'mean distance between neighbouring buildings (Q3)',
'mtcWNe_q1': 'perimeter-weighted neighbours of ETC (Q1)',
'mtcWNe_q2': 'perimeter-weighted neighbours of ETC (Q2)',
'mtcWNe_q3': 'perimeter-weighted neighbours of ETC (Q3)',
'mdcAre_q1': 'area covered by neighbouring cells (Q1)',
'mdcAre_q2': 'area covered by neighbouring cells (Q2)',
'mdcAre_q3': 'area covered by neighbouring cells (Q3)',
'ltcWRE_q1': 'weighted reached enclosures of ETC (Q1)',
'ltcWRE_q2': 'weighted reached enclosures of ETC (Q2)',
'ltcWRE_q3': 'weighted reached enclosures of ETC (Q3)',
'ltbIBD_q1': 'mean inter-building distance (Q1)',
'ltbIBD_q2': 'mean inter-building distance (Q2)',
'ltbIBD_q3': 'mean inter-building distance (Q3)',
'sdsSPW_q1': 'width of street profile (Q1)',
'sdsSPW_q2': 'width of street profile (Q2)',
'sdsSPW_q3': 'width of street profile (Q3)',
'sdsSWD_q1': 'width deviation of street profile (Q1)',
'sdsSWD_q2': 'width deviation of street profile (Q2)',
'sdsSWD_q3': 'width deviation of street profile (Q3)',
'sdsSPO_q1': 'openness of street profile (Q1)',
'sdsSPO_q2': 'openness of street profile (Q2)',
'sdsSPO_q3': 'openness of street profile (Q3)',
'sdsLen_q1': 'length of street segment (Q1)',
'sdsLen_q2': 'length of street segment (Q2)',
'sdsLen_q3': 'length of street segment (Q3)',
'sssLin_q1': 'linearity of street segment (Q1)',
'sssLin_q2': 'linearity of street segment (Q2)',
'sssLin_q3': 'linearity of street segment (Q3)',
'ldsMSL_q1': 'mean segment length within 3 steps (Q1)',
'ldsMSL_q2': 'mean segment length within 3 steps (Q2)',
'ldsMSL_q3': 'mean segment length within 3 steps (Q3)',
'mtdDeg_q1': 'node degree of junction (Q1)',
'mtdDeg_q2': 'node degree of junction (Q2)',
'mtdDeg_q3': 'node degree of junction (Q3)',
'lcdMes_q1': 'local meshedness of street network (Q1)',
'lcdMes_q2': 'local meshedness of street network (Q2)',
'lcdMes_q3': 'local meshedness of street network (Q3)',
'linP3W_q1': 'local proportion of 3-way intersections of street network (Q1)',
'linP3W_q2': 'local proportion of 3-way intersections of street network (Q2)',
'linP3W_q3': 'local proportion of 3-way intersections of street network (Q3)',
'linP4W_q1': 'local proportion of 4-way intersections of street network (Q1)',
'linP4W_q2': 'local proportion of 4-way intersections of street network (Q2)',
'linP4W_q3': 'local proportion of 4-way intersections of street network (Q3)',
'linPDE_q1': 'local proportion of cul-de-sacs of street network (Q1)',
'linPDE_q2': 'local proportion of cul-de-sacs of street network (Q2)',
'linPDE_q3': 'local proportion of cul-de-sacs of street network (Q3)',
'lcnClo_q1': 'local closeness of street network (Q1)',
'lcnClo_q2': 'local closeness of street network (Q2)',
'lcnClo_q3': 'local closeness of street network (Q3)',
'ldsCDL_q1': 'local cul-de-sac length of street network (Q1)',
'ldsCDL_q2': 'local cul-de-sac length of street network (Q2)',
'ldsCDL_q3': 'local cul-de-sac length of street network (Q3)',
'xcnSCl_q1': 'square clustering of street network (Q1)',
'xcnSCl_q2': 'square clustering of street network (Q2)',
'xcnSCl_q3': 'square clustering of street network (Q3)',
'mtdMDi_q1': 'mean distance to neighbouring nodes of street network (Q1)',
'mtdMDi_q2': 'mean distance to neighbouring nodes of street network (Q2)',
'mtdMDi_q3': 'mean distance to neighbouring nodes of street network (Q3)',
'lddNDe_q1': 'local node density of street network (Q1)',
'lddNDe_q2': 'local node density of street network (Q2)',
'lddNDe_q3': 'local node density of street network (Q3)',
'linWID_q1': 'local degree weighted node density of street network (Q1)',
'linWID_q2': 'local degree weighted node density of street network (Q2)',
'linWID_q3': 'local degree weighted node density of street network (Q3)',
'stbSAl_q1': 'street alignment of building (Q1)',
'stbSAl_q2': 'street alignment of building (Q2)',
'stbSAl_q3': 'street alignment of building (Q3)',
'sddAre_q1': 'area covered by node-attached ETCs (Q1)',
'sddAre_q2': 'area covered by node-attached ETCs (Q2)',
'sddAre_q3': 'area covered by node-attached ETCs (Q3)',
'sdsAre_q1': 'area covered by edge-attached ETCs (Q1)',
'sdsAre_q2': 'area covered by edge-attached ETCs (Q2)',
'sdsAre_q3': 'area covered by edge-attached ETCs (Q3)',
'sisBpM_q1': 'buildings per meter of street segment (Q1)',
'sisBpM_q2': 'buildings per meter of street segment (Q2)',
'sisBpM_q3': 'buildings per meter of street segment (Q3)',
'misCel_q1': 'reached ETCs by neighbouring segments (Q1)',
'misCel_q2': 'reached ETCs by neighbouring segments (Q2)',
'misCel_q3': 'reached ETCs by neighbouring segments (Q3)',
'mdsAre_q1': 'reached area by neighbouring segments (Q1)',
'mdsAre_q2': 'reached area by neighbouring segments (Q2)',
'mdsAre_q3': 'reached area by neighbouring segments (Q3)',
'lisCel_q1': 'reached ETCs by local street network (Q1)',
'lisCel_q2': 'reached ETCs by local street network (Q2)',
'lisCel_q3': 'reached ETCs by local street network (Q3)',
'ldsAre_q1': 'reached area by local street network (Q1)',
'ldsAre_q2': 'reached area by local street network (Q2)',
'ldsAre_q3': 'reached area by local street network (Q3)',
'ltcRea_q1': 'reached ETCs by tessellation contiguity (Q1)',
'ltcRea_q2': 'reached ETCs by tessellation contiguity (Q2)',
'ltcRea_q3': 'reached ETCs by tessellation contiguity (Q3)',
'ltcAre_q1': 'reached area by tessellation contiguity (Q1)',
'ltcAre_q2': 'reached area by tessellation contiguity (Q2)',
'ltcAre_q3': 'reached area by tessellation contiguity (Q3)',
'ldeAre_q1': 'area of enclosure (Q1)',
'ldeAre_q2': 'area of enclosure (Q2)',
'ldeAre_q3': 'area of enclosure (Q3)',
'ldePer_q1': 'perimeter of enclosure (Q1)',
'ldePer_q2': 'perimeter of enclosure (Q2)',
'ldePer_q3': 'perimeter of enclosure (Q3)',
'lseCCo_q1': 'circular compactness of enclosure (Q1)',
'lseCCo_q2': 'circular compactness of enclosure (Q2)',
'lseCCo_q3': 'circular compactness of enclosure (Q3)',
'lseERI_q1': 'equivalent rectangular index of enclosure (Q1)',
'lseERI_q2': 'equivalent rectangular index of enclosure (Q2)',
'lseERI_q3': 'equivalent rectangular index of enclosure (Q3)',
'lseCWA_q1': 'compactness-weighted axis of enclosure (Q1)',
'lseCWA_q2': 'compactness-weighted axis of enclosure (Q2)',
'lseCWA_q3': 'compactness-weighted axis of enclosure (Q3)',
'lteOri_q1': 'orientation of enclosure (Q1)',
'lteOri_q2': 'orientation of enclosure (Q2)',
'lteOri_q3': 'orientation of enclosure (Q3)',
'lteWNB_q1': 'perimeter-weighted neighbours of enclosure (Q1)',
'lteWNB_q2': 'perimeter-weighted neighbours of enclosure (Q2)',
'lteWNB_q3': 'perimeter-weighted neighbours of enclosure (Q3)',
'lieWCe_q1': 'area-weighted ETCs of enclosure (Q1)',
'lieWCe_q2': 'area-weighted ETCs of enclosure (Q2)',
'lieWCe_q3': 'area-weighted ETCs of enclosure (Q3)',
}
Dump ETCs to an archive repository as a partitioned parquet¶
Create partitioned ETC-level datasets to be stored in an archival GitHub repository.
from dask.distributed import Client, LocalCluster
client = Client(LocalCluster(n_workers=8))
client
Client
Client-8c657e0b-94bf-11ec-840d-115a56b33b25
Connection method: Cluster object | Cluster type: distributed.LocalCluster |
Dashboard: http://127.0.0.1:8787/status |
Cluster Info
LocalCluster
077353cd
Dashboard: http://127.0.0.1:8787/status | Workers: 8 |
Total threads: 16 | Total memory: 125.54 GiB |
Status: running | Using processes: True |
Scheduler Info
Scheduler
Scheduler-6c5cf5f9-8b46-49f3-92f3-a146dc26f205
Comm: tcp://127.0.0.1:41533 | Workers: 8 |
Dashboard: http://127.0.0.1:8787/status | Total threads: 16 |
Started: Just now | Total memory: 125.54 GiB |
Workers
Worker: 0
Comm: tcp://127.0.0.1:45761 | Total threads: 2 |
Dashboard: http://127.0.0.1:45937/status | Memory: 15.69 GiB |
Nanny: tcp://127.0.0.1:46555 | |
Local directory: /home/jovyan/work/spatial_signatures/data_product/dask-worker-space/worker-bi7gc9f7 |
Worker: 1
Comm: tcp://127.0.0.1:43561 | Total threads: 2 |
Dashboard: http://127.0.0.1:42629/status | Memory: 15.69 GiB |
Nanny: tcp://127.0.0.1:37275 | |
Local directory: /home/jovyan/work/spatial_signatures/data_product/dask-worker-space/worker-6nb7xvam |
Worker: 2
Comm: tcp://127.0.0.1:34809 | Total threads: 2 |
Dashboard: http://127.0.0.1:42191/status | Memory: 15.69 GiB |
Nanny: tcp://127.0.0.1:40163 | |
Local directory: /home/jovyan/work/spatial_signatures/data_product/dask-worker-space/worker-6eu74kse |
Worker: 3
Comm: tcp://127.0.0.1:42791 | Total threads: 2 |
Dashboard: http://127.0.0.1:46581/status | Memory: 15.69 GiB |
Nanny: tcp://127.0.0.1:35041 | |
Local directory: /home/jovyan/work/spatial_signatures/data_product/dask-worker-space/worker-2q93e4x0 |
Worker: 4
Comm: tcp://127.0.0.1:45789 | Total threads: 2 |
Dashboard: http://127.0.0.1:39645/status | Memory: 15.69 GiB |
Nanny: tcp://127.0.0.1:32919 | |
Local directory: /home/jovyan/work/spatial_signatures/data_product/dask-worker-space/worker-2p6xt4ln |
Worker: 5
Comm: tcp://127.0.0.1:34877 | Total threads: 2 |
Dashboard: http://127.0.0.1:46445/status | Memory: 15.69 GiB |
Nanny: tcp://127.0.0.1:44617 | |
Local directory: /home/jovyan/work/spatial_signatures/data_product/dask-worker-space/worker-0mi2h2tv |
Worker: 6
Comm: tcp://127.0.0.1:46809 | Total threads: 2 |
Dashboard: http://127.0.0.1:46797/status | Memory: 15.69 GiB |
Nanny: tcp://127.0.0.1:38497 | |
Local directory: /home/jovyan/work/spatial_signatures/data_product/dask-worker-space/worker-l5q90poh |
Worker: 7
Comm: tcp://127.0.0.1:44479 | Total threads: 2 |
Dashboard: http://127.0.0.1:35383/status | Memory: 15.69 GiB |
Nanny: tcp://127.0.0.1:43407 | |
Local directory: /home/jovyan/work/spatial_signatures/data_product/dask-worker-space/worker-gfz82bz3 |
include = ['hindex', 'tessellation', 'sdbAre', 'sdbPer', 'sdbCoA', 'ssbCCo', 'ssbCor', 'ssbSqu', 'ssbERI', 'ssbElo',
'ssbCCM', 'ssbCCD', 'stbOri', 'sdcLAL', 'sdcAre', 'sscCCo', 'sscERI', 'stcOri',
'sicCAR', 'stbCeA', 'mtbAli', 'mtbNDi', 'mtcWNe', 'mdcAre', 'ltcWRE', 'ltbIBD',
'sdsSPW', 'sdsSWD', 'sdsSPO', 'sdsLen', 'sssLin', 'ldsMSL', 'mtdDeg', 'lcdMes',
'linP3W', 'linP4W', 'linPDE', 'lcnClo', 'ldsCDL', 'xcnSCl', 'mtdMDi', 'lddNDe',
'linWID', 'stbSAl', 'sddAre', 'sdsAre', 'sisBpM', 'misCel', 'mdsAre', 'lisCel',
'ldsAre', 'ltcRea', 'ltcAre', 'ldeAre', 'ldePer', 'lseCCo', 'lseERI', 'lseCWA',
'lteOri', 'lteWNB', 'lieWCe'
]
primary = dask_geopandas.read_parquet("../../urbangrammar_samba/spatial_signatures/morphometrics/cells/*", columns=include)
primary
hindex | tessellation | sdbAre | sdbPer | sdbCoA | ssbCCo | ssbCor | ssbSqu | ssbERI | ssbElo | ssbCCM | ssbCCD | stbOri | sdcLAL | sdcAre | sscCCo | sscERI | stcOri | sicCAR | stbCeA | mtbAli | mtbNDi | mtcWNe | mdcAre | ltcWRE | ltbIBD | sdsSPW | sdsSWD | sdsSPO | sdsLen | sssLin | ldsMSL | mtdDeg | lcdMes | linP3W | linP4W | linPDE | lcnClo | ldsCDL | xcnSCl | mtdMDi | lddNDe | linWID | stbSAl | sddAre | sdsAre | sisBpM | misCel | mdsAre | lisCel | ldsAre | ltcRea | ltcAre | ldeAre | ldePer | lseCCo | lseERI | lseCWA | lteOri | lteWNB | lieWCe | |
---|---|---|---|---|---|---|---|---|---|---|---|---|---|---|---|---|---|---|---|---|---|---|---|---|---|---|---|---|---|---|---|---|---|---|---|---|---|---|---|---|---|---|---|---|---|---|---|---|---|---|---|---|---|---|---|---|---|---|---|---|---|
npartitions=103 | |||||||||||||||||||||||||||||||||||||||||||||||||||||||||||||
object | geometry | float64 | float64 | float64 | float64 | float64 | float64 | float64 | float64 | float64 | float64 | float64 | float64 | float64 | float64 | float64 | float64 | float64 | float64 | float64 | float64 | float64 | float64 | float64 | float64 | float64 | float64 | float64 | float64 | float64 | float64 | int64 | float64 | float64 | float64 | float64 | float64 | float64 | float64 | float64 | float64 | float64 | float64 | float64 | float64 | float64 | float64 | float64 | float64 | float64 | int64 | float64 | float64 | float64 | float64 | float64 | float64 | float64 | float64 | float64 | |
... | ... | ... | ... | ... | ... | ... | ... | ... | ... | ... | ... | ... | ... | ... | ... | ... | ... | ... | ... | ... | ... | ... | ... | ... | ... | ... | ... | ... | ... | ... | ... | ... | ... | ... | ... | ... | ... | ... | ... | ... | ... | ... | ... | ... | ... | ... | ... | ... | ... | ... | ... | ... | ... | ... | ... | ... | ... | ... | ... | ... | |
... | ... | ... | ... | ... | ... | ... | ... | ... | ... | ... | ... | ... | ... | ... | ... | ... | ... | ... | ... | ... | ... | ... | ... | ... | ... | ... | ... | ... | ... | ... | ... | ... | ... | ... | ... | ... | ... | ... | ... | ... | ... | ... | ... | ... | ... | ... | ... | ... | ... | ... | ... | ... | ... | ... | ... | ... | ... | ... | ... | ... | ... |
... | ... | ... | ... | ... | ... | ... | ... | ... | ... | ... | ... | ... | ... | ... | ... | ... | ... | ... | ... | ... | ... | ... | ... | ... | ... | ... | ... | ... | ... | ... | ... | ... | ... | ... | ... | ... | ... | ... | ... | ... | ... | ... | ... | ... | ... | ... | ... | ... | ... | ... | ... | ... | ... | ... | ... | ... | ... | ... | ... | ... | |
... | ... | ... | ... | ... | ... | ... | ... | ... | ... | ... | ... | ... | ... | ... | ... | ... | ... | ... | ... | ... | ... | ... | ... | ... | ... | ... | ... | ... | ... | ... | ... | ... | ... | ... | ... | ... | ... | ... | ... | ... | ... | ... | ... | ... | ... | ... | ... | ... | ... | ... | ... | ... | ... | ... | ... | ... | ... | ... | ... | ... |
conv = dask.dataframe.read_parquet(f"../../urbangrammar_samba/spatial_signatures/morphometrics/convolutions/*")
conv
sdbAre_q1 | sdbAre_q2 | sdbAre_q3 | sdbPer_q1 | sdbPer_q2 | sdbPer_q3 | sdbCoA_q1 | sdbCoA_q2 | sdbCoA_q3 | ssbCCo_q1 | ssbCCo_q2 | ssbCCo_q3 | ssbCor_q1 | ssbCor_q2 | ssbCor_q3 | ssbSqu_q1 | ssbSqu_q2 | ssbSqu_q3 | ssbERI_q1 | ssbERI_q2 | ssbERI_q3 | ssbElo_q1 | ssbElo_q2 | ssbElo_q3 | ssbCCM_q1 | ssbCCM_q2 | ssbCCM_q3 | ssbCCD_q1 | ssbCCD_q2 | ssbCCD_q3 | stbOri_q1 | stbOri_q2 | stbOri_q3 | sdcLAL_q1 | sdcLAL_q2 | sdcLAL_q3 | sdcAre_q1 | sdcAre_q2 | sdcAre_q3 | sscCCo_q1 | sscCCo_q2 | sscCCo_q3 | sscERI_q1 | sscERI_q2 | sscERI_q3 | stcOri_q1 | stcOri_q2 | stcOri_q3 | sicCAR_q1 | sicCAR_q2 | sicCAR_q3 | stbCeA_q1 | stbCeA_q2 | stbCeA_q3 | mtbAli_q1 | mtbAli_q2 | mtbAli_q3 | mtbNDi_q1 | mtbNDi_q2 | mtbNDi_q3 | mtcWNe_q1 | mtcWNe_q2 | mtcWNe_q3 | mdcAre_q1 | mdcAre_q2 | mdcAre_q3 | ltcWRE_q1 | ltcWRE_q2 | ltcWRE_q3 | ltbIBD_q1 | ltbIBD_q2 | ltbIBD_q3 | sdsSPW_q1 | sdsSPW_q2 | sdsSPW_q3 | sdsSWD_q1 | sdsSWD_q2 | sdsSWD_q3 | sdsSPO_q1 | sdsSPO_q2 | sdsSPO_q3 | sdsLen_q1 | sdsLen_q2 | sdsLen_q3 | sssLin_q1 | sssLin_q2 | sssLin_q3 | ldsMSL_q1 | ldsMSL_q2 | ldsMSL_q3 | mtdDeg_q1 | mtdDeg_q2 | mtdDeg_q3 | lcdMes_q1 | lcdMes_q2 | lcdMes_q3 | linP3W_q1 | linP3W_q2 | linP3W_q3 | linP4W_q1 | linP4W_q2 | linP4W_q3 | linPDE_q1 | linPDE_q2 | linPDE_q3 | lcnClo_q1 | lcnClo_q2 | lcnClo_q3 | ldsCDL_q1 | ldsCDL_q2 | ldsCDL_q3 | xcnSCl_q1 | xcnSCl_q2 | xcnSCl_q3 | mtdMDi_q1 | mtdMDi_q2 | mtdMDi_q3 | lddNDe_q1 | lddNDe_q2 | lddNDe_q3 | linWID_q1 | linWID_q2 | linWID_q3 | stbSAl_q1 | stbSAl_q2 | stbSAl_q3 | sddAre_q1 | sddAre_q2 | sddAre_q3 | sdsAre_q1 | sdsAre_q2 | sdsAre_q3 | sisBpM_q1 | sisBpM_q2 | sisBpM_q3 | misCel_q1 | misCel_q2 | misCel_q3 | mdsAre_q1 | mdsAre_q2 | mdsAre_q3 | lisCel_q1 | lisCel_q2 | lisCel_q3 | ldsAre_q1 | ldsAre_q2 | ldsAre_q3 | ltcRea_q1 | ltcRea_q2 | ltcRea_q3 | ltcAre_q1 | ltcAre_q2 | ltcAre_q3 | ldeAre_q1 | ldeAre_q2 | ldeAre_q3 | ldePer_q1 | ldePer_q2 | ldePer_q3 | lseCCo_q1 | lseCCo_q2 | lseCCo_q3 | lseERI_q1 | lseERI_q2 | lseERI_q3 | lseCWA_q1 | lseCWA_q2 | lseCWA_q3 | lteOri_q1 | lteOri_q2 | lteOri_q3 | lteWNB_q1 | lteWNB_q2 | lteWNB_q3 | lieWCe_q1 | lieWCe_q2 | lieWCe_q3 | |
---|---|---|---|---|---|---|---|---|---|---|---|---|---|---|---|---|---|---|---|---|---|---|---|---|---|---|---|---|---|---|---|---|---|---|---|---|---|---|---|---|---|---|---|---|---|---|---|---|---|---|---|---|---|---|---|---|---|---|---|---|---|---|---|---|---|---|---|---|---|---|---|---|---|---|---|---|---|---|---|---|---|---|---|---|---|---|---|---|---|---|---|---|---|---|---|---|---|---|---|---|---|---|---|---|---|---|---|---|---|---|---|---|---|---|---|---|---|---|---|---|---|---|---|---|---|---|---|---|---|---|---|---|---|---|---|---|---|---|---|---|---|---|---|---|---|---|---|---|---|---|---|---|---|---|---|---|---|---|---|---|---|---|---|---|---|---|---|---|---|---|---|---|---|---|---|---|---|
npartitions=103 | |||||||||||||||||||||||||||||||||||||||||||||||||||||||||||||||||||||||||||||||||||||||||||||||||||||||||||||||||||||||||||||||||||||||||||||||||||||||||||||||||||||||||||||||||
float64 | float64 | float64 | float64 | float64 | float64 | float64 | float64 | float64 | float64 | float64 | float64 | float64 | float64 | float64 | float64 | float64 | float64 | float64 | float64 | float64 | float64 | float64 | float64 | float64 | float64 | float64 | float64 | float64 | float64 | float64 | float64 | float64 | float64 | float64 | float64 | float64 | float64 | float64 | float64 | float64 | float64 | float64 | float64 | float64 | float64 | float64 | float64 | float64 | float64 | float64 | float64 | float64 | float64 | float64 | float64 | float64 | float64 | float64 | float64 | float64 | float64 | float64 | float64 | float64 | float64 | float64 | float64 | float64 | float64 | float64 | float64 | float64 | float64 | float64 | float64 | float64 | float64 | float64 | float64 | float64 | float64 | float64 | float64 | float64 | float64 | float64 | float64 | float64 | float64 | float64 | float64 | float64 | float64 | float64 | float64 | float64 | float64 | float64 | float64 | float64 | float64 | float64 | float64 | float64 | float64 | float64 | float64 | float64 | float64 | float64 | float64 | float64 | float64 | float64 | float64 | float64 | float64 | float64 | float64 | float64 | float64 | float64 | float64 | float64 | float64 | float64 | float64 | float64 | float64 | float64 | float64 | float64 | float64 | float64 | float64 | float64 | float64 | float64 | float64 | float64 | float64 | float64 | float64 | float64 | float64 | float64 | float64 | float64 | float64 | float64 | float64 | float64 | float64 | float64 | float64 | float64 | float64 | float64 | float64 | float64 | float64 | float64 | float64 | float64 | float64 | float64 | float64 | float64 | float64 | float64 | float64 | float64 | float64 | float64 | float64 | float64 | |
... | ... | ... | ... | ... | ... | ... | ... | ... | ... | ... | ... | ... | ... | ... | ... | ... | ... | ... | ... | ... | ... | ... | ... | ... | ... | ... | ... | ... | ... | ... | ... | ... | ... | ... | ... | ... | ... | ... | ... | ... | ... | ... | ... | ... | ... | ... | ... | ... | ... | ... | ... | ... | ... | ... | ... | ... | ... | ... | ... | ... | ... | ... | ... | ... | ... | ... | ... | ... | ... | ... | ... | ... | ... | ... | ... | ... | ... | ... | ... | ... | ... | ... | ... | ... | ... | ... | ... | ... | ... | ... | ... | ... | ... | ... | ... | ... | ... | ... | ... | ... | ... | ... | ... | ... | ... | ... | ... | ... | ... | ... | ... | ... | ... | ... | ... | ... | ... | ... | ... | ... | ... | ... | ... | ... | ... | ... | ... | ... | ... | ... | ... | ... | ... | ... | ... | ... | ... | ... | ... | ... | ... | ... | ... | ... | ... | ... | ... | ... | ... | ... | ... | ... | ... | ... | ... | ... | ... | ... | ... | ... | ... | ... | ... | ... | ... | ... | ... | ... | ... | ... | ... | ... | ... | ... | ... | ... | |
... | ... | ... | ... | ... | ... | ... | ... | ... | ... | ... | ... | ... | ... | ... | ... | ... | ... | ... | ... | ... | ... | ... | ... | ... | ... | ... | ... | ... | ... | ... | ... | ... | ... | ... | ... | ... | ... | ... | ... | ... | ... | ... | ... | ... | ... | ... | ... | ... | ... | ... | ... | ... | ... | ... | ... | ... | ... | ... | ... | ... | ... | ... | ... | ... | ... | ... | ... | ... | ... | ... | ... | ... | ... | ... | ... | ... | ... | ... | ... | ... | ... | ... | ... | ... | ... | ... | ... | ... | ... | ... | ... | ... | ... | ... | ... | ... | ... | ... | ... | ... | ... | ... | ... | ... | ... | ... | ... | ... | ... | ... | ... | ... | ... | ... | ... | ... | ... | ... | ... | ... | ... | ... | ... | ... | ... | ... | ... | ... | ... | ... | ... | ... | ... | ... | ... | ... | ... | ... | ... | ... | ... | ... | ... | ... | ... | ... | ... | ... | ... | ... | ... | ... | ... | ... | ... | ... | ... | ... | ... | ... | ... | ... | ... | ... | ... | ... | ... | ... | ... | ... | ... | ... | ... | ... | ... | ... | ... |
... | ... | ... | ... | ... | ... | ... | ... | ... | ... | ... | ... | ... | ... | ... | ... | ... | ... | ... | ... | ... | ... | ... | ... | ... | ... | ... | ... | ... | ... | ... | ... | ... | ... | ... | ... | ... | ... | ... | ... | ... | ... | ... | ... | ... | ... | ... | ... | ... | ... | ... | ... | ... | ... | ... | ... | ... | ... | ... | ... | ... | ... | ... | ... | ... | ... | ... | ... | ... | ... | ... | ... | ... | ... | ... | ... | ... | ... | ... | ... | ... | ... | ... | ... | ... | ... | ... | ... | ... | ... | ... | ... | ... | ... | ... | ... | ... | ... | ... | ... | ... | ... | ... | ... | ... | ... | ... | ... | ... | ... | ... | ... | ... | ... | ... | ... | ... | ... | ... | ... | ... | ... | ... | ... | ... | ... | ... | ... | ... | ... | ... | ... | ... | ... | ... | ... | ... | ... | ... | ... | ... | ... | ... | ... | ... | ... | ... | ... | ... | ... | ... | ... | ... | ... | ... | ... | ... | ... | ... | ... | ... | ... | ... | ... | ... | ... | ... | ... | ... | ... | ... | ... | ... | ... | ... | ... | ... | |
... | ... | ... | ... | ... | ... | ... | ... | ... | ... | ... | ... | ... | ... | ... | ... | ... | ... | ... | ... | ... | ... | ... | ... | ... | ... | ... | ... | ... | ... | ... | ... | ... | ... | ... | ... | ... | ... | ... | ... | ... | ... | ... | ... | ... | ... | ... | ... | ... | ... | ... | ... | ... | ... | ... | ... | ... | ... | ... | ... | ... | ... | ... | ... | ... | ... | ... | ... | ... | ... | ... | ... | ... | ... | ... | ... | ... | ... | ... | ... | ... | ... | ... | ... | ... | ... | ... | ... | ... | ... | ... | ... | ... | ... | ... | ... | ... | ... | ... | ... | ... | ... | ... | ... | ... | ... | ... | ... | ... | ... | ... | ... | ... | ... | ... | ... | ... | ... | ... | ... | ... | ... | ... | ... | ... | ... | ... | ... | ... | ... | ... | ... | ... | ... | ... | ... | ... | ... | ... | ... | ... | ... | ... | ... | ... | ... | ... | ... | ... | ... | ... | ... | ... | ... | ... | ... | ... | ... | ... | ... | ... | ... | ... | ... | ... | ... | ... | ... | ... | ... | ... | ... | ... | ... | ... | ... | ... |
renamer = {**{k:key[v] for k, v in coded.items()}, **key_for_all}
dask.dataframe.multi.concat([primary, conv], axis=1).rename(columns=renamer).repartition(npartitions=1000).to_parquet("../../signatures_gb/form")
function = dask.dataframe.read_parquet("../../urbangrammar_samba/spatial_signatures/functional/combined_raw").reset_index(drop=True)
function
hindex | population | night_lights | A, B, D, E. Agriculture, energy and water | C. Manufacturing | F. Construction | G, I. Distribution, hotels and restaurants | H, J. Transport and communication | K, L, M, N. Financial, real estate, professional and administrative activities | O,P,Q. Public administration, education and health | R, S, T, U. Other | Code_18_124 | Code_18_211 | Code_18_121 | Code_18_421 | Code_18_522 | Code_18_142 | Code_18_141 | Code_18_112 | Code_18_231 | Code_18_311 | Code_18_131 | Code_18_123 | Code_18_122 | Code_18_512 | Code_18_243 | Code_18_313 | Code_18_412 | Code_18_321 | Code_18_322 | Code_18_324 | Code_18_111 | Code_18_423 | Code_18_523 | Code_18_133 | Code_18_334 | Code_18_132 | Code_18_242 | Code_18_411 | Code_18_511 | Code_18_312 | Code_18_332 | Code_18_521 | Code_18_331 | Code_18_244 | Code_18_333 | Code_18_222 | mean | supermarkets_nearest | supermarkets_counts | listed_nearest | listed_counts | fhrs_nearest | fhrs_counts | culture_nearest | culture_counts | nearest_water | nearest_retail_centre | |
---|---|---|---|---|---|---|---|---|---|---|---|---|---|---|---|---|---|---|---|---|---|---|---|---|---|---|---|---|---|---|---|---|---|---|---|---|---|---|---|---|---|---|---|---|---|---|---|---|---|---|---|---|---|---|---|---|---|---|
npartitions=103 | ||||||||||||||||||||||||||||||||||||||||||||||||||||||||||
object | float64 | float64 | float64 | float64 | float64 | float64 | float64 | float64 | float64 | float64 | float64 | float64 | float64 | float64 | float64 | float64 | float64 | float64 | float64 | float64 | float64 | float64 | float64 | float64 | float64 | float64 | float64 | float64 | float64 | float64 | float64 | float64 | float64 | int64 | int64 | int64 | int64 | int64 | int64 | int64 | int64 | int64 | int64 | int64 | int64 | int64 | float64 | float64 | int64 | float64 | int64 | float64 | int64 | float64 | int64 | float64 | float64 | |
... | ... | ... | ... | ... | ... | ... | ... | ... | ... | ... | ... | ... | ... | ... | ... | ... | ... | ... | ... | ... | ... | ... | ... | ... | ... | ... | ... | ... | ... | ... | ... | ... | ... | ... | ... | ... | ... | ... | ... | ... | ... | ... | ... | ... | ... | ... | ... | ... | ... | ... | ... | ... | ... | ... | ... | ... | ... | |
... | ... | ... | ... | ... | ... | ... | ... | ... | ... | ... | ... | ... | ... | ... | ... | ... | ... | ... | ... | ... | ... | ... | ... | ... | ... | ... | ... | ... | ... | ... | ... | ... | ... | ... | ... | ... | ... | ... | ... | ... | ... | ... | ... | ... | ... | ... | ... | ... | ... | ... | ... | ... | ... | ... | ... | ... | ... | ... |
... | ... | ... | ... | ... | ... | ... | ... | ... | ... | ... | ... | ... | ... | ... | ... | ... | ... | ... | ... | ... | ... | ... | ... | ... | ... | ... | ... | ... | ... | ... | ... | ... | ... | ... | ... | ... | ... | ... | ... | ... | ... | ... | ... | ... | ... | ... | ... | ... | ... | ... | ... | ... | ... | ... | ... | ... | ... | |
... | ... | ... | ... | ... | ... | ... | ... | ... | ... | ... | ... | ... | ... | ... | ... | ... | ... | ... | ... | ... | ... | ... | ... | ... | ... | ... | ... | ... | ... | ... | ... | ... | ... | ... | ... | ... | ... | ... | ... | ... | ... | ... | ... | ... | ... | ... | ... | ... | ... | ... | ... | ... | ... | ... | ... | ... | ... |
fn_conv = dask.dataframe.read_parquet("../../urbangrammar_samba/spatial_signatures/functional/functional/*").drop(columns=["supermarkets_nearest","supermarkets_counts","listed_nearest", "listed_counts", "fhrs_nearest", "fhrs_counts", "culture_nearest", "culture_counts", "nearest_water","nearest_retail_centre", "keep_q1", "keep_q2", "keep_q3"]).reset_index(drop=True)
fn_conv
population_q1 | population_q2 | population_q3 | night_lights_q1 | night_lights_q2 | night_lights_q3 | A, B, D, E. Agriculture, energy and water_q1 | A, B, D, E. Agriculture, energy and water_q2 | A, B, D, E. Agriculture, energy and water_q3 | C. Manufacturing_q1 | C. Manufacturing_q2 | C. Manufacturing_q3 | F. Construction_q1 | F. Construction_q2 | F. Construction_q3 | G, I. Distribution, hotels and restaurants_q1 | G, I. Distribution, hotels and restaurants_q2 | G, I. Distribution, hotels and restaurants_q3 | H, J. Transport and communication_q1 | H, J. Transport and communication_q2 | H, J. Transport and communication_q3 | K, L, M, N. Financial, real estate, professional and administrative activities_q1 | K, L, M, N. Financial, real estate, professional and administrative activities_q2 | K, L, M, N. Financial, real estate, professional and administrative activities_q3 | O,P,Q. Public administration, education and health_q1 | O,P,Q. Public administration, education and health_q2 | O,P,Q. Public administration, education and health_q3 | R, S, T, U. Other_q1 | R, S, T, U. Other_q2 | R, S, T, U. Other_q3 | Code_18_124_q1 | Code_18_124_q2 | Code_18_124_q3 | Code_18_211_q1 | Code_18_211_q2 | Code_18_211_q3 | Code_18_121_q1 | Code_18_121_q2 | Code_18_121_q3 | Code_18_421_q1 | Code_18_421_q2 | Code_18_421_q3 | Code_18_522_q1 | Code_18_522_q2 | Code_18_522_q3 | Code_18_142_q1 | Code_18_142_q2 | Code_18_142_q3 | Code_18_141_q1 | Code_18_141_q2 | Code_18_141_q3 | Code_18_112_q1 | Code_18_112_q2 | Code_18_112_q3 | Code_18_231_q1 | Code_18_231_q2 | Code_18_231_q3 | Code_18_311_q1 | Code_18_311_q2 | Code_18_311_q3 | Code_18_131_q1 | Code_18_131_q2 | Code_18_131_q3 | Code_18_123_q1 | Code_18_123_q2 | Code_18_123_q3 | Code_18_122_q1 | Code_18_122_q2 | Code_18_122_q3 | Code_18_512_q1 | Code_18_512_q2 | Code_18_512_q3 | Code_18_243_q1 | Code_18_243_q2 | Code_18_243_q3 | Code_18_313_q1 | Code_18_313_q2 | Code_18_313_q3 | Code_18_412_q1 | Code_18_412_q2 | Code_18_412_q3 | Code_18_321_q1 | Code_18_321_q2 | Code_18_321_q3 | Code_18_322_q1 | Code_18_322_q2 | Code_18_322_q3 | Code_18_324_q1 | Code_18_324_q2 | Code_18_324_q3 | Code_18_111_q1 | Code_18_111_q2 | Code_18_111_q3 | Code_18_423_q1 | Code_18_423_q2 | Code_18_423_q3 | Code_18_523_q1 | Code_18_523_q2 | Code_18_523_q3 | mean_q1 | mean_q2 | mean_q3 | Code_18_312_q1 | Code_18_312_q2 | Code_18_312_q3 | Code_18_133_q1 | Code_18_133_q2 | Code_18_133_q3 | Code_18_333_q1 | Code_18_333_q2 | Code_18_333_q3 | Code_18_332_q1 | Code_18_332_q2 | Code_18_332_q3 | Code_18_411_q1 | Code_18_411_q2 | Code_18_411_q3 | Code_18_132_q1 | Code_18_331_q2 | Code_18_222_q1 | Code_18_511_q3 | Code_18_242_q1 | Code_18_511_q2 | Code_18_242_q3 | Code_18_331_q1 | Code_18_334_q2 | Code_18_511_q1 | Code_18_334_q1 | Code_18_222_q3 | Code_18_242_q2 | Code_18_244_q3 | Code_18_521_q2 | Code_18_334_q3 | Code_18_244_q1 | Code_18_244_q2 | Code_18_331_q3 | Code_18_132_q2 | Code_18_132_q3 | Code_18_521_q1 | Code_18_222_q2 | Code_18_521_q3 | |
---|---|---|---|---|---|---|---|---|---|---|---|---|---|---|---|---|---|---|---|---|---|---|---|---|---|---|---|---|---|---|---|---|---|---|---|---|---|---|---|---|---|---|---|---|---|---|---|---|---|---|---|---|---|---|---|---|---|---|---|---|---|---|---|---|---|---|---|---|---|---|---|---|---|---|---|---|---|---|---|---|---|---|---|---|---|---|---|---|---|---|---|---|---|---|---|---|---|---|---|---|---|---|---|---|---|---|---|---|---|---|---|---|---|---|---|---|---|---|---|---|---|---|---|---|---|---|---|---|---|---|---|---|---|---|---|---|---|---|---|---|---|
npartitions=103 | |||||||||||||||||||||||||||||||||||||||||||||||||||||||||||||||||||||||||||||||||||||||||||||||||||||||||||||||||||||||||||||||||||||||||||||
float64 | float64 | float64 | float64 | float64 | float64 | float64 | float64 | float64 | float64 | float64 | float64 | float64 | float64 | float64 | float64 | float64 | float64 | float64 | float64 | float64 | float64 | float64 | float64 | float64 | float64 | float64 | float64 | float64 | float64 | float64 | float64 | float64 | float64 | float64 | float64 | float64 | float64 | float64 | float64 | float64 | float64 | float64 | float64 | float64 | float64 | float64 | float64 | float64 | float64 | float64 | float64 | float64 | float64 | float64 | float64 | float64 | float64 | float64 | float64 | float64 | float64 | float64 | float64 | float64 | float64 | float64 | float64 | float64 | float64 | float64 | float64 | float64 | float64 | float64 | float64 | float64 | float64 | float64 | float64 | float64 | float64 | float64 | float64 | float64 | float64 | float64 | float64 | float64 | float64 | float64 | float64 | float64 | float64 | float64 | float64 | float64 | float64 | float64 | float64 | float64 | float64 | float64 | float64 | float64 | float64 | float64 | float64 | float64 | float64 | float64 | float64 | float64 | float64 | float64 | float64 | float64 | int64 | int64 | int64 | int64 | int64 | int64 | int64 | int64 | int64 | int64 | int64 | int64 | int64 | int64 | int64 | int64 | int64 | int64 | int64 | int64 | int64 | int64 | int64 | int64 | |
... | ... | ... | ... | ... | ... | ... | ... | ... | ... | ... | ... | ... | ... | ... | ... | ... | ... | ... | ... | ... | ... | ... | ... | ... | ... | ... | ... | ... | ... | ... | ... | ... | ... | ... | ... | ... | ... | ... | ... | ... | ... | ... | ... | ... | ... | ... | ... | ... | ... | ... | ... | ... | ... | ... | ... | ... | ... | ... | ... | ... | ... | ... | ... | ... | ... | ... | ... | ... | ... | ... | ... | ... | ... | ... | ... | ... | ... | ... | ... | ... | ... | ... | ... | ... | ... | ... | ... | ... | ... | ... | ... | ... | ... | ... | ... | ... | ... | ... | ... | ... | ... | ... | ... | ... | ... | ... | ... | ... | ... | ... | ... | ... | ... | ... | ... | ... | ... | ... | ... | ... | ... | ... | ... | ... | ... | ... | ... | ... | ... | ... | ... | ... | ... | ... | ... | ... | ... | ... | ... | ... | |
... | ... | ... | ... | ... | ... | ... | ... | ... | ... | ... | ... | ... | ... | ... | ... | ... | ... | ... | ... | ... | ... | ... | ... | ... | ... | ... | ... | ... | ... | ... | ... | ... | ... | ... | ... | ... | ... | ... | ... | ... | ... | ... | ... | ... | ... | ... | ... | ... | ... | ... | ... | ... | ... | ... | ... | ... | ... | ... | ... | ... | ... | ... | ... | ... | ... | ... | ... | ... | ... | ... | ... | ... | ... | ... | ... | ... | ... | ... | ... | ... | ... | ... | ... | ... | ... | ... | ... | ... | ... | ... | ... | ... | ... | ... | ... | ... | ... | ... | ... | ... | ... | ... | ... | ... | ... | ... | ... | ... | ... | ... | ... | ... | ... | ... | ... | ... | ... | ... | ... | ... | ... | ... | ... | ... | ... | ... | ... | ... | ... | ... | ... | ... | ... | ... | ... | ... | ... | ... | ... | ... | ... |
... | ... | ... | ... | ... | ... | ... | ... | ... | ... | ... | ... | ... | ... | ... | ... | ... | ... | ... | ... | ... | ... | ... | ... | ... | ... | ... | ... | ... | ... | ... | ... | ... | ... | ... | ... | ... | ... | ... | ... | ... | ... | ... | ... | ... | ... | ... | ... | ... | ... | ... | ... | ... | ... | ... | ... | ... | ... | ... | ... | ... | ... | ... | ... | ... | ... | ... | ... | ... | ... | ... | ... | ... | ... | ... | ... | ... | ... | ... | ... | ... | ... | ... | ... | ... | ... | ... | ... | ... | ... | ... | ... | ... | ... | ... | ... | ... | ... | ... | ... | ... | ... | ... | ... | ... | ... | ... | ... | ... | ... | ... | ... | ... | ... | ... | ... | ... | ... | ... | ... | ... | ... | ... | ... | ... | ... | ... | ... | ... | ... | ... | ... | ... | ... | ... | ... | ... | ... | ... | ... | ... | |
... | ... | ... | ... | ... | ... | ... | ... | ... | ... | ... | ... | ... | ... | ... | ... | ... | ... | ... | ... | ... | ... | ... | ... | ... | ... | ... | ... | ... | ... | ... | ... | ... | ... | ... | ... | ... | ... | ... | ... | ... | ... | ... | ... | ... | ... | ... | ... | ... | ... | ... | ... | ... | ... | ... | ... | ... | ... | ... | ... | ... | ... | ... | ... | ... | ... | ... | ... | ... | ... | ... | ... | ... | ... | ... | ... | ... | ... | ... | ... | ... | ... | ... | ... | ... | ... | ... | ... | ... | ... | ... | ... | ... | ... | ... | ... | ... | ... | ... | ... | ... | ... | ... | ... | ... | ... | ... | ... | ... | ... | ... | ... | ... | ... | ... | ... | ... | ... | ... | ... | ... | ... | ... | ... | ... | ... | ... | ... | ... | ... | ... | ... | ... | ... | ... | ... | ... | ... | ... | ... | ... |
fn = dask.dataframe.multi.concat([function, fn_conv], axis=1).rename(columns=renamer)
/opt/conda/lib/python3.9/site-packages/dask/dataframe/multi.py:1222: UserWarning: Concatenating dataframes with unknown divisions.
We're assuming that the indices of each dataframes are
aligned. This assumption is not generally safe.
warnings.warn(
import pyarrow as pa
schema = {k: pa.float64() for k in fn.columns}
schema["hindex"] = pa.string()
fn.repartition(npartitions=1000).to_parquet("../../signatures_gb/function", schema=schema)
[None]
client.restart()
Client
Client-5d4fe926-94ba-11ec-827f-6774297aa622
Connection method: Cluster object | Cluster type: distributed.LocalCluster |
Dashboard: http://127.0.0.1:8787/status |
Cluster Info
LocalCluster
25452591
Dashboard: http://127.0.0.1:8787/status | Workers: 8 |
Total threads: 16 | Total memory: 125.54 GiB |
Status: running | Using processes: True |
Scheduler Info
Scheduler
Scheduler-b7325f1b-c6cd-4c23-9a2c-b642314528a7
Comm: tcp://127.0.0.1:39497 | Workers: 8 |
Dashboard: http://127.0.0.1:8787/status | Total threads: 16 |
Started: 26 minutes ago | Total memory: 125.54 GiB |
Workers
Worker: 0
Comm: tcp://127.0.0.1:38377 | Total threads: 2 |
Dashboard: http://127.0.0.1:40007/status | Memory: 15.69 GiB |
Nanny: tcp://127.0.0.1:44439 | |
Local directory: /home/jovyan/work/spatial_signatures/data_product/dask-worker-space/worker-eowefyf5 |
Worker: 1
Comm: tcp://127.0.0.1:44561 | Total threads: 2 |
Dashboard: http://127.0.0.1:45835/status | Memory: 15.69 GiB |
Nanny: tcp://127.0.0.1:46445 | |
Local directory: /home/jovyan/work/spatial_signatures/data_product/dask-worker-space/worker-wcnccrg_ |
Worker: 2
Comm: tcp://127.0.0.1:44141 | Total threads: 2 |
Dashboard: http://127.0.0.1:45401/status | Memory: 15.69 GiB |
Nanny: tcp://127.0.0.1:42789 | |
Local directory: /home/jovyan/work/spatial_signatures/data_product/dask-worker-space/worker-d_b1cznu |
Worker: 3
Comm: tcp://127.0.0.1:46367 | Total threads: 2 |
Dashboard: http://127.0.0.1:38675/status | Memory: 15.69 GiB |
Nanny: tcp://127.0.0.1:33619 | |
Local directory: /home/jovyan/work/spatial_signatures/data_product/dask-worker-space/worker-ix070j9r |
Worker: 4
Comm: tcp://127.0.0.1:45547 | Total threads: 2 |
Dashboard: http://127.0.0.1:40375/status | Memory: 15.69 GiB |
Nanny: tcp://127.0.0.1:39231 | |
Local directory: /home/jovyan/work/spatial_signatures/data_product/dask-worker-space/worker-ltgjdbus |
Worker: 5
Comm: tcp://127.0.0.1:39895 | Total threads: 2 |
Dashboard: http://127.0.0.1:46737/status | Memory: 15.69 GiB |
Nanny: tcp://127.0.0.1:43801 | |
Local directory: /home/jovyan/work/spatial_signatures/data_product/dask-worker-space/worker-9s0njlc1 |
Worker: 6
Comm: tcp://127.0.0.1:37101 | Total threads: 2 |
Dashboard: http://127.0.0.1:33435/status | Memory: 15.69 GiB |
Nanny: tcp://127.0.0.1:38807 | |
Local directory: /home/jovyan/work/spatial_signatures/data_product/dask-worker-space/worker-r6jib2lo |
Worker: 7
Comm: tcp://127.0.0.1:36381 | Total threads: 2 |
Dashboard: http://127.0.0.1:36777/status | Memory: 15.69 GiB |
Nanny: tcp://127.0.0.1:35769 | |
Local directory: /home/jovyan/work/spatial_signatures/data_product/dask-worker-space/worker-dxhuphng |
joined = dask.dataframe.read_parquet("../../urbangrammar_samba/spatial_signatures/signatures/hindex_to_type", columns=["hindex", "type"]).rename(columns={"type": "signature_type"})
joined.repartition(npartitions=1000).to_parquet("../../signatures_gb/signature_type")
[None]
Tables of characters¶
Create tables of characters to be used in the paper.
morph = pandas.read_csv("morphometric_chars.csv")
morph.head()
context | category | reference | id | |
---|---|---|---|---|
0 | building | dimension | [@hallowell2013] | sdbAre |
1 | building | dimension | [@vanderhaegen2017] | sdbPer |
2 | building | dimension | [@schirmer2015] | sdbCoA |
3 | building | shape | [@dibble2017] | ssbCCo |
4 | building | shape | [@steiniger2008] | ssbCor |
morph["reference"] = morph["reference"].fillna("[@fleischmann2021]").apply(lambda x: x[2:-1])
morph["reference"] = "\\cite{" + morph["reference"] + "}"
morph["character"] = morph["id"].map(renamer)
morph = morph[["character", "category", "reference"]]
print(morph.to_latex(escape=False, index=False, longtable=True))
\begin{longtable}{lll}
\toprule
character & category & reference \\
\midrule
\endfirsthead
\toprule
character & category & reference \\
\midrule
\endhead
\midrule
\multicolumn{3}{r}{{Continued on next page}} \\
\midrule
\endfoot
\bottomrule
\endlastfoot
area of building & dimension & \cite{hallowell2013} \\
perimeter of building & dimension & \cite{vanderhaegen2017} \\
courtyard area of building & dimension & \cite{schirmer2015} \\
circular compactness of building & shape & \cite{dibble2017} \\
corners of building & shape & \cite{steiniger2008} \\
squareness of building & shape & \cite{steiniger2008} \\
equivalent rectangular index of building & shape & \cite{basaraner2017} \\
elongation of building & shape & \cite{steiniger2008} \\
centroid - corner distance deviation of building & shape & \cite{fleischmann2021} \\
centroid - corner mean distance of building & dimension & \cite{schirmer2015} \\
orientation of building & distribution & \cite{schirmer2015} \\
street alignment of building & distribution & \cite{schirmer2015} \\
cell alignment of building & distribution & \cite{fleischmann2021} \\
longest axis length of ETC & dimension & \cite{fleischmann2021} \\
area of ETC & dimension & \cite{hamaina2012a} \\
circular compactness of ETC & shape & \cite{fleischmann2021} \\
equivalent rectangular index of ETC & shape & \cite{fleischmann2021} \\
orientation of ETC & distribution & \cite{fleischmann2021} \\
covered area ratio of ETC & intensity & \cite{hamaina2013} \\
length of street segment & dimension & \cite{gil2012} \\
width of street profile & dimension & \cite{araldi2019} \\
openness of street profile & distribution & \cite{araldi2019} \\
width deviation of street profile & diversity & \cite{araldi2019} \\
linearity of street segment & shape & \cite{araldi2019} \\
area covered by edge-attached ETCs & dimension & \cite{fleischmann2021} \\
buildings per meter of street segment & intensity & \cite{fleischmann2021} \\
area covered by node-attached ETCs & dimension & \cite{fleischmann2021} \\
alignment of neighbouring buildings & distribution & \cite{hijazi2016} \\
mean distance between neighbouring buildings & distribution & \cite{hijazi2016} \\
perimeter-weighted neighbours of ETC & distribution & \cite{fleischmann2021} \\
area covered by neighbouring cells & dimension & \cite{fleischmann2021} \\
reached ETCs by neighbouring segments & intensity & \cite{fleischmann2021} \\
reached area by neighbouring segments & dimension & \cite{fleischmann2021} \\
node degree of junction & distribution & \cite{boeing2018} \\
mean distance to neighbouring nodes of street n... & dimension & \cite{fleischmann2021} \\
mean inter-building distance & distribution & \cite{caruso2017} \\
weighted reached enclosures of ETC & intensity & \cite{fleischmann2021} \\
reached ETCs by tessellation contiguity & intensity & \cite{fleischmann2021} \\
reached area by tessellation contiguity & dimension & \cite{fleischmann2021} \\
area of enclosure & dimension & \cite{dibble2017} \\
perimeter of enclosure & dimension & \cite{gil2012} \\
circular compactness of enclosure & shape & \cite{schirmer2015} \\
equivalent rectangular index of enclosure & shape & \cite{basaraner2017} \\
compactness-weighted axis of enclosure & shape & \cite{feliciotti2018} \\
orientation of enclosure & distribution & \cite{gil2012} \\
perimeter-weighted neighbours of enclosure & distribution & \cite{fleischmann2021} \\
area-weighted ETCs of enclosure & intensity & \cite{fleischmann2021} \\
local meshedness of street network & connectivity & \cite{feliciotti2018} \\
mean segment length within 3 steps & dimension & \cite{fleischmann2021} \\
local cul-de-sac length of street network & dimension & \cite{fleischmann2021} \\
reached area by local street network & dimension & \cite{fleischmann2021} \\
reached ETCs by local street network & intensity & \cite{fleischmann2021} \\
local node density of street network & intensity & \cite{fleischmann2021} \\
local proportion of cul-de-sacs of street network & connectivity & \cite{lowry2014} \\
local proportion of 3-way intersections of stre... & connectivity & \cite{boeing2018} \\
local proportion of 4-way intersections of stre... & connectivity & \cite{boeing2018} \\
local degree weighted node density of street ne... & intensity & \cite{dibble2017} \\
local closeness of street network & connectivity & \cite{porta2006} \\
square clustering of street network & connectivity & \cite{fleischmann2021} \\
\end{longtable}
func = pandas.Series({'population': 'Population',
'night_lights': 'Night lights',
'A, B, D, E. Agriculture, energy and water': 'Workplace population [Agriculture, energy and water]',
'C. Manufacturing': 'Workplace population [Manufacturing]',
'F. Construction': 'Workplace population [Construction]',
'G, I. Distribution, hotels and restaurants': 'Workplace population [Distribution, hotels and restaurants]',
'H, J. Transport and communication': 'Workplace population [Transport and communication]',
'K, L, M, N. Financial, real estate, professional and administrative activities': 'Workplace population [Financial, real estate, professional and administrative activities]',
'O,P,Q. Public administration, education and health': 'Workplace population [Public administration, education and health]',
'R, S, T, U. Other': 'Workplace population [Other]',
'Code_18_124': 'Land cover [Airports]',
'Code_18_211': 'Land cover [Non-irrigated arable land]',
'Code_18_121': 'Land cover [Industrial or commercial units]',
'Code_18_421': 'Land cover [Salt marshes]',
'Code_18_522': 'Land cover [Estuaries]',
'Code_18_142': 'Land cover [Sport and leisure facilities]',
'Code_18_141': 'Land cover [Green urban areas]',
'Code_18_112': 'Land cover [Discontinuous urban fabric]',
'Code_18_231': 'Land cover [Pastures]',
'Code_18_311': 'Land cover [Broad-leaved forest]',
'Code_18_131': 'Land cover [Mineral extraction sites]',
'Code_18_123': 'Land cover [Port areas]',
'Code_18_122': 'Land cover [Road and rail networks and associated land]',
'Code_18_512': 'Land cover [Water bodies]',
'Code_18_243': 'Land cover [Land principally occupied by agriculture, with significant areas of natural vegetation]',
'Code_18_313': 'Land cover [Mixed forest]',
'Code_18_412': 'Land cover [Peat bogs]',
'Code_18_321': 'Land cover [Natural grasslands]',
'Code_18_322': 'Land cover [Moors and heathland]',
'Code_18_324': 'Land cover [Transitional woodland-shrub]',
'Code_18_111': 'Land cover [Continuous urban fabric]',
'Code_18_423': 'Land cover [Intertidal flats]',
'Code_18_523': 'Land cover [Sea and ocean]',
'Code_18_312': 'Land cover [Coniferous forest]',
'Code_18_133': 'Land cover [Construction sites]',
'Code_18_333': 'Land cover [Sparsely vegetated areas]',
'Code_18_332': 'Land cover [Bare rocks]',
'Code_18_411': 'Land cover [Inland marshes]',
'Code_18_132': 'Land cover [Dump sites]',
'Code_18_222': 'Land cover [Fruit trees and berry plantations]',
'Code_18_242': 'Land cover [Complex cultivation patterns]',
'Code_18_331': 'Land cover [Beaches, dunes, sands]',
'Code_18_511': 'Land cover [Water courses]',
'Code_18_334': 'Land cover [Burnt areas]',
'Code_18_244': 'Land cover [Agro-forestry areas]',
'Code_18_521': 'Land cover [Coastal lagoons]',
'mean': 'NDVI',
'supermarkets_nearest': 'Supermarkets [distance to nearest]',
'supermarkets_counts': 'Supermarkets [counts within 1200m]',
'listed_nearest': 'Listed buildings [distance to nearest]',
'listed_counts': 'Listed buildings [counts within 1200m]',
'fhrs_nearest': 'FHRS points [distance to nearest]',
'fhrs_counts': 'FHRS points [counts within 1200m]',
'culture_nearest': 'Cultural venues [distance to nearest]',
'culture_counts': 'Cultural venues [counts within 1200m]',
'nearest_water': 'Water bodies [distance to nearest]',
'nearest_retail_centre': 'Retail centres [distance to nearest]',}, name="character").reset_index(drop=True)
func = func.to_frame()
or_func = pandas.read_csv("func.csv", delimiter=";")
or_func = or_func.drop(columns="Unnamed: 4")
rows = [0, 4, 6, 6, 6, 6, 6, 6, 6] + ([8] * 37) + [9, 1, 1, 3, 3, 5, 5, 7, 7, 2, 10]
or_func.iloc[rows].shape
(57, 4)
functional = pandas.concat([func, or_func.iloc[rows].reset_index(drop=True)], axis=1)
with pandas.option_context("max_colwidth", 1000):
print(functional.to_latex(index=False, longtable=True))
\begin{longtable}{lllll}
\toprule
character & data & source & input geometry & transfer method \\
\midrule
\endfirsthead
\toprule
character & data & source & input geometry & transfer method \\
\midrule
\endhead
\midrule
\multicolumn{5}{r}{{Continued on next page}} \\
\midrule
\endfoot
\bottomrule
\endlastfoot
Population & Population estimates & ONS Census Output Area population estimates, Statistics.gov.scot & Vector (output area polygon) & Building-based dasymetric areal interpolation \\
Night lights & Night Lights & VIIRS DNB Nighttime Lights & Raster (500m) & Zonal statistics \\
Workplace population [Agriculture, energy and water] & Workplace population & ONS Census Workplace population, Scotland's census Workplace population & Vector (output area polygon) & Building-based dasymetric areal interpolation \\
Workplace population [Manufacturing] & Workplace population & ONS Census Workplace population, Scotland's census Workplace population & Vector (output area polygon) & Building-based dasymetric areal interpolation \\
Workplace population [Construction] & Workplace population & ONS Census Workplace population, Scotland's census Workplace population & Vector (output area polygon) & Building-based dasymetric areal interpolation \\
Workplace population [Distribution, hotels and restaurants] & Workplace population & ONS Census Workplace population, Scotland's census Workplace population & Vector (output area polygon) & Building-based dasymetric areal interpolation \\
Workplace population [Transport and communication] & Workplace population & ONS Census Workplace population, Scotland's census Workplace population & Vector (output area polygon) & Building-based dasymetric areal interpolation \\
Workplace population [Financial, real estate, professional and administrative activities] & Workplace population & ONS Census Workplace population, Scotland's census Workplace population & Vector (output area polygon) & Building-based dasymetric areal interpolation \\
Workplace population [Public administration, education and health] & Workplace population & ONS Census Workplace population, Scotland's census Workplace population & Vector (output area polygon) & Building-based dasymetric areal interpolation \\
Workplace population [Other] & Corine land cover & Copernicus Land Monitoring Service & Vector (land cover zone polygon) & Areal interpolation \\
Land cover [Airports] & Corine land cover & Copernicus Land Monitoring Service & Vector (land cover zone polygon) & Areal interpolation \\
Land cover [Non-irrigated arable land] & Corine land cover & Copernicus Land Monitoring Service & Vector (land cover zone polygon) & Areal interpolation \\
Land cover [Industrial or commercial units] & Corine land cover & Copernicus Land Monitoring Service & Vector (land cover zone polygon) & Areal interpolation \\
Land cover [Salt marshes] & Corine land cover & Copernicus Land Monitoring Service & Vector (land cover zone polygon) & Areal interpolation \\
Land cover [Estuaries] & Corine land cover & Copernicus Land Monitoring Service & Vector (land cover zone polygon) & Areal interpolation \\
Land cover [Sport and leisure facilities] & Corine land cover & Copernicus Land Monitoring Service & Vector (land cover zone polygon) & Areal interpolation \\
Land cover [Green urban areas] & Corine land cover & Copernicus Land Monitoring Service & Vector (land cover zone polygon) & Areal interpolation \\
Land cover [Discontinuous urban fabric] & Corine land cover & Copernicus Land Monitoring Service & Vector (land cover zone polygon) & Areal interpolation \\
Land cover [Pastures] & Corine land cover & Copernicus Land Monitoring Service & Vector (land cover zone polygon) & Areal interpolation \\
Land cover [Broad-leaved forest] & Corine land cover & Copernicus Land Monitoring Service & Vector (land cover zone polygon) & Areal interpolation \\
Land cover [Mineral extraction sites] & Corine land cover & Copernicus Land Monitoring Service & Vector (land cover zone polygon) & Areal interpolation \\
Land cover [Port areas] & Corine land cover & Copernicus Land Monitoring Service & Vector (land cover zone polygon) & Areal interpolation \\
Land cover [Road and rail networks and associated land] & Corine land cover & Copernicus Land Monitoring Service & Vector (land cover zone polygon) & Areal interpolation \\
Land cover [Water bodies] & Corine land cover & Copernicus Land Monitoring Service & Vector (land cover zone polygon) & Areal interpolation \\
Land cover [Land principally occupied by agriculture, with significant areas of natural vegetation] & Corine land cover & Copernicus Land Monitoring Service & Vector (land cover zone polygon) & Areal interpolation \\
Land cover [Mixed forest] & Corine land cover & Copernicus Land Monitoring Service & Vector (land cover zone polygon) & Areal interpolation \\
Land cover [Peat bogs] & Corine land cover & Copernicus Land Monitoring Service & Vector (land cover zone polygon) & Areal interpolation \\
Land cover [Natural grasslands] & Corine land cover & Copernicus Land Monitoring Service & Vector (land cover zone polygon) & Areal interpolation \\
Land cover [Moors and heathland] & Corine land cover & Copernicus Land Monitoring Service & Vector (land cover zone polygon) & Areal interpolation \\
Land cover [Transitional woodland-shrub] & Corine land cover & Copernicus Land Monitoring Service & Vector (land cover zone polygon) & Areal interpolation \\
Land cover [Continuous urban fabric] & Corine land cover & Copernicus Land Monitoring Service & Vector (land cover zone polygon) & Areal interpolation \\
Land cover [Intertidal flats] & Corine land cover & Copernicus Land Monitoring Service & Vector (land cover zone polygon) & Areal interpolation \\
Land cover [Sea and ocean] & Corine land cover & Copernicus Land Monitoring Service & Vector (land cover zone polygon) & Areal interpolation \\
Land cover [Coniferous forest] & Corine land cover & Copernicus Land Monitoring Service & Vector (land cover zone polygon) & Areal interpolation \\
Land cover [Construction sites] & Corine land cover & Copernicus Land Monitoring Service & Vector (land cover zone polygon) & Areal interpolation \\
Land cover [Sparsely vegetated areas] & Corine land cover & Copernicus Land Monitoring Service & Vector (land cover zone polygon) & Areal interpolation \\
Land cover [Bare rocks] & Corine land cover & Copernicus Land Monitoring Service & Vector (land cover zone polygon) & Areal interpolation \\
Land cover [Inland marshes] & Corine land cover & Copernicus Land Monitoring Service & Vector (land cover zone polygon) & Areal interpolation \\
Land cover [Dump sites] & Corine land cover & Copernicus Land Monitoring Service & Vector (land cover zone polygon) & Areal interpolation \\
Land cover [Fruit trees and berry plantations] & Corine land cover & Copernicus Land Monitoring Service & Vector (land cover zone polygon) & Areal interpolation \\
Land cover [Complex cultivation patterns] & Corine land cover & Copernicus Land Monitoring Service & Vector (land cover zone polygon) & Areal interpolation \\
Land cover [Beaches, dunes, sands] & Corine land cover & Copernicus Land Monitoring Service & Vector (land cover zone polygon) & Areal interpolation \\
Land cover [Water courses] & Corine land cover & Copernicus Land Monitoring Service & Vector (land cover zone polygon) & Areal interpolation \\
Land cover [Burnt areas] & Corine land cover & Copernicus Land Monitoring Service & Vector (land cover zone polygon) & Areal interpolation \\
Land cover [Agro-forestry areas] & Corine land cover & Copernicus Land Monitoring Service & Vector (land cover zone polygon) & Areal interpolation \\
Land cover [Coastal lagoons] & Corine land cover & Copernicus Land Monitoring Service & Vector (land cover zone polygon) & Areal interpolation \\
NDVI & NDVI & GHS-composite-S2 R2020A & Raster (10m) & Zonal statistics \\
Supermarkets [distance to nearest] & Retail POIs (supermarkets) & Geolytix & Vector (point) & Network-constrained accessibility \\
Supermarkets [counts within 1200m] & Retail POIs (supermarkets) & Geolytix & Vector (point) & Network-constrained accessibility \\
Listed buildings [distance to nearest] & Listed Buildings & Historic England, Historic Environment Scotland, Lle Geo-Portal for Wales & Vector (point) & Network-constrained accessibility \\
Listed buildings [counts within 1200m] & Listed Buildings & Historic England, Historic Environment Scotland, Lle Geo-Portal for Wales & Vector (point) & Network-constrained accessibility \\
FHRS points [distance to nearest] & Food Hygiene Rating Scheme Ratings & CDRC.ac.uk & Vector (point) & Network-constrained accessibility \\
FHRS points [counts within 1200m] & Food Hygiene Rating Scheme Ratings & CDRC.ac.uk & Vector (point) & Network-constrained accessibility \\
Cultural venues [distance to nearest] & Culture (theatres, cinemas) & OpenStreetMap & Vector (point) & Network-constrained accessibility \\
Cultural venues [counts within 1200m] & Culture (theatres, cinemas) & OpenStreetMap & Vector (point) & Network-constrained accessibility \\
Water bodies [distance to nearest] & Water bodies & OS OpenMap Local & Vector (water body polygon) & Euclidean accessibility \\
Retail centres [distance to nearest] & Retail centres & CDRC.ac.uk & Vector (retail centre polygon) & Euclidean accessibility \\
\end{longtable}
with pandas.option_context("max_colwidth", 10000):
print(pandas.Series(
{
"Wild countryside": "In “Wild countryside”, human influence is the least intensive. This signature covers large open spaces in the countryside where no urbanisation happens apart from occasional roads, cottages, and pastures. You can find it across the Scottish Highlands, numerous national parks such as Lake District, or in the majority of Wales.",
"Countryside agriculture": "“Countryside agriculture” features much of the English countryside and displays a high degree of agriculture including both fields and pastures. There are a few buildings scattered across the area but, for the most part, it is green space.",
"Urban buffer": "“Urban buffer” can be characterised as a green belt around cities. This signature includes mostly agricultural land in the immediate adjacency of towns and cities, often including edge development. It still feels more like countryside than urban, but these signatures are much smaller compared to other countryside types.",
"Open sprawl": "“Open sprawl” represents the transition between countryside and urbanised land. It is located in the outskirts of cities or around smaller towns and is typically made up of large open space areas intertwined with different kinds of human development, from highways to smaller neighbourhoods.",
"Disconnected suburbia": "“Disconnected suburbia” includes residential developments in the outskirts of cities or even towns and villages with convoluted, disconnected street networks, low built-up and population densities, and lack of jobs and services. This signature type is entirely car-dependent.",
"Accessible suburbia": "“Accessible suburbia” covers residential development on the urban periphery with a relatively legible and connected street network, albeit less so than other more urban signature types. Areas in this signature feature low density, both in terms of population and built-up area, lack of jobs and services. For these reasons, “accessible suburbia” largely acts as dormitories.",
"Warehouse/Park land": "“Warehouse/Park land” covers predominantly industrial areas and other work-related developments made of box-like buildings with large footprints. It contains many jobs of manual nature such as manufacturing or construction, and very little population live here compared to the rest of urban areas. Occasionally this type also covers areas of parks with large scale green open areas.",
"Gridded residential quarters": "“Gridded residential quarters” are areas with street networks forming a well-connected grid-like (high density of 4-way intersections) pattern, resulting in places with smaller blocks and higher granularity. This signature is mostly residential but includes some services and jobs, and it tends to be located away from city centres.",
"Connected residential neighbourhoods": "“Connected residential neighbourhoods” are relatively dense urban areas, both in terms of population and built-up area, that tend to be formed around well-connected street networks. They have access to services and some jobs but may be further away from city centres leading to higher dependency on cars and public transport for their residents.",
"Dense residential neighbourhoods": "A “dense residential neighbourhood” is an abundant signature often covering large parts of cities outside of their centres. It has primarily residential purpose and high population density, varied street network patterns, and some services and jobs but not in high intensity.",
"Dense urban neighbourhoods": "“Dense urban neighbourhoods” are areas of inner-city with high population and built-up density of a predominantly residential nature but with direct access to jobs and services. This signature type tends to be relatively walkable and, in the case of some towns, may even form their centres.",
"Local urbanity": "“Local urbanity” reflects town centres, outer parts of city centres or even district centres. In all cases, this signature is very much urban in essence, combining high population and built-up density, access to amenities and jobs. Yet, it is on the lower end of the hierarchy of signature types denoting urban centres with only a local significance.",
"Regional urbanity": "“Regional urbanity” captures centres of mid-size cities with regional importance such as Liverpool, Plymouth or Newcastle upon Tyne. It is often encircled by “Local urbanity” signatures and can form outer rings of city centres in large cities. It features high population density, as well as a high number of jobs and amenities within walkable distance.",
"Metropolitan urbanity": "Signature type “Metropolitan urbanity” captures the centre of the largest cities in Great Britain such as Glasgow, Birmingham or Manchester. It is characterised by a very high number of jobs in the area, high built-up density and often high population density. This type serves as the core centre of the entire metropolitan areas.",
"Concentrated urbanity": "Concentrated urbanity” is a signature type found in the city centre of London and nowhere else in Great Britain. It reflects the uniqueness of London in the British context with an extremely high number of jobs and amenities located nearby, as well as high built-up and population densities. Buildings in this signature are large and tightly packed, forming complex shapes with courtyards and little green space.",
"Hyper concentrated urbanity": "The epitome of urbanity in the British context. “Hyper concentrated urbanity” is a signature type present only in the centre of London, around the Soho district, and covering Oxford and Regent streets. This signature is the result of centuries of urban primacy, with a multitude of historical layers interwoven, very high built-up and population density, and extreme abundance of amenities, services and jobs.",
}
).to_latex())
\begin{tabular}{ll}
\toprule
{} & 0 \\
\midrule
Wild countryside & In “Wild countryside”, human influence is the least intensive. This signature covers large open spaces in the countryside where no urbanisation happens apart from occasional roads, cottages, and pastures. You can find it across the Scottish Highlands, numerous national parks such as Lake District, or in the majority of Wales. \\
Countryside agriculture & “Countryside agriculture” features much of the English countryside and displays a high degree of agriculture including both fields and pastures. There are a few buildings scattered across the area but, for the most part, it is green space. \\
Urban buffer & “Urban buffer” can be characterised as a green belt around cities. This signature includes mostly agricultural land in the immediate adjacency of towns and cities, often including edge development. It still feels more like countryside than urban, but these signatures are much smaller compared to other countryside types. \\
Open sprawl & “Open sprawl” represents the transition between countryside and urbanised land. It is located in the outskirts of cities or around smaller towns and is typically made up of large open space areas intertwined with different kinds of human development, from highways to smaller neighbourhoods. \\
Disconnected suburbia & “Disconnected suburbia” includes residential developments in the outskirts of cities or even towns and villages with convoluted, disconnected street networks, low built-up and population densities, and lack of jobs and services. This signature type is entirely car-dependent. \\
Accessible suburbia & “Accessible suburbia” covers residential development on the urban periphery with a relatively legible and connected street network, albeit less so than other more urban signature types. Areas in this signature feature low density, both in terms of population and built-up area, lack of jobs and services. For these reasons, “accessible suburbia” largely acts as dormitories. \\
Warehouse/Park land & “Warehouse/Park land” covers predominantly industrial areas and other work-related developments made of box-like buildings with large footprints. It contains many jobs of manual nature such as manufacturing or construction, and very little population live here compared to the rest of urban areas. Occasionally this type also covers areas of parks with large scale green open areas. \\
Gridded residential quarters & “Gridded residential quarters” are areas with street networks forming a well-connected grid-like (high density of 4-way intersections) pattern, resulting in places with smaller blocks and higher granularity. This signature is mostly residential but includes some services and jobs, and it tends to be located away from city centres. \\
Connected residential neighbourhoods & “Connected residential neighbourhoods” are relatively dense urban areas, both in terms of population and built-up area, that tend to be formed around well-connected street networks. They have access to services and some jobs but may be further away from city centres leading to higher dependency on cars and public transport for their residents. \\
Dense residential neighbourhoods & A “dense residential neighbourhood” is an abundant signature often covering large parts of cities outside of their centres. It has primarily residential purpose and high population density, varied street network patterns, and some services and jobs but not in high intensity. \\
Dense urban neighbourhoods & “Dense urban neighbourhoods” are areas of inner-city with high population and built-up density of a predominantly residential nature but with direct access to jobs and services. This signature type tends to be relatively walkable and, in the case of some towns, may even form their centres. \\
Local urbanity & “Local urbanity” reflects town centres, outer parts of city centres or even district centres. In all cases, this signature is very much urban in essence, combining high population and built-up density, access to amenities and jobs. Yet, it is on the lower end of the hierarchy of signature types denoting urban centres with only a local significance. \\
Regional urbanity & “Regional urbanity” captures centres of mid-size cities with regional importance such as Liverpool, Plymouth or Newcastle upon Tyne. It is often encircled by “Local urbanity” signatures and can form outer rings of city centres in large cities. It features high population density, as well as a high number of jobs and amenities within walkable distance. \\
Metropolitan urbanity & Signature type “Metropolitan urbanity” captures the centre of the largest cities in Great Britain such as Glasgow, Birmingham or Manchester. It is characterised by a very high number of jobs in the area, high built-up density and often high population density. This type serves as the core centre of the entire metropolitan areas. \\
Concentrated urbanity & Concentrated urbanity” is a signature type found in the city centre of London and nowhere else in Great Britain. It reflects the uniqueness of London in the British context with an extremely high number of jobs and amenities located nearby, as well as high built-up and population densities. Buildings in this signature are large and tightly packed, forming complex shapes with courtyards and little green space. \\
Hyper concentrated urbanity & The epitome of urbanity in the British context. “Hyper concentrated urbanity” is a signature type present only in the centre of London, around the Soho district, and covering Oxford and Regent streets. This signature is the result of centuries of urban primacy, with a multitude of historical layers interwoven, very high built-up and population density, and extreme abundance of amenities, services and jobs. \\
\bottomrule
\end{tabular}
prof = pandas.read_csv("../../urbangrammar_samba/spatial_signatures/data_product/per_type.csv").drop(columns="code").set_index("type").T
prof.index = prof.index.map(key)
with pandas.option_context("max_colwidth", 10000):
print(prof.to_latex(float_format="%.2f"))
\begin{tabular}{lrrrrrrrrrrrrrrrr}
\toprule
type & Accessible suburbia & Connected residential neighbourhoods & Countryside agriculture & Dense residential neighbourhoods & Dense urban neighbourhoods & Disconnected suburbia & Concentrated urbanity & Gridded residential quarters & Hyper concentrated urbanity & Local urbanity & Metropolitan urbanity & Open sprawl & Regional urbanity & Urban buffer & Warehouse/Park land & Wild countryside \\
\midrule
area of building & 176.95 & 272.52 & 204.10 & 375.60 & 588.36 & 212.71 & 3713.38 & 283.89 & 3358.10 & 823.35 & 2413.94 & 226.72 & 1480.26 & 209.42 & 393.22 & 209.86 \\
perimeter of building & 53.90 & 69.12 & 56.05 & 80.56 & 107.36 & 61.63 & 376.30 & 69.67 & 330.82 & 135.54 & 283.94 & 59.64 & 195.98 & 55.94 & 75.68 & 57.12 \\
courtyard area of building & 0.48 & 1.07 & 0.51 & 2.13 & 5.03 & 0.52 & 159.09 & 0.75 & 90.82 & 12.67 & 118.95 & 0.90 & 43.19 & 0.74 & 3.26 & 0.22 \\
circular compactness of building & 0.53 & 0.48 & 0.51 & 0.47 & 0.44 & 0.49 & 0.43 & 0.49 & 0.45 & 0.41 & 0.40 & 0.52 & 0.39 & 0.52 & 0.47 & 0.50 \\
corners of building & 4.25 & 4.45 & 4.37 & 4.69 & 5.21 & 4.35 & 12.48 & 4.51 & 9.27 & 6.01 & 9.72 & 4.37 & 7.78 & 4.34 & 4.56 & 4.38 \\
squareness of building & 0.78 & 1.47 & 0.81 & 1.86 & 3.28 & 1.02 & 18.59 & 1.66 & 22.51 & 5.07 & 12.41 & 0.99 & 8.84 & 0.86 & 1.35 & 0.71 \\
equivalent rectangular index of building & 0.99 & 0.98 & 0.98 & 0.97 & 0.95 & 0.98 & 0.78 & 0.98 & 0.80 & 0.92 & 0.82 & 0.98 & 0.87 & 0.98 & 0.98 & 0.98 \\
elongation of building & 0.64 & 0.56 & 0.60 & 0.56 & 0.52 & 0.57 & 0.59 & 0.58 & 0.62 & 0.51 & 0.53 & 0.62 & 0.51 & 0.63 & 0.54 & 0.59 \\
centroid - corner mean distance of building & 9.60 & 12.41 & 9.79 & 13.96 & 18.00 & 11.11 & 35.93 & 12.41 & 37.22 & 20.71 & 29.68 & 10.49 & 25.25 & 9.81 & 13.20 & 9.95 \\
centroid - corner distance deviation of building & 0.36 & 0.71 & 0.56 & 1.07 & 1.88 & 0.54 & 9.03 & 0.80 & 7.70 & 2.98 & 6.78 & 0.55 & 4.98 & 0.49 & 0.88 & 0.60 \\
orientation of building & 19.56 & 25.50 & 20.57 & 16.41 & 20.64 & 26.39 & 20.32 & 23.13 & 26.26 & 20.78 & 22.30 & 20.21 & 21.82 & 21.10 & 23.30 & 21.86 \\
longest axis length of ETC & 50.84 & 57.72 & 220.30 & 64.46 & 73.56 & 53.55 & 112.12 & 52.89 & 126.58 & 80.14 & 100.52 & 60.97 & 91.91 & 105.16 & 78.67 & 449.71 \\
area of ETC & 1147.25 & 1517.81 & 31193.48 & 1917.31 & 2410.32 & 1259.03 & 5708.23 & 1251.54 & 8654.32 & 2696.40 & 4442.21 & 2000.37 & 3535.28 & 8658.83 & 3520.84 & 155623.92 \\
circular compactness of ETC & 0.47 & 0.48 & 0.38 & 0.48 & 0.47 & 0.49 & 0.46 & 0.48 & 0.47 & 0.46 & 0.42 & 0.47 & 0.44 & 0.44 & 0.46 & 0.35 \\
equivalent rectangular index of ETC & 0.97 & 0.97 & 0.93 & 0.96 & 0.96 & 0.97 & 0.94 & 0.97 & 0.95 & 0.95 & 0.93 & 0.97 & 0.94 & 0.95 & 0.96 & 0.91 \\
orientation of ETC & 20.40 & 24.94 & 21.92 & 17.77 & 21.07 & 25.28 & 20.37 & 23.06 & 25.96 & 21.22 & 22.38 & 21.07 & 21.88 & 21.86 & 23.27 & 22.51 \\
covered area ratio of ETC & 0.19 & 0.20 & 0.07 & 0.52 & 0.27 & 0.22 & 0.91 & 0.23 & 0.61 & 0.60 & 4.85 & 0.18 & 1122.51 & 0.14 & 0.18 & 0.04 \\
cell alignment of building & 7.38 & 6.12 & 11.49 & 6.52 & 5.61 & 8.08 & 4.43 & 5.48 & 2.72 & 5.64 & 4.86 & 8.64 & 5.25 & 9.76 & 8.03 & 12.55 \\
alignment of neighbouring buildings & 5.31 & 5.36 & 8.45 & 5.39 & 5.17 & 5.67 & 5.95 & 4.93 & 6.55 & 5.67 & 6.37 & 6.48 & 6.27 & 7.06 & 6.06 & 10.05 \\
mean distance between neighbouring buildings & 17.82 & 19.17 & 111.38 & 20.84 & 21.13 & 18.63 & 18.96 & 16.48 & 22.95 & 20.62 & 22.33 & 22.13 & 20.94 & 45.37 & 28.71 & 238.45 \\
perimeter-weighted neighbours of ETC & 0.04 & 0.04 & 0.02 & 0.04 & 0.07 & 0.05 & 0.03 & 0.04 & 0.04 & 0.11 & 0.04 & 0.06 & 7.46 & 0.13 & 0.04 & 0.01 \\
area covered by neighbouring cells & 8620.11 & 11990.46 & 277883.95 & 15619.36 & 20375.37 & 9503.57 & 52023.10 & 9962.17 & 61122.40 & 22892.04 & 39665.51 & 16780.98 & 31594.99 & 76942.43 & 31956.96 & 1485709.28 \\
weighted reached enclosures of ETC & 0.00 & 0.00 & 0.00 & 0.00 & 0.00 & 0.00 & 0.00 & 0.00 & 0.00 & 0.00 & 0.00 & 0.00 & 0.00 & 0.00 & 0.00 & 0.00 \\
mean inter-building distance & 21.97 & 24.07 & 167.60 & 26.48 & 27.37 & 22.03 & 22.73 & 21.34 & 23.74 & 26.28 & 26.99 & 28.94 & 26.32 & 67.27 & 40.97 & 367.72 \\
width of street profile & 28.38 & 26.84 & 32.84 & 26.29 & 24.84 & 27.65 & 19.47 & 24.27 & 17.47 & 24.56 & 22.61 & 28.59 & 23.44 & 31.00 & 30.85 & 34.31 \\
width deviation of street profile & 3.30 & 3.27 & 3.91 & 3.50 & 3.45 & 3.71 & 3.29 & 3.87 & 2.85 & 3.60 & 3.50 & 3.74 & 3.62 & 3.76 & 3.26 & 3.41 \\
openness of street profile & 0.42 & 0.41 & 0.83 & 0.43 & 0.41 & 0.44 & 0.28 & 0.38 & 0.22 & 0.41 & 0.37 & 0.48 & 0.39 & 0.62 & 0.53 & 0.92 \\
length of street segment & 187.61 & 162.45 & 574.25 & 153.66 & 151.58 & 150.53 & 108.90 & 126.02 & 93.90 & 143.14 & 123.30 & 183.43 & 132.18 & 333.77 & 220.94 & 842.79 \\
linearity of street segment & 0.93 & 0.94 & 0.93 & 0.92 & 0.93 & 0.92 & 0.94 & 0.94 & 0.97 & 0.92 & 0.93 & 0.90 & 0.92 & 0.91 & 0.91 & 0.91 \\
mean segment length within 3 steps & 2327.31 & 2374.39 & 5884.25 & 1992.44 & 2113.58 & 1707.52 & 1944.94 & 1950.07 & 2057.70 & 2011.42 & 2112.12 & 1862.02 & 2034.72 & 3170.78 & 2339.74 & 8062.03 \\
node degree of junction & 2.87 & 3.00 & 2.78 & 2.89 & 2.94 & 2.68 & 3.12 & 3.04 & 3.33 & 2.94 & 3.14 & 2.68 & 3.01 & 2.70 & 2.77 & 2.69 \\
local meshedness of street network & 0.08 & 0.11 & 0.06 & 0.10 & 0.11 & 0.05 & 0.14 & 0.13 & 0.17 & 0.11 & 0.14 & 0.06 & 0.12 & 0.05 & 0.08 & 0.05 \\
local proportion of 3-way intersections of street network & 0.74 & 0.74 & 0.72 & 0.74 & 0.74 & 0.71 & 0.76 & 0.72 & 0.70 & 0.75 & 0.75 & 0.71 & 0.76 & 0.71 & 0.75 & 0.68 \\
local proportion of 4-way intersections of street network & 0.07 & 0.12 & 0.04 & 0.09 & 0.11 & 0.04 & 0.15 & 0.16 & 0.23 & 0.11 & 0.17 & 0.04 & 0.13 & 0.04 & 0.05 & 0.04 \\
local proportion of cul-de-sacs of street network & 0.19 & 0.14 & 0.24 & 0.17 & 0.14 & 0.25 & 0.09 & 0.12 & 0.06 & 0.14 & 0.08 & 0.25 & 0.11 & 0.25 & 0.20 & 0.28 \\
local closeness of street network & 0.00 & 0.00 & 0.00 & 0.00 & 0.00 & 0.00 & 0.00 & 0.00 & 0.00 & 0.00 & 0.00 & 0.00 & 0.00 & 0.00 & 0.00 & 0.00 \\
local cul-de-sac length of street network & 228.58 & 163.78 & 636.07 & 196.63 & 170.89 & 275.96 & 84.41 & 133.13 & 75.11 & 167.72 & 79.56 & 288.26 & 128.76 & 408.67 & 253.49 & 1186.52 \\
square clustering of street network & 0.03 & 0.04 & 0.01 & 0.03 & 0.04 & 0.01 & 0.03 & 0.04 & 0.04 & 0.03 & 0.04 & 0.02 & 0.03 & 0.02 & 0.03 & 0.01 \\
mean distance to neighbouring nodes of street network & 132.49 & 118.06 & 373.74 & 112.48 & 111.55 & 111.69 & 86.38 & 92.19 & 81.24 & 106.90 & 93.66 & 129.03 & 99.79 & 212.34 & 150.43 & 601.60 \\
local node density of street network & 0.02 & 0.02 & 0.01 & 0.02 & 0.02 & 0.03 & 0.02 & 0.02 & 0.02 & 0.02 & 0.02 & 0.03 & 0.02 & 0.02 & 0.02 & 0.01 \\
local degree weighted node density of street network & 0.03 & 0.03 & 0.02 & 0.04 & 0.04 & 0.04 & 0.04 & 0.04 & 0.04 & 0.04 & 0.04 & 0.04 & 0.04 & 0.03 & 0.03 & 0.01 \\
street alignment of building & 8.73 & 7.53 & 11.81 & 8.25 & 7.57 & 9.98 & 7.84 & 6.95 & 6.23 & 8.05 & 8.32 & 10.97 & 8.06 & 11.33 & 10.02 & 12.77 \\
area covered by node-attached ETCs & 22426.36 & 14599.22 & 286081.33 & 14037.94 & 13513.86 & 15656.96 & 13069.71 & 9488.11 & 20051.57 & 11878.66 & 13201.87 & 25443.99 & 12080.93 & 100470.30 & 33097.00 & 1215083.95 \\
area covered by edge-attached ETCs & 36496.96 & 24423.69 & 502883.47 & 25413.44 & 26111.44 & 26810.65 & 33257.27 & 17094.77 & 38566.99 & 25905.75 & 31497.77 & 47178.87 & 29440.27 & 188719.66 & 66614.68 & 2174736.93 \\
buildings per meter of street segment & 0.11 & 0.08 & 0.05 & 0.08 & 0.07 & 0.10 & 0.05 & 0.09 & 0.05 & 0.06 & 0.05 & 0.10 & 0.05 & 0.09 & 0.07 & 0.02 \\
reached ETCs by neighbouring segments & 49.09 & 33.99 & 38.08 & 26.79 & 21.56 & 32.35 & 8.88 & 26.76 & 8.57 & 16.97 & 11.04 & 35.17 & 13.53 & 43.96 & 30.27 & 26.08 \\
reached area by neighbouring segments & 113290.06 & 88462.74 & 1591397.39 & 89313.79 & 97515.74 & 84060.33 & 145420.68 & 64059.40 & 151507.35 & 100616.06 & 132683.99 & 140813.94 & 119718.73 & 556190.10 & 211678.46 & 5556960.68 \\
reached ETCs by local street network & 166.98 & 126.07 & 110.89 & 90.93 & 74.36 & 102.39 & 28.79 & 99.87 & 27.00 & 56.17 & 36.29 & 103.45 & 43.97 & 123.39 & 93.33 & 71.94 \\
reached area by local street network & 451276.21 & 390719.33 & 5858316.88 & 369240.03 & 416784.68 & 316062.25 & 703631.50 & 296524.52 & 621126.10 & 439804.09 & 643746.00 & 506987.49 & 540975.07 & 1982158.35 & 794621.33 & 17403052.98 \\
reached ETCs by tessellation contiguity & 36.80 & 40.24 & 46.23 & 43.10 & 45.61 & 39.57 & 53.52 & 42.04 & 48.46 & 47.29 & 51.81 & 41.55 & 51.95 & 43.57 & 42.93 & 47.56 \\
reached area by tessellation contiguity & 60511.46 & 87537.63 & 2410926.40 & 115962.63 & 152810.21 & 63671.98 & 372984.21 & 73335.07 & 306427.88 & 173857.48 & 302746.84 & 136577.35 & 238390.55 & 692699.47 & 297667.66 & 14081627.81 \\
area of enclosure & 242778.35 & 95677.02 & 3591565.15 & 133719.21 & 105561.74 & 282930.77 & 28859.85 & 110195.65 & 31788.41 & 83656.67 & 29460.25 & 640071.17 & 63476.79 & 1854684.23 & 430998.35 & 44036373.80 \\
perimeter of enclosure & 2046.29 & 1360.81 & 7599.46 & 1693.62 & 1463.16 & 2380.50 & 683.27 & 1150.58 & 538.87 & 1299.32 & 671.29 & 3793.33 & 1009.07 & 5664.05 & 2992.30 & 21952.84 \\
circular compactness of enclosure & 0.40 & 0.39 & 0.40 & 0.38 & 0.38 & 0.42 & 0.44 & 0.41 & 0.45 & 0.39 & 0.40 & 0.38 & 0.40 & 0.39 & 0.38 & 0.38 \\
equivalent rectangular index of enclosure & 0.85 & 0.87 & 0.84 & 0.84 & 0.86 & 0.83 & 0.91 & 0.89 & 0.94 & 0.85 & 0.89 & 0.77 & 0.87 & 0.80 & 0.80 & 0.79 \\
compactness-weighted axis of enclosure & 515.77 & 344.74 & 1777.66 & 441.16 & 397.37 & 567.78 & 144.75 & 289.81 & 120.13 & 345.64 & 153.64 & 986.37 & 249.05 & 1434.02 & 780.52 & 5069.06 \\
orientation of enclosure & 19.24 & 25.62 & 21.39 & 16.18 & 20.88 & 27.07 & 20.23 & 23.04 & 24.93 & 21.09 & 21.77 & 20.39 & 22.00 & 21.52 & 24.08 & 22.66 \\
perimeter-weighted neighbours of enclosure & 0.01 & 0.01 & 0.01 & 0.02 & 0.08 & 0.02 & 0.04 & 0.02 & 0.08 & 0.12 & 0.06 & 0.05 & 9.94 & 0.11 & 0.01 & 0.01 \\
area-weighted ETCs of enclosure & 36.32 & 2.82 & 746.28 & 3.03 & 4.63 & 2137242.86 & 0.00 & 0.43 & 0.00 & 0.14 & 0.01 & 330422.61 & 0.01 & 1178106554.77 & 627879.43 & 2270509.66 \\
Population & 4.51 & 8.57 & 1.91 & 10.02 & 17.52 & 6.55 & 36.91 & 7.74 & 37.93 & 28.87 & 43.70 & 5.06 & 42.99 & 3.43 & 6.93 & 1.31 \\
Night lights & 11.02 & 19.99 & 1.39 & 22.63 & 34.74 & 12.35 & 115.70 & 15.17 & 183.23 & 51.19 & 87.38 & 10.96 & 67.53 & 5.08 & 18.29 & 0.48 \\
Workplace population [Agriculture, energy and water] & 0.01 & 0.03 & 0.08 & 0.07 & 0.11 & 0.02 & 2.44 & 0.03 & 1.41 & 0.18 & 1.01 & 0.04 & 0.39 & 0.05 & 0.10 & 0.11 \\
Workplace population [Manufacturing] & 0.12 & 0.29 & 0.22 & 0.64 & 1.10 & 0.21 & 12.80 & 0.36 & 20.14 & 1.32 & 4.18 & 0.42 & 2.03 & 0.38 & 1.25 & 0.09 \\
Workplace population [Construction] & 0.12 & 0.22 & 0.10 & 0.33 & 0.56 & 0.18 & 9.16 & 0.20 & 10.68 & 0.80 & 3.80 & 0.17 & 1.40 & 0.14 & 0.34 & 0.07 \\
Workplace population [Distribution, hotels and restaurants] & 0.21 & 0.61 & 0.19 & 1.17 & 2.30 & 0.38 & 54.16 & 0.73 & 152.31 & 4.16 & 22.76 & 0.45 & 11.90 & 0.32 & 1.00 & 0.12 \\
Workplace population [Transport and communication] & 0.07 & 0.21 & 0.07 & 0.41 & 0.88 & 0.13 & 39.51 & 0.18 & 97.90 & 1.96 & 18.93 & 0.16 & 5.70 & 0.14 & 0.51 & 0.04 \\
Workplace population [Financial, real estate, professional and administrative activities] & 0.15 & 0.40 & 0.13 & 0.78 & 1.81 & 0.26 & 258.67 & 0.38 & 172.75 & 4.89 & 65.30 & 0.27 & 16.45 & 0.21 & 0.61 & 0.06 \\
Workplace population [Public administration, education and health] & 0.43 & 0.94 & 0.22 & 1.67 & 3.21 & 0.59 & 41.70 & 0.98 & 30.82 & 5.71 & 42.90 & 0.59 & 14.50 & 0.39 & 1.06 & 0.12 \\
Workplace population [Other] & 0.06 & 0.15 & 0.05 & 0.26 & 0.56 & 0.10 & 23.06 & 0.17 & 38.16 & 1.14 & 8.74 & 0.09 & 3.40 & 0.07 & 0.16 & 0.03 \\
Land cover [Airports] & 0.00 & 0.00 & 0.00 & 0.00 & 0.00 & 0.00 & 0.00 & 0.00 & 0.00 & 0.00 & 0.00 & 0.00 & 0.00 & 0.00 & 0.00 & 0.00 \\
Land cover [Non-irrigated arable land] & 0.00 & 0.00 & 0.35 & 0.00 & 0.00 & 0.00 & 0.00 & 0.00 & 0.00 & 0.00 & 0.00 & 0.01 & 0.00 & 0.11 & 0.02 & 0.15 \\
Land cover [Industrial or commercial units] & 0.00 & 0.02 & 0.01 & 0.05 & 0.09 & 0.01 & 0.00 & 0.00 & 0.00 & 0.09 & 0.01 & 0.03 & 0.06 & 0.03 & 0.14 & 0.00 \\
Land cover [Salt marshes] & 0.00 & 0.00 & 0.00 & 0.00 & 0.00 & 0.00 & 0.00 & 0.00 & 0.01 & 0.00 & 0.00 & 0.00 & 0.00 & 0.00 & 0.00 & 0.00 \\
Land cover [Estuaries] & 0.00 & 0.00 & 0.00 & 0.00 & 0.00 & 0.00 & 0.00 & 0.00 & 0.00 & 0.00 & 0.00 & 0.00 & 0.00 & 0.00 & 0.00 & 0.00 \\
Land cover [Sport and leisure facilities] & 0.00 & 0.00 & 0.02 & 0.00 & 0.00 & 0.00 & 0.00 & 0.00 & 0.00 & 0.00 & 0.00 & 0.01 & 0.00 & 0.02 & 0.01 & 0.01 \\
Land cover [Green urban areas] & 0.01 & 0.01 & 0.00 & 0.01 & 0.01 & 0.00 & 0.03 & 0.00 & 0.00 & 0.01 & 0.03 & 0.01 & 0.01 & 0.00 & 0.02 & 0.00 \\
Land cover [Discontinuous urban fabric] & 0.98 & 0.95 & 0.20 & 0.88 & 0.75 & 0.98 & 0.06 & 0.92 & 0.00 & 0.63 & 0.08 & 0.91 & 0.34 & 0.68 & 0.77 & 0.03 \\
Land cover [Pastures] & 0.00 & 0.00 & 0.36 & 0.00 & 0.00 & 0.00 & 0.00 & 0.00 & 0.02 & 0.00 & 0.00 & 0.02 & 0.00 & 0.12 & 0.02 & 0.59 \\
Land cover [Broad-leaved forest] & 0.00 & 0.00 & 0.02 & 0.00 & 0.00 & 0.00 & 0.00 & 0.00 & 0.00 & 0.00 & 0.00 & 0.00 & 0.00 & 0.01 & 0.00 & 0.03 \\
Land cover [Mineral extraction sites] & 0.00 & 0.00 & 0.00 & 0.00 & 0.00 & 0.00 & 0.00 & 0.00 & 0.00 & 0.00 & 0.00 & 0.00 & 0.00 & 0.00 & 0.00 & 0.00 \\
Land cover [Port areas] & 0.00 & 0.00 & 0.00 & 0.00 & 0.01 & 0.00 & 0.00 & 0.00 & 0.00 & 0.01 & 0.00 & 0.00 & 0.01 & 0.00 & 0.01 & 0.00 \\
Land cover [Road and rail networks and associated land] & 0.00 & 0.00 & 0.00 & 0.00 & 0.00 & 0.00 & 0.00 & 0.00 & 0.00 & 0.00 & 0.00 & 0.00 & 0.00 & 0.00 & 0.00 & 0.00 \\
Land cover [Water bodies] & 0.00 & 0.00 & 0.00 & 0.00 & 0.00 & 0.00 & 0.00 & 0.00 & 0.00 & 0.00 & 0.00 & 0.00 & 0.00 & 0.00 & 0.00 & 0.00 \\
Land cover [Land principally occupied by agriculture, with significant areas of natural vegetation] & 0.00 & 0.00 & 0.01 & 0.00 & 0.00 & 0.00 & 0.00 & 0.00 & 0.00 & 0.00 & 0.00 & 0.00 & 0.00 & 0.00 & 0.00 & 0.01 \\
Land cover [Mixed forest] & 0.00 & 0.00 & 0.01 & 0.00 & 0.00 & 0.00 & 0.00 & 0.00 & 0.00 & 0.00 & 0.00 & 0.00 & 0.00 & 0.00 & 0.00 & 0.02 \\
Land cover [Peat bogs] & 0.00 & 0.00 & 0.00 & 0.00 & 0.00 & 0.00 & 0.00 & 0.00 & 0.00 & 0.00 & 0.00 & 0.00 & 0.00 & 0.00 & 0.00 & 0.02 \\
Land cover [Natural grasslands] & 0.00 & 0.00 & 0.00 & 0.00 & 0.00 & 0.00 & 0.00 & 0.00 & 0.00 & 0.00 & 0.00 & 0.00 & 0.00 & 0.00 & 0.00 & 0.04 \\
Land cover [Moors and heathland] & 0.00 & 0.00 & 0.00 & 0.00 & 0.00 & 0.00 & 0.00 & 0.00 & 0.00 & 0.00 & 0.00 & 0.00 & 0.00 & 0.00 & 0.00 & 0.04 \\
Land cover [Transitional woodland-shrub] & 0.00 & 0.00 & 0.00 & 0.00 & 0.00 & 0.00 & 0.00 & 0.00 & 0.00 & 0.00 & 0.00 & 0.00 & 0.00 & 0.00 & 0.00 & 0.01 \\
Land cover [Continuous urban fabric] & 0.00 & 0.02 & 0.00 & 0.04 & 0.13 & 0.00 & 0.90 & 0.07 & 0.97 & 0.25 & 0.88 & 0.00 & 0.57 & 0.00 & 0.00 & 0.00 \\
Land cover [Intertidal flats] & 0.00 & 0.00 & 0.00 & 0.00 & 0.00 & 0.00 & 0.00 & 0.00 & 0.00 & 0.00 & 0.00 & 0.00 & 0.00 & 0.00 & 0.00 & 0.00 \\
Land cover [Sea and ocean] & 0.00 & 0.00 & 0.00 & 0.00 & 0.00 & 0.00 & 0.00 & 0.00 & 0.00 & 0.00 & 0.00 & 0.00 & 0.00 & 0.00 & 0.00 & 0.00 \\
Land cover [Construction sites] & 0.00 & 0.00 & 0.00 & 0.00 & 0.00 & 0.00 & 0.00 & 0.00 & 0.00 & 0.00 & 0.00 & 0.00 & 0.00 & 0.01 & 0.00 & 0.00 \\
Land cover [Burnt areas] & 0.00 & 0.00 & 0.00 & 0.00 & 0.00 & 0.00 & 0.00 & 0.00 & 0.00 & 0.00 & 0.00 & 0.00 & 0.00 & 0.00 & 0.00 & 0.00 \\
Land cover [Dump sites] & 0.00 & 0.00 & 0.00 & 0.00 & 0.00 & 0.00 & 0.00 & 0.00 & 0.00 & 0.00 & 0.00 & 0.00 & 0.00 & 0.00 & 0.00 & 0.00 \\
Land cover [Complex cultivation patterns] & 0.00 & 0.00 & 0.00 & 0.00 & 0.00 & 0.00 & 0.00 & 0.00 & 0.00 & 0.00 & 0.00 & 0.00 & 0.00 & 0.00 & 0.00 & 0.00 \\
Land cover [Inland marshes] & 0.00 & 0.00 & 0.00 & 0.00 & 0.00 & 0.00 & 0.00 & 0.00 & 0.00 & 0.00 & 0.00 & 0.00 & 0.00 & 0.00 & 0.00 & 0.00 \\
Land cover [Water courses] & 0.00 & 0.00 & 0.00 & 0.00 & 0.00 & 0.00 & 0.01 & 0.00 & 0.00 & 0.00 & 0.01 & 0.00 & 0.01 & 0.00 & 0.00 & 0.00 \\
Land cover [Coniferous forest] & 0.00 & 0.00 & 0.01 & 0.00 & 0.00 & 0.00 & 0.00 & 0.00 & 0.00 & 0.00 & 0.00 & 0.00 & 0.00 & 0.00 & 0.00 & 0.04 \\
Land cover [Bare rocks] & 0.00 & 0.00 & 0.00 & 0.00 & 0.00 & 0.00 & 0.00 & 0.00 & 0.00 & 0.00 & 0.00 & 0.00 & 0.00 & 0.00 & 0.00 & 0.00 \\
Land cover [Coastal lagoons] & 0.00 & 0.00 & 0.00 & 0.00 & 0.00 & 0.00 & 0.00 & 0.00 & 0.00 & 0.00 & 0.00 & 0.00 & 0.00 & 0.00 & 0.00 & 0.00 \\
Land cover [Beaches, dunes, sands] & 0.00 & 0.00 & 0.00 & 0.00 & 0.00 & 0.00 & 0.00 & 0.00 & 0.00 & 0.00 & 0.00 & 0.00 & 0.00 & 0.00 & 0.00 & 0.00 \\
Land cover [Agro-forestry areas] & 0.00 & 0.00 & 0.00 & 0.00 & 0.00 & 0.00 & 0.00 & 0.00 & 0.00 & 0.00 & 0.00 & 0.00 & 0.00 & 0.00 & 0.00 & 0.00 \\
Land cover [Sparsely vegetated areas] & 0.00 & 0.00 & 0.00 & 0.00 & 0.00 & 0.00 & 0.00 & 0.00 & 0.00 & 0.00 & 0.00 & 0.00 & 0.00 & 0.00 & 0.00 & 0.00 \\
Land cover [Fruit trees and berry plantations] & 0.00 & 0.00 & 0.00 & 0.00 & 0.00 & 0.00 & 0.00 & 0.00 & 0.00 & 0.00 & 0.00 & 0.00 & 0.00 & 0.00 & 0.00 & 0.00 \\
NDVI & 0.29 & 0.25 & 0.48 & 0.23 & 0.19 & 0.29 & 0.03 & 0.21 & 0.00 & 0.16 & 0.06 & 0.29 & 0.11 & 0.37 & 0.29 & 0.56 \\
Supermarkets [distance to nearest] & 828.82 & 679.96 & 4751.23 & 661.77 & 587.28 & 761.86 & 229.90 & 577.68 & 324.42 & 483.02 & 299.93 & 948.03 & 331.07 & 1752.87 & 1043.84 & 9854.12 \\
Supermarkets [counts within 1200m] & 1.89 & 2.86 & 0.09 & 3.13 & 4.44 & 2.07 & 22.51 & 3.41 & 18.79 & 6.85 & 17.27 & 1.47 & 12.53 & 0.65 & 1.43 & 0.03 \\
Listed buildings [distance to nearest] & 744.22 & 596.61 & 557.94 & 506.61 & 350.89 & 729.61 & 31.73 & 516.20 & 69.75 & 216.86 & 51.87 & 760.26 & 115.00 & 673.93 & 934.00 & 1324.03 \\
Listed buildings [counts within 1200m] & 11.27 & 24.28 & 11.22 & 37.47 & 62.78 & 24.18 & 685.16 & 31.77 & 1142.57 & 140.03 & 456.53 & 18.17 & 324.50 & 16.14 & 10.57 & 4.21 \\
FHRS points [distance to nearest] & 218.46 & 152.48 & 725.69 & 144.02 & 106.08 & 217.95 & 16.22 & 129.24 & 14.10 & 82.47 & 40.06 & 267.24 & 56.87 & 379.17 & 256.22 & 1699.17 \\
FHRS points [counts within 1200m] & 334.43 & 692.66 & 44.47 & 860.93 & 1568.44 & 342.08 & 6297.61 & 1081.38 & 9213.15 & 2167.91 & 4490.95 & 253.88 & 3163.83 & 132.66 & 271.09 & 33.07 \\
Cultural venues [distance to nearest] & 5384.64 & 3946.05 & 13156.20 & 3497.51 & 2287.43 & 5831.52 & 702.75 & 4094.92 & 351.33 & 1273.23 & 644.53 & 6309.75 & 850.25 & 8939.65 & 5121.47 & 20695.29 \\
Cultural venues [counts within 1200m] & 0.06 & 0.13 & 0.00 & 0.26 & 0.48 & 0.08 & 10.39 & 0.24 & 34.20 & 1.13 & 4.45 & 0.06 & 2.23 & 0.02 & 0.06 & 0.00 \\
Water bodies [distance to nearest] & 542.61 & 555.96 & 304.49 & 483.12 & 528.85 & 523.05 & 565.25 & 522.09 & 759.60 & 507.71 & 467.71 & 378.36 & 461.42 & 345.79 & 417.43 & 236.73 \\
Retail centres [distance to nearest] & 849.45 & 536.47 & 4943.97 & 421.09 & 224.33 & 725.57 & 29.80 & 445.52 & 32.54 & 161.85 & 66.32 & 1002.66 & 90.87 & 2102.46 & 898.17 & 11041.32 \\
\bottomrule
\end{tabular}
Figure of context¶
Create a figure illustrating context.
tess = geopandas.read_parquet("../../urbangrammar_samba/spatial_signatures/morphometrics/cells/cells_76.pq", columns=["hindex", "tessellation"])
from shapely.geometry import Point
import urbangrammar_graphics as ugg
import contextily
from matplotlib_scalebar.scalebar import ScaleBar
import seaborn as sns
import matplotlib.pyplot as plt
minx, maxx, miny, maxy = (357500, 360000, 173500, 176000)
subset = tess.cx[minx:maxx, miny:maxy]
central = subset[subset.intersects(Point((maxx+minx) / 2, (maxy+miny) / 2))]
import momepy
W = momepy.sw_high(10, subset, ids="hindex")
neighbors = subset[subset.hindex.isin(W.neighbors['c076e329053t0011'])]
neighbors["distance"] = neighbors.centroid.distance(central.centroid.iloc[0])
/opt/conda/lib/python3.9/site-packages/geopandas/geodataframe.py:1351: SettingWithCopyWarning:
A value is trying to be set on a copy of a slice from a DataFrame.
Try using .loc[row_indexer,col_indexer] = value instead
See the caveats in the documentation: https://pandas.pydata.org/pandas-docs/stable/user_guide/indexing.html#returning-a-view-versus-a-copy
super().__setitem__(key, value)
ax = subset.clip(Point((maxx+minx) / 2, (maxy+miny) / 2).buffer(1200)).plot(figsize=(12, 12), facecolor="none", edgecolor="w", linewidth=1)
central.plot(ax=ax, color=ugg.COLORS[4])
neighbors.plot("distance", ax=ax, cmap=sns.light_palette(ugg.COLORS[1], 256, as_cmap=True, reverse=True), edgecolor="w", linewidth=.5, alpha=.8)
# buildings.plot(ax=ax, color=ugg.COLORS[1], alpha=.1, zorder=-1)
ax.set_axis_off()
scalebar = ScaleBar(dx=1,
color="w",
location='lower right',
height_fraction=0.002,
pad=.5,
frameon=False,
)
ax.add_artist(scalebar)
contextily.add_basemap(ax=ax, source=contextily.providers.Esri.WorldImagery, crs=subset.crs)
plt.savefig("cell_context.png", dpi=150, bbox_inches="tight")
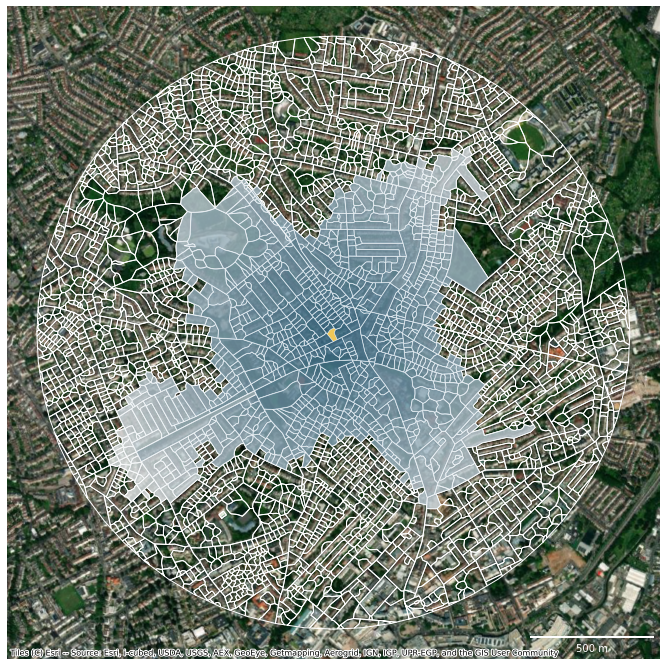
Feature importance¶
importances = pandas.read_csv("../../urbangrammar_samba/spatial_signatures/clustering_data/spsig_feature_importance.csv", index_col=0)
importances.index = importances.index.map(renamer)
importances.columns = ["relative importance"]
print(importances.head(10).to_latex(longtable=True))
\begin{longtable}{lr}
\toprule
{} & relative importance \\
\midrule
\endfirsthead
\toprule
{} & relative importance \\
\midrule
\endhead
\midrule
\multicolumn{2}{r}{{Continued on next page}} \\
\midrule
\endfoot
\bottomrule
\endlastfoot
covered area ratio of ETC (Q1) & 0.036944 \\
covered area ratio of ETC (Q2) & 0.031717 \\
perimeter-weighted neighbours of ETC (Q2) & 0.023476 \\
mean inter-building distance (Q2) & 0.016662 \\
area of ETC (Q3) & 0.016005 \\
area covered by node-attached ETCs (Q3) & 0.014813 \\
longest axis length of ETC (Q2) & 0.014501 \\
weighted reached enclosures of ETC (Q1) & 0.014115 \\
reached area by neighbouring segments (Q3) & 0.014000 \\
reached area by neighbouring segments (Q1) & 0.013904 \\
\end{longtable}
importances.head(10).sum()
relative importance 0.196135
dtype: float64
importances.to_excel("importances.xlsx")
type_imp = pandas.read_parquet("../../urbangrammar_samba/spatial_signatures/clustering_data/per_cluster_importance.pq")
classes = [
'cluster_4', 'cluster_0', 'cluster_6', 'cluster_1', 'cluster_21',
'cluster_7', 'cluster_3', 'cluster_5', 'cluster_90', 'cluster_20',
'cluster_8', 'cluster_22', 'cluster_92', 'cluster_94', 'cluster_91',
'cluster_95'
]
type_imp[classes] = type_imp[classes].apply(lambda x: x.map(renamer))
types = {
0: "Countryside agriculture",
1: "Accessible suburbia",
3: "Open sprawl",
4: "Wild countryside",
5: "Warehouse/Park land",
6: "Gridded residential quarters",
7: "Urban buffer",
8: "Disconnected suburbia",
20: "Dense residential neighbourhoods",
21: "Connected residential neighbourhoods",
22: "Dense urban neighbourhoods",
90: "Local urbanity",
91: "Concentrated urbanity",
92: "Regional urbanity",
94: "Metropolitan urbanity",
95: "Hyper concentrated urbanity",
93: "outlier",
96: "outlier",
97: "outlier",
98: "outlier",
}
tups = []
for c in type_imp.columns:
if 'vals' in c:
tups.append((types[int(c[8:-5])], 'rel. importance'))
else:
tups.append((types[int(c[8:])], 'name'))
type_imp.columns = pandas.MultiIndex.from_tuples(tups)
print(type_imp.iloc[:10].round(3).to_latex(longtable=True))
\begin{longtable}{llrlrlrlrlrlrlrlrlrlrlrlrlrlrlrlr}
\toprule
{} & \multicolumn{2}{l}{Wild countryside} & \multicolumn{2}{l}{Countryside agriculture} & \multicolumn{2}{l}{Gridded residential quarters} & \multicolumn{2}{l}{Accessible suburbia} & \multicolumn{2}{l}{Connected residential neighbourhoods} & \multicolumn{2}{l}{Urban buffer} & \multicolumn{2}{l}{Open sprawl} & \multicolumn{2}{l}{Warehouse/Park land} & \multicolumn{2}{l}{Local urbanity} & \multicolumn{2}{l}{Dense residential neighbourhoods} & \multicolumn{2}{l}{Disconnected suburbia} & \multicolumn{2}{l}{Dense urban neighbourhoods} & \multicolumn{2}{l}{Regional urbanity} & \multicolumn{2}{l}{Metropolitan urbanity} & \multicolumn{2}{l}{Concentrated urbanity} & \multicolumn{2}{l}{Hyper concentrated urbanity} \\
{} & name & rel. importance & name & rel. importance & name & rel. importance & name & rel. importance & name & rel. importance & name & rel. importance & name & rel. importance & name & rel. importance & name & rel. importance & name & rel. importance & name & rel. importance & name & rel. importance & name & rel. importance & name & rel. importance & name & rel. importance & name & rel. importance \\
\midrule
\endfirsthead
\toprule
{} & \multicolumn{2}{l}{Wild countryside} & \multicolumn{2}{l}{Countryside agriculture} & \multicolumn{2}{l}{Gridded residential quarters} & \multicolumn{2}{l}{Accessible suburbia} & \multicolumn{2}{l}{Connected residential neighbourhoods} & \multicolumn{2}{l}{Urban buffer} & \multicolumn{2}{l}{Open sprawl} & \multicolumn{2}{l}{Warehouse/Park land} & \multicolumn{2}{l}{Local urbanity} & \multicolumn{2}{l}{Dense residential neighbourhoods} & \multicolumn{2}{l}{Disconnected suburbia} & \multicolumn{2}{l}{Dense urban neighbourhoods} & \multicolumn{2}{l}{Regional urbanity} & \multicolumn{2}{l}{Metropolitan urbanity} & \multicolumn{2}{l}{Concentrated urbanity} & \multicolumn{2}{l}{Hyper concentrated urbanity} \\
{} & name & rel. importance & name & rel. importance & name & rel. importance & name & rel. importance & name & rel. importance & name & rel. importance & name & rel. importance & name & rel. importance & name & rel. importance & name & rel. importance & name & rel. importance & name & rel. importance & name & rel. importance & name & rel. importance & name & rel. importance & name & rel. importance \\
\midrule
\endhead
\midrule
\multicolumn{33}{r}{{Continued on next page}} \\
\midrule
\endfoot
\bottomrule
\endlastfoot
0 & longest axis length of ETC (Q1) & 0.197 & covered area ratio of ETC (Q1) & 0.154 & local closeness of street network (Q3) & 0.095 & weighted reached enclosures of ETC (Q3) & 0.064 & cell alignment of building (Q1) & 0.028 & area covered by neighbouring cells (Q2) & 0.072 & reached area by local street network (Q1) & 0.058 & elongation of building (Q1) & 0.034 & perimeter of building (Q2) & 0.101 & centroid - corner mean distance of building (Q2) & 0.037 & local proportion of cul-de-sacs of street netw... & 0.024 & perimeter of building (Q2) & 0.107 & centroid - corner distance deviation of buildi... & 0.115 & equivalent rectangular index of building (Q2) & 0.111 & area of building (Q1) & 0.128 & covered area ratio of ETC (Q2) & 0.154 \\
1 & covered area ratio of ETC (Q2) & 0.151 & covered area ratio of ETC (Q2) & 0.144 & local closeness of street network (Q2) & 0.046 & reached ETCs by tessellation contiguity (Q3) & 0.062 & local proportion of 4-way intersections of str... & 0.023 & covered area ratio of ETC (Q2) & 0.050 & reached area by neighbouring segments (Q1) & 0.034 & centroid - corner mean distance of building (Q3) & 0.028 & equivalent rectangular index of building (Q1) & 0.094 & centroid - corner mean distance of building (Q3) & 0.030 & local meshedness of street network (Q3) & 0.021 & centroid - corner mean distance of building (Q2) & 0.084 & centroid - corner mean distance of building (Q2) & 0.088 & centroid - corner mean distance of building (Q2) & 0.087 & Workplace population [Distribution, hotels and... & 0.100 & Workplace population [Manufacturing] (Q2) & 0.144 \\
2 & covered area ratio of ETC (Q1) & 0.146 & mean inter-building distance (Q2) & 0.079 & perimeter of enclosure (Q1) & 0.044 & reached area by tessellation contiguity (Q2) & 0.048 & cell alignment of building (Q2) & 0.017 & mean distance to neighbouring nodes of street ... & 0.049 & area covered by node-attached ETCs (Q2) & 0.024 & elongation of building (Q2) & 0.025 & centroid - corner mean distance of building (Q2) & 0.082 & area of building (Q3) & 0.029 & local meshedness of street network (Q2) & 0.021 & perimeter of building (Q3) & 0.082 & squareness of building (Q3) & 0.082 & centroid - corner distance deviation of buildi... & 0.081 & Workplace population [Financial, real estate, ... & 0.077 & Workplace population [Other] (Q2) & 0.102 \\
3 & area of ETC (Q2) & 0.096 & area of ETC (Q2) & 0.073 & area of enclosure (Q2) & 0.037 & area of ETC (Q2) & 0.045 & area of enclosure (Q2) & 0.017 & covered area ratio of ETC (Q1) & 0.046 & covered area ratio of ETC (Q2) & 0.022 & circular compactness of building (Q1) & 0.020 & squareness of building (Q3) & 0.054 & Population (Q3) & 0.028 & equivalent rectangular index of building (Q1) & 0.020 & area of building (Q2) & 0.066 & Workplace population [Financial, real estate, ... & 0.071 & corners of building (Q2) & 0.072 & Workplace population [Other] (Q2) & 0.076 & Workplace population [Distribution, hotels and... & 0.082 \\
4 & perimeter-weighted neighbours of ETC (Q3) & 0.075 & area covered by node-attached ETCs (Q2) & 0.067 & local closeness of street network (Q1) & 0.037 & reached ETCs by neighbouring segments (Q1) & 0.037 & orientation of ETC (Q2) & 0.017 & reached area by neighbouring segments (Q1) & 0.038 & local node density of street network (Q3) & 0.019 & centroid - corner distance deviation of buildi... & 0.018 & area of building (Q2) & 0.051 & perimeter of building (Q2) & 0.026 & circular compactness of building (Q1) & 0.019 & Population (Q3) & 0.040 & perimeter of building (Q2) & 0.065 & Workplace population [Financial, real estate, ... & 0.060 & Workplace population [Distribution, hotels and... & 0.071 & covered area ratio of ETC (Q1) & 0.079 \\
5 & reached area by neighbouring segments (Q1) & 0.049 & mean distance to neighbouring nodes of street ... & 0.066 & weighted reached enclosures of ETC (Q3) & 0.032 & reached ETCs by neighbouring segments (Q2) & 0.030 & equivalent rectangular index of building (Q1) & 0.016 & circular compactness of ETC (Q2) & 0.035 & reached area by neighbouring segments (Q2) & 0.018 & perimeter of building (Q3) & 0.017 & centroid - corner distance deviation of buildi... & 0.045 & area of building (Q2) & 0.023 & Population (Q1) & 0.018 & squareness of building (Q3) & 0.039 & perimeter of building (Q3) & 0.058 & Workplace population [Distribution, hotels and... & 0.051 & Workplace population [Financial, real estate, ... & 0.060 & Workplace population [Manufacturing] (Q3) & 0.075 \\
6 & reached area by tessellation contiguity (Q1) & 0.018 & reached area by neighbouring segments (Q1) & 0.063 & local proportion of 4-way intersections of str... & 0.021 & reached ETCs by local street network (Q2) & 0.026 & local proportion of 4-way intersections of str... & 0.014 & area covered by neighbouring cells (Q1) & 0.033 & covered area ratio of ETC (Q1) & 0.018 & width of street profile (Q2) & 0.017 & Workplace population [Financial, real estate, ... & 0.044 & perimeter of enclosure (Q1) & 0.021 & elongation of building (Q2) & 0.016 & centroid - corner distance deviation of buildi... & 0.034 & area of building (Q2) & 0.050 & perimeter of building (Q2) & 0.047 & Workplace population [Manufacturing] (Q2) & 0.055 & centroid - corner mean distance of building (Q2) & 0.070 \\
7 & area of ETC (Q3) & 0.016 & Land cover [Discontinuous urban fabric] (Q2) & 0.055 & area covered by node-attached ETCs (Q1) & 0.019 & perimeter-weighted neighbours of ETC (Q1) & 0.024 & perimeter of enclosure (Q1) & 0.014 & buildings per meter of street segment (Q2) & 0.032 & area of enclosure (Q2) & 0.017 & circular compactness of building (Q2) & 0.016 & Workplace population [Distribution, hotels and... & 0.035 & orientation of enclosure (Q2) & 0.018 & reached area by neighbouring segments (Q2) & 0.016 & Workplace population [Financial, real estate, ... & 0.029 & Workplace population [Distribution, hotels and... & 0.049 & squareness of building (Q3) & 0.039 & perimeter of building (Q2) & 0.047 & perimeter of building (Q2) & 0.055 \\
8 & mean distance between neighbouring buildings (Q2) & 0.015 & perimeter-weighted neighbours of ETC (Q2) & 0.022 & area covered by node-attached ETCs (Q2) & 0.018 & reached area by tessellation contiguity (Q1) & 0.023 & local proportion of cul-de-sacs of street netw... & 0.014 & reached area by tessellation contiguity (Q1) & 0.030 & compactness-weighted axis of enclosure (Q3) & 0.017 & reached area by tessellation contiguity (Q1) & 0.016 & perimeter of building (Q3) & 0.034 & perimeter of building (Q3) & 0.017 & area covered by edge-attached ETCs (Q3) & 0.016 & equivalent rectangular index of building (Q1) & 0.018 & corners of building (Q3) & 0.029 & Workplace population [Financial, real estate, ... & 0.030 & centroid - corner mean distance of building (Q2) & 0.045 & openness of street profile (Q2) & 0.031 \\
9 & mean inter-building distance (Q2) & 0.011 & longest axis length of ETC (Q2) & 0.021 & weighted reached enclosures of ETC (Q2) & 0.017 & reached ETCs by local street network (Q1) & 0.020 & orientation of enclosure (Q1) & 0.013 & area covered by node-attached ETCs (Q3) & 0.028 & area of ETC (Q2) & 0.016 & perimeter of building (Q2) & 0.015 & area of building (Q1) & 0.023 & area of enclosure (Q1) & 0.015 & circular compactness of building (Q2) & 0.015 & Workplace population [Other] (Q2) & 0.016 & centroid - corner distance deviation of buildi... & 0.021 & centroid - corner mean distance of building (Q1) & 0.019 & Land cover [Non-irrigated arable land] (Q1) & 0.026 & NDVI (Q3) & 0.027 \\
\end{longtable}
type_imp.iloc[:10].round(3).T
0 | 1 | 2 | 3 | 4 | 5 | 6 | 7 | 8 | 9 | ||
---|---|---|---|---|---|---|---|---|---|---|---|
Wild countryside | name | longest axis length of ETC (Q1) | covered area ratio of ETC (Q2) | covered area ratio of ETC (Q1) | area of ETC (Q2) | perimeter-weighted neighbours of ETC (Q3) | reached area by neighbouring segments (Q1) | reached area by tessellation contiguity (Q1) | area of ETC (Q3) | mean distance between neighbouring buildings (Q2) | mean inter-building distance (Q2) |
rel. importance | 0.197 | 0.151 | 0.146 | 0.096 | 0.075 | 0.049 | 0.018 | 0.016 | 0.015 | 0.011 | |
Countryside agriculture | name | covered area ratio of ETC (Q1) | covered area ratio of ETC (Q2) | mean inter-building distance (Q2) | area of ETC (Q2) | area covered by node-attached ETCs (Q2) | mean distance to neighbouring nodes of street ... | reached area by neighbouring segments (Q1) | Land cover [Discontinuous urban fabric] (Q2) | perimeter-weighted neighbours of ETC (Q2) | longest axis length of ETC (Q2) |
rel. importance | 0.154 | 0.144 | 0.079 | 0.073 | 0.067 | 0.066 | 0.063 | 0.055 | 0.022 | 0.021 | |
Gridded residential quarters | name | local closeness of street network (Q3) | local closeness of street network (Q2) | perimeter of enclosure (Q1) | area of enclosure (Q2) | local closeness of street network (Q1) | weighted reached enclosures of ETC (Q3) | local proportion of 4-way intersections of str... | area covered by node-attached ETCs (Q1) | area covered by node-attached ETCs (Q2) | weighted reached enclosures of ETC (Q2) |
rel. importance | 0.095 | 0.046 | 0.044 | 0.037 | 0.037 | 0.032 | 0.021 | 0.019 | 0.018 | 0.017 | |
Accessible suburbia | name | weighted reached enclosures of ETC (Q3) | reached ETCs by tessellation contiguity (Q3) | reached area by tessellation contiguity (Q2) | area of ETC (Q2) | reached ETCs by neighbouring segments (Q1) | reached ETCs by neighbouring segments (Q2) | reached ETCs by local street network (Q2) | perimeter-weighted neighbours of ETC (Q1) | reached area by tessellation contiguity (Q1) | reached ETCs by local street network (Q1) |
rel. importance | 0.064 | 0.062 | 0.048 | 0.045 | 0.037 | 0.03 | 0.026 | 0.024 | 0.023 | 0.02 | |
Connected residential neighbourhoods | name | cell alignment of building (Q1) | local proportion of 4-way intersections of str... | cell alignment of building (Q2) | area of enclosure (Q2) | orientation of ETC (Q2) | equivalent rectangular index of building (Q1) | local proportion of 4-way intersections of str... | perimeter of enclosure (Q1) | local proportion of cul-de-sacs of street netw... | orientation of enclosure (Q1) |
rel. importance | 0.028 | 0.023 | 0.017 | 0.017 | 0.017 | 0.016 | 0.014 | 0.014 | 0.014 | 0.013 | |
Urban buffer | name | area covered by neighbouring cells (Q2) | covered area ratio of ETC (Q2) | mean distance to neighbouring nodes of street ... | covered area ratio of ETC (Q1) | reached area by neighbouring segments (Q1) | circular compactness of ETC (Q2) | area covered by neighbouring cells (Q1) | buildings per meter of street segment (Q2) | reached area by tessellation contiguity (Q1) | area covered by node-attached ETCs (Q3) |
rel. importance | 0.072 | 0.05 | 0.049 | 0.046 | 0.038 | 0.035 | 0.033 | 0.032 | 0.03 | 0.028 | |
Open sprawl | name | reached area by local street network (Q1) | reached area by neighbouring segments (Q1) | area covered by node-attached ETCs (Q2) | covered area ratio of ETC (Q2) | local node density of street network (Q3) | reached area by neighbouring segments (Q2) | covered area ratio of ETC (Q1) | area of enclosure (Q2) | compactness-weighted axis of enclosure (Q3) | area of ETC (Q2) |
rel. importance | 0.058 | 0.034 | 0.024 | 0.022 | 0.019 | 0.018 | 0.018 | 0.017 | 0.017 | 0.016 | |
Warehouse/Park land | name | elongation of building (Q1) | centroid - corner mean distance of building (Q3) | elongation of building (Q2) | circular compactness of building (Q1) | centroid - corner distance deviation of buildi... | perimeter of building (Q3) | width of street profile (Q2) | circular compactness of building (Q2) | reached area by tessellation contiguity (Q1) | perimeter of building (Q2) |
rel. importance | 0.034 | 0.028 | 0.025 | 0.02 | 0.018 | 0.017 | 0.017 | 0.016 | 0.016 | 0.015 | |
Local urbanity | name | perimeter of building (Q2) | equivalent rectangular index of building (Q1) | centroid - corner mean distance of building (Q2) | squareness of building (Q3) | area of building (Q2) | centroid - corner distance deviation of buildi... | Workplace population [Financial, real estate, ... | Workplace population [Distribution, hotels and... | perimeter of building (Q3) | area of building (Q1) |
rel. importance | 0.101 | 0.094 | 0.082 | 0.054 | 0.051 | 0.045 | 0.044 | 0.035 | 0.034 | 0.023 | |
Dense residential neighbourhoods | name | centroid - corner mean distance of building (Q2) | centroid - corner mean distance of building (Q3) | area of building (Q3) | Population (Q3) | perimeter of building (Q2) | area of building (Q2) | perimeter of enclosure (Q1) | orientation of enclosure (Q2) | perimeter of building (Q3) | area of enclosure (Q1) |
rel. importance | 0.037 | 0.03 | 0.029 | 0.028 | 0.026 | 0.023 | 0.021 | 0.018 | 0.017 | 0.015 | |
Disconnected suburbia | name | local proportion of cul-de-sacs of street netw... | local meshedness of street network (Q3) | local meshedness of street network (Q2) | equivalent rectangular index of building (Q1) | circular compactness of building (Q1) | Population (Q1) | elongation of building (Q2) | reached area by neighbouring segments (Q2) | area covered by edge-attached ETCs (Q3) | circular compactness of building (Q2) |
rel. importance | 0.024 | 0.021 | 0.021 | 0.02 | 0.019 | 0.018 | 0.016 | 0.016 | 0.016 | 0.015 | |
Dense urban neighbourhoods | name | perimeter of building (Q2) | centroid - corner mean distance of building (Q2) | perimeter of building (Q3) | area of building (Q2) | Population (Q3) | squareness of building (Q3) | centroid - corner distance deviation of buildi... | Workplace population [Financial, real estate, ... | equivalent rectangular index of building (Q1) | Workplace population [Other] (Q2) |
rel. importance | 0.107 | 0.084 | 0.082 | 0.066 | 0.04 | 0.039 | 0.034 | 0.029 | 0.018 | 0.016 | |
Regional urbanity | name | centroid - corner distance deviation of buildi... | centroid - corner mean distance of building (Q2) | squareness of building (Q3) | Workplace population [Financial, real estate, ... | perimeter of building (Q2) | perimeter of building (Q3) | area of building (Q2) | Workplace population [Distribution, hotels and... | corners of building (Q3) | centroid - corner distance deviation of buildi... |
rel. importance | 0.115 | 0.088 | 0.082 | 0.071 | 0.065 | 0.058 | 0.05 | 0.049 | 0.029 | 0.021 | |
Metropolitan urbanity | name | equivalent rectangular index of building (Q2) | centroid - corner mean distance of building (Q2) | centroid - corner distance deviation of buildi... | corners of building (Q2) | Workplace population [Financial, real estate, ... | Workplace population [Distribution, hotels and... | perimeter of building (Q2) | squareness of building (Q3) | Workplace population [Financial, real estate, ... | centroid - corner mean distance of building (Q1) |
rel. importance | 0.111 | 0.087 | 0.081 | 0.072 | 0.06 | 0.051 | 0.047 | 0.039 | 0.03 | 0.019 | |
Concentrated urbanity | name | area of building (Q1) | Workplace population [Distribution, hotels and... | Workplace population [Financial, real estate, ... | Workplace population [Other] (Q2) | Workplace population [Distribution, hotels and... | Workplace population [Financial, real estate, ... | Workplace population [Manufacturing] (Q2) | perimeter of building (Q2) | centroid - corner mean distance of building (Q2) | Land cover [Non-irrigated arable land] (Q1) |
rel. importance | 0.128 | 0.1 | 0.077 | 0.076 | 0.071 | 0.06 | 0.055 | 0.047 | 0.045 | 0.026 | |
Hyper concentrated urbanity | name | covered area ratio of ETC (Q2) | Workplace population [Manufacturing] (Q2) | Workplace population [Other] (Q2) | Workplace population [Distribution, hotels and... | covered area ratio of ETC (Q1) | Workplace population [Manufacturing] (Q3) | centroid - corner mean distance of building (Q2) | perimeter of building (Q2) | openness of street profile (Q2) | NDVI (Q3) |
rel. importance | 0.154 | 0.144 | 0.102 | 0.082 | 0.079 | 0.075 | 0.07 | 0.055 | 0.031 | 0.027 |
type_imp.to_excel("importances_type.xlsx")