External validation¶
This notebook compares classifiction of Great Britain using spatial signatures with three other classifications - WorldPop urban footprints, MODUM and Copernicus Urban Atlas. This comparison serves as an external validation of signatures.
import geopandas as gpd
import pandas as pd
import seaborn as sns
import scipy as sp
import numpy as np
import xarray
import rioxarray
import rasterstats
import matplotlib.pyplot as plt
from itertools import product
import tobler
import dask_geopandas
import urbangrammar_graphics as ugg
from shapely.geometry import box
from download import download
from geocube.api.core import make_geocube
def cramers_v(confusion_matrix):
chi2 = sp.stats.chi2_contingency(confusion_matrix)[0]
n = confusion_matrix.sum().sum()
phi2 = chi2/n
r,k = confusion_matrix.shape
phi2corr = max(0, phi2-((k-1)*(r-1))/(n-1))
rcorr = r-((r-1)**2)/(n-1)
kcorr = k-((k-1)**2)/(n-1)
return np.sqrt(phi2corr/min((kcorr-1),(rcorr-1)))
WorldPop urban classification¶
Download and read data.
soton_path = download("https://eprints.soton.ac.uk/446482/1/GB_100m_mbclust6_3x3maj.tif",
'../../urbangrammar_samba/spatial_signatures/validation/B_100m_mbclust6_3x3maj.tif')
Replace is False and data exists, so doing nothing. Use replace=True to re-download the data.
soton = rioxarray.open_rasterio("../../urbangrammar_samba/spatial_signatures/validation/B_100m_mbclust6_3x3maj.tif")
signatures = gpd.read_parquet("../../urbangrammar_samba/spatial_signatures/signatures/signatures_combined_levels_simplified.pq")
/opt/conda/lib/python3.8/site-packages/ipykernel/ipkernel.py:283: DeprecationWarning: `should_run_async` will not call `transform_cell` automatically in the future. Please pass the result to `transformed_cell` argument and any exception that happen during thetransform in `preprocessing_exc_tuple` in IPython 7.17 and above.
and should_run_async(code)
Reproject.
soton.rio.crs
CRS.from_wkt('PROJCS["Airy_1830_Transverse_Mercator",GEOGCS["Unknown datum based upon the Airy 1830 ellipsoid",DATUM["unknown",SPHEROID["Airy 1830",6377563.396,299.3249646,AUTHORITY["EPSG","7001"]]],PRIMEM["Greenwich",0],UNIT["degree",0.0174532925199433,AUTHORITY["EPSG","9122"]]],PROJECTION["Transverse_Mercator"],PARAMETER["latitude_of_origin",49],PARAMETER["central_meridian",-2],PARAMETER["scale_factor",0.9996012717],PARAMETER["false_easting",400000],PARAMETER["false_northing",-100000],UNIT["metre",1,AUTHORITY["EPSG","9001"]],AXIS["Easting",EAST],AXIS["Northing",NORTH]]')
soton_osgb = soton.rio.reproject("EPSG:27700")
Get signature type as float.
signatures.signature_type = (signatures.kmeans10gb * 10) + signatures.level2
signatures
kmeans10gb | geometry | level2 | signature_type | |
---|---|---|---|---|
0 | 0 | POLYGON ((62219.999 798499.999, 62109.999 7985... | 0.0 | 0.0 |
1 | 0 | POLYGON ((63507.682 796515.168, 63471.096 7965... | 0.0 | 0.0 |
2 | 0 | POLYGON ((65953.174 802246.171, 65523.864 8023... | 0.0 | 0.0 |
3 | 0 | POLYGON ((67297.740 803435.799, 67220.290 8034... | 0.0 | 0.0 |
4 | 0 | POLYGON ((75760.000 852669.999, 75699.999 8527... | 0.0 | 0.0 |
... | ... | ... | ... | ... |
96699 | 9 | POLYGON ((323321.005 463795.415, 323236.741 46... | 8.0 | 98.0 |
96700 | 9 | POLYGON ((325929.840 1008792.060, 325890.989 1... | 8.0 | 98.0 |
96701 | 9 | POLYGON ((337804.769 1013422.582, 337547.855 1... | 8.0 | 98.0 |
96702 | 9 | POLYGON ((422304.270 1147826.989, 422296.000 1... | 8.0 | 98.0 |
96703 | 9 | POLYGON ((525396.260 439215.479, 525360.919 43... | 8.0 | 98.0 |
96704 rows × 4 columns
Rasterise signatures.
%%time
signatures_raster = make_geocube(signatures, measurements=["signature_type"], like=soton_osgb)
/opt/conda/lib/python3.8/site-packages/ipykernel/ipkernel.py:283: DeprecationWarning: `should_run_async` will not call `transform_cell` automatically in the future. Please pass the result to `transformed_cell` argument and any exception that happen during thetransform in `preprocessing_exc_tuple` in IPython 7.17 and above.
and should_run_async(code)
CPU times: user 19.2 s, sys: 772 ms, total: 20 s
Wall time: 20 s
Save raster to file.
signatures_raster.signature_type.rio.to_raster("signatures_raster.tif")
/opt/conda/lib/python3.8/site-packages/ipykernel/ipkernel.py:283: DeprecationWarning: `should_run_async` will not call `transform_cell` automatically in the future. Please pass the result to `transformed_cell` argument and any exception that happen during thetransform in `preprocessing_exc_tuple` in IPython 7.17 and above.
and should_run_async(code)
Create cross tabulation. There may be a smarter way but this one works.
spsig_vals = signatures.signature_type.unique()
soton_vals = [1, 2, 3, 4, 5, 6, 15]
crosstab = pd.DataFrame(columns=soton_vals, index=spsig_vals)
for sps, so in product(spsig_vals, soton_vals):
crosstab.loc[sps, so] = np.logical_and(signatures_raster.signature_type == sps, soton_osgb[0] == so).data.sum()
crosstab
1 | 2 | 3 | 4 | 5 | 6 | 15 | |
---|---|---|---|---|---|---|---|
0.0 | 245644 | 261108 | 92204 | 63004 | 28364 | 72865 | 8622602 |
10.0 | 168 | 322 | 106061 | 31752 | 2523 | 52230 | 31393 |
30.0 | 2192 | 2879 | 118522 | 73965 | 20202 | 102378 | 187853 |
40.0 | 86139 | 72260 | 3923 | 5522 | 5364 | 5755 | 8951880 |
50.0 | 1714 | 1596 | 10016 | 47775 | 25739 | 42455 | 116893 |
60.0 | 2 | 3 | 2845 | 10010 | 937 | 10284 | 2044 |
70.0 | 62430 | 77909 | 214575 | 92106 | 35194 | 116073 | 2560377 |
80.0 | 5 | 42 | 18644 | 16477 | 1089 | 30128 | 4621 |
20.0 | 35 | 50 | 3052 | 37679 | 8640 | 30630 | 15670 |
21.0 | 46 | 35 | 5140 | 18442 | 2334 | 22985 | 7508 |
22.0 | 33 | 11 | 33 | 31936 | 10027 | 6249 | 8787 |
90.0 | 4 | 9 | 0 | 13547 | 7071 | 184 | 2264 |
91.0 | 0 | 0 | 0 | 0 | 709 | 0 | 86 |
92.0 | 1 | 0 | 0 | 1815 | 4964 | 1 | 888 |
93.0 | 0 | 0 | 0 | 0 | 2 | 0 | 13 |
94.0 | 3 | 2 | 0 | 34 | 1300 | 0 | 298 |
95.0 | 0 | 2 | 0 | 0 | 126 | 0 | 98 |
96.0 | 0 | 0 | 0 | 0 | 0 | 0 | 40 |
97.0 | 0 | 2 | 0 | 0 | 16 | 0 | 23 |
98.0 | 1 | 0 | 0 | 0 | 0 | 0 | 39 |
crosstab.columns = [str(c) for c in crosstab.columns]
crosstab.to_parquet("../../urbangrammar_samba/spatial_signatures/validation/ctab_worldpop.pq")
crosstab = pd.read_parquet("../../urbangrammar_samba/spatial_signatures/validation/ctab_worldpop.pq")
Plot percentages.
types = {
0: "Countryside agriculture",
10: "Accessible suburbia",
30: "Open sprawl",
40: "Wild countryside",
50: "Warehouse/Park land",
60: "Gridded residential quarters",
70: "Urban buffer",
80: "Disconnected suburbia",
20: "Dense residential neighbourhoods",
21: "Connected residential neighbourhoods",
22: "Dense urban neighbourhoods",
90: "Local urbanity",
91: "Concentrated urbanity",
92: "Regional urbanity",
94: "Metropolitan urbanity",
95: "Hyper concentrated urbanity",
}
crosstab = crosstab.drop([x for x in crosstab.index if x not in types.keys()])
crosstab.index = [types[x] for x in crosstab.index]
crosstab
1 | 2 | 3 | 4 | 5 | 6 | 15 | |
---|---|---|---|---|---|---|---|
Countryside agriculture | 245644 | 261108 | 92204 | 63004 | 28364 | 72865 | 8622602 |
Accessible suburbia | 168 | 322 | 106061 | 31752 | 2523 | 52230 | 31393 |
Open sprawl | 2192 | 2879 | 118522 | 73965 | 20202 | 102378 | 187853 |
Wild countryside | 86139 | 72260 | 3923 | 5522 | 5364 | 5755 | 8951880 |
Warehouse/Park land | 1714 | 1596 | 10016 | 47775 | 25739 | 42455 | 116893 |
Gridded residential quarters | 2 | 3 | 2845 | 10010 | 937 | 10284 | 2044 |
Urban buffer | 62430 | 77909 | 214575 | 92106 | 35194 | 116073 | 2560377 |
Disconnected suburbia | 5 | 42 | 18644 | 16477 | 1089 | 30128 | 4621 |
Dense residential neighbourhoods | 35 | 50 | 3052 | 37679 | 8640 | 30630 | 15670 |
Connected residential neighbourhoods | 46 | 35 | 5140 | 18442 | 2334 | 22985 | 7508 |
Dense urban neighbourhoods | 33 | 11 | 33 | 31936 | 10027 | 6249 | 8787 |
Local urbanity | 4 | 9 | 0 | 13547 | 7071 | 184 | 2264 |
Concentrated urbanity | 0 | 0 | 0 | 0 | 709 | 0 | 86 |
Regional urbanity | 1 | 0 | 0 | 1815 | 4964 | 1 | 888 |
Metropolitan urbanity | 3 | 2 | 0 | 34 | 1300 | 0 | 298 |
Hyper concentrated urbanity | 0 | 2 | 0 | 0 | 126 | 0 | 98 |
order = [
"Wild countryside",
"Countryside agriculture",
"Urban buffer",
"Open sprawl",
"Disconnected suburbia",
"Accessible suburbia",
"Warehouse/Park land",
"Gridded residential quarters",
"Connected residential neighbourhoods",
"Dense residential neighbourhoods",
"Dense urban neighbourhoods",
"Local urbanity",
"Regional urbanity",
"Metropolitan urbanity",
"Concentrated urbanity",
"Hyper concentrated urbanity",
]
crosstab = crosstab.loc[order]
crosstab.columns.name = "WorldPop class"
crosstab.index.name = "Spatial Signature type"
fig, ax = plt.subplots(figsize=(8, 8))
sns.heatmap(crosstab.divide(crosstab.sum(axis=1), axis=0).astype('float') * 100, cmap=sns.light_palette(ugg.HEX[2], n_colors=256), annot=True, fmt='.0f', vmax=100)
plt.savefig("crosstab_worldpop.pdf", bbox_inches="tight")
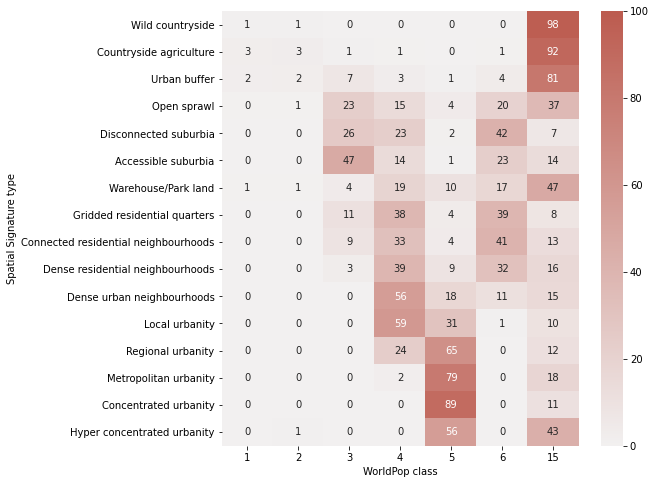
Compute chi-squared
chi = sp.stats.chi2_contingency(crosstab)
print(f"chi2: {chi[0]}, p: {chi[1]}, dof: {chi[2]}, N: {crosstab.sum().sum()}")
chi2: 13341832.31867016, p: 0.0, dof: 114, N: 22993921
Compute Cramer’s V.
cramers_v(crosstab)
0.3109737978543489
Results indicate moderate associtation.
MODUM¶
Load MODUM data (manually downloaded from CDRC).
modum = gpd.read_file("../../urbangrammar_samba/spatial_signatures/validation/modumew2016.zip")
modum
OA_CODE | LAD_NAME | REGION_NAM | CLUSTER_CO | CLUSTER_LA | geometry | |
---|---|---|---|---|---|---|
0 | E00044639 | Sunderland | North East | 5 | Waterside Settings | POLYGON ((440611.474 558272.251, 440527.700 55... |
1 | E00036646 | Wirral | North West | 4 | Victorian Terraces | POLYGON ((330917.000 394161.000, 330937.346 39... |
2 | E00092449 | Cheshire West and Chester | North West | 5 | Waterside Settings | POLYGON ((339836.566 367010.434, 339864.000 36... |
3 | E00044638 | Sunderland | North East | 8 | Central Business District | POLYGON ((440006.000 558293.000, 440091.001 55... |
4 | E00094441 | Cheshire West and Chester | North West | 1 | Suburban Landscapes | POLYGON ((364937.770 373630.624, 364860.312 37... |
... | ... | ... | ... | ... | ... | ... |
181403 | E00175248 | Westminster | London | 8 | Central Business District | POLYGON ((529580.098 179194.237, 529586.108 17... |
181404 | E00169799 | Leeds | Yorkshire and The Humber | 8 | Central Business District | POLYGON ((428969.394 433302.516, 428964.400 43... |
181405 | E00115865 | Gosport | South East | 5 | Waterside Settings | POLYGON ((461450.326 98059.723, 461404.687 981... |
181406 | E00175716 | Birmingham | West Midlands | 8 | Central Business District | POLYGON ((406120.776 286455.941, 406119.217 28... |
181407 | E00086517 | Portsmouth | South East | 8 | Central Business District | POLYGON ((464507.745 99838.197, 464507.715 998... |
181408 rows × 6 columns
Load ET celss with signature type label as Dask GeoDataFrame.
cells = dask_geopandas.read_parquet("../../urbangrammar_samba/spatial_signatures/signatures/signatures_combined_tessellation/")
cells
hindex | geometry | signature_type | |
---|---|---|---|
npartitions=103 | |||
object | geometry | int32 | |
... | ... | ... | |
... | ... | ... | ... |
... | ... | ... | |
... | ... | ... |
Interpolate MODUM classes to ET cells.
def _join_modum(ch):
tb = ch.total_bounds
modum_ch = modum.cx[tb[0]:tb[2], tb[1]:tb[3]]
return tobler.area_weighted.area_join(modum_ch, ch, ["CLUSTER_CO"])
meta = tobler.area_weighted.area_join(modum, cells._meta, ["CLUSTER_CO"])
modum_joined = cells.map_partitions(_join_modum, meta=meta)
/opt/conda/lib/python3.8/site-packages/IPython/core/interactiveshell.py:3357: UserWarning: CRS mismatch between the CRS of left geometries and the CRS of right geometries.
Use `to_crs()` to reproject one of the input geometries to match the CRS of the other.
Left CRS: None
Right CRS: EPSG:27700
if (await self.run_code(code, result, async_=asy)):
Select only subset of data needed for crosstab.
modum_data = modum_joined[["signature_type", "CLUSTER_CO"]]
Compute Dask-based steps.
%%time
modum_data = modum_data.compute()
/opt/conda/lib/python3.8/site-packages/ipykernel/ipkernel.py:283: DeprecationWarning: `should_run_async` will not call `transform_cell` automatically in the future. Please pass the result to `transformed_cell` argument and any exception that happen during thetransform in `preprocessing_exc_tuple` in IPython 7.17 and above.
and should_run_async(code)
/opt/conda/lib/python3.8/site-packages/tobler/area_weighted/area_join.py:63: UserWarning: Cannot preserve dtype of 'CLUSTER_CO'. Falling back to `dtype=object`.
warnings.warn(
CPU times: user 2h 38min 50s, sys: 20min 41s, total: 2h 59min 31s
Wall time: 38min 10s
modum_data
/opt/conda/lib/python3.8/site-packages/ipykernel/ipkernel.py:283: DeprecationWarning: `should_run_async` will not call `transform_cell` automatically in the future. Please pass the result to `transformed_cell` argument and any exception that happen during thetransform in `preprocessing_exc_tuple` in IPython 7.17 and above.
and should_run_async(code)
signature_type | CLUSTER_CO | |
---|---|---|
0 | 6 | 3 |
1 | 6 | 3 |
2 | 6 | 3 |
3 | 6 | 3 |
4 | 6 | 3 |
... | ... | ... |
139305 | 0 | 6 |
139306 | 0 | 6 |
139307 | 0 | 6 |
139308 | 0 | 6 |
139309 | 0 | 6 |
14539578 rows × 2 columns
Generate cross tabulation
crosstab = pd.crosstab(modum_data.signature_type, modum_data.CLUSTER_CO)
/opt/conda/lib/python3.8/site-packages/ipykernel/ipkernel.py:283: DeprecationWarning: `should_run_async` will not call `transform_cell` automatically in the future. Please pass the result to `transformed_cell` argument and any exception that happen during thetransform in `preprocessing_exc_tuple` in IPython 7.17 and above.
and should_run_async(code)
crosstab
/opt/conda/lib/python3.8/site-packages/ipykernel/ipkernel.py:283: DeprecationWarning: `should_run_async` will not call `transform_cell` automatically in the future. Please pass the result to `transformed_cell` argument and any exception that happen during thetransform in `preprocessing_exc_tuple` in IPython 7.17 and above.
and should_run_async(code)
CLUSTER_CO | 1 | 2 | 3 | 4 | 5 | 6 | 7 | 8 |
---|---|---|---|---|---|---|---|---|
signature_type | ||||||||
0 | 69795 | 15802 | 4221 | 23472 | 182137 | 2476766 | 17319 | 368 |
1 | 965634 | 77081 | 4747 | 132642 | 74036 | 496728 | 35341 | 18402 |
3 | 732427 | 134316 | 31433 | 321086 | 269592 | 743994 | 80637 | 25704 |
4 | 530 | 179 | 5 | 275 | 8875 | 323286 | 66 | 62 |
5 | 181327 | 41638 | 4315 | 154154 | 74066 | 141490 | 21848 | 13472 |
6 | 55918 | 14731 | 6579 | 80570 | 9560 | 7671 | 7728 | 18096 |
7 | 518434 | 100597 | 27825 | 171963 | 341933 | 2075046 | 80646 | 6225 |
8 | 210331 | 30133 | 11048 | 157174 | 36122 | 68072 | 16956 | 15258 |
20 | 98914 | 59058 | 12731 | 147608 | 37505 | 17095 | 24222 | 57099 |
21 | 119297 | 28384 | 3409 | 101829 | 15030 | 11771 | 9894 | 24907 |
22 | 13726 | 33810 | 13937 | 75548 | 14257 | 3575 | 13439 | 59140 |
90 | 815 | 10831 | 11178 | 11802 | 3613 | 823 | 4803 | 35536 |
91 | 0 | 10 | 1099 | 0 | 0 | 0 | 41 | 238 |
92 | 94 | 1870 | 4779 | 542 | 427 | 26 | 1603 | 8812 |
93 | 0 | 0 | 0 | 0 | 1 | 1 | 0 | 0 |
94 | 0 | 258 | 1699 | 6 | 0 | 3 | 303 | 1090 |
95 | 3 | 0 | 239 | 0 | 0 | 2 | 18 | 1 |
96 | 0 | 0 | 0 | 0 | 0 | 1 | 0 | 0 |
97 | 0 | 0 | 0 | 0 | 2 | 0 | 0 | 0 |
98 | 0 | 0 | 0 | 0 | 1 | 1 | 1 | 0 |
crosstab.to_parquet("../../urbangrammar_samba/spatial_signatures/validation/ctab_modum.pq")
/opt/conda/lib/python3.8/site-packages/ipykernel/ipkernel.py:283: DeprecationWarning: `should_run_async` will not call `transform_cell` automatically in the future. Please pass the result to `transformed_cell` argument and any exception that happen during thetransform in `preprocessing_exc_tuple` in IPython 7.17 and above.
and should_run_async(code)
crosstab = pd.read_parquet("../../urbangrammar_samba/spatial_signatures/validation/ctab_modum.pq")
crosstab.index
Index(['0', '1', '3', '4', '5', '6', '7', '8', '20', '21', '22', '90', '91',
'92', '93', '94', '95', '96', '97', '98'],
dtype='object', name='signature_type')
types = {
0: "Countryside agriculture",
1: "Accessible suburbia",
3: "Open sprawl",
4: "Wild countryside",
5: "Warehouse/Park land",
6: "Gridded residential quarters",
7: "Urban buffer",
8: "Disconnected suburbia",
20: "Dense residential neighbourhoods",
21: "Connected residential neighbourhoods",
22: "Dense urban neighbourhoods",
90: "Local urbanity",
91: "Concentrated urbanity",
92: "Regional urbanity",
94: "Metropolitan urbanity",
95: "Hyper concentrated urbanity",
}
crosstab = crosstab.drop([x for x in crosstab.index if int(x) not in types.keys()])
crosstab.index = [types[int(x)] for x in crosstab.index]
crosstab
CLUSTER_CO | 1 | 2 | 3 | 4 | 5 | 6 | 7 | 8 |
---|---|---|---|---|---|---|---|---|
Countryside agriculture | 69795 | 15802 | 4221 | 23472 | 182137 | 2476766 | 17319 | 368 |
Accessible suburbia | 965634 | 77081 | 4747 | 132642 | 74036 | 496728 | 35341 | 18402 |
Open sprawl | 732427 | 134316 | 31433 | 321086 | 269592 | 743994 | 80637 | 25704 |
Wild countryside | 530 | 179 | 5 | 275 | 8875 | 323286 | 66 | 62 |
Warehouse/Park land | 181327 | 41638 | 4315 | 154154 | 74066 | 141490 | 21848 | 13472 |
Gridded residential quarters | 55918 | 14731 | 6579 | 80570 | 9560 | 7671 | 7728 | 18096 |
Urban buffer | 518434 | 100597 | 27825 | 171963 | 341933 | 2075046 | 80646 | 6225 |
Disconnected suburbia | 210331 | 30133 | 11048 | 157174 | 36122 | 68072 | 16956 | 15258 |
Dense residential neighbourhoods | 98914 | 59058 | 12731 | 147608 | 37505 | 17095 | 24222 | 57099 |
Connected residential neighbourhoods | 119297 | 28384 | 3409 | 101829 | 15030 | 11771 | 9894 | 24907 |
Dense urban neighbourhoods | 13726 | 33810 | 13937 | 75548 | 14257 | 3575 | 13439 | 59140 |
Local urbanity | 815 | 10831 | 11178 | 11802 | 3613 | 823 | 4803 | 35536 |
Concentrated urbanity | 0 | 10 | 1099 | 0 | 0 | 0 | 41 | 238 |
Regional urbanity | 94 | 1870 | 4779 | 542 | 427 | 26 | 1603 | 8812 |
Metropolitan urbanity | 0 | 258 | 1699 | 6 | 0 | 3 | 303 | 1090 |
Hyper concentrated urbanity | 3 | 0 | 239 | 0 | 0 | 2 | 18 | 1 |
crosstab = crosstab.loc[order]
crosstab
CLUSTER_CO | 1 | 2 | 3 | 4 | 5 | 6 | 7 | 8 |
---|---|---|---|---|---|---|---|---|
Wild countryside | 530 | 179 | 5 | 275 | 8875 | 323286 | 66 | 62 |
Countryside agriculture | 69795 | 15802 | 4221 | 23472 | 182137 | 2476766 | 17319 | 368 |
Urban buffer | 518434 | 100597 | 27825 | 171963 | 341933 | 2075046 | 80646 | 6225 |
Open sprawl | 732427 | 134316 | 31433 | 321086 | 269592 | 743994 | 80637 | 25704 |
Disconnected suburbia | 210331 | 30133 | 11048 | 157174 | 36122 | 68072 | 16956 | 15258 |
Accessible suburbia | 965634 | 77081 | 4747 | 132642 | 74036 | 496728 | 35341 | 18402 |
Warehouse/Park land | 181327 | 41638 | 4315 | 154154 | 74066 | 141490 | 21848 | 13472 |
Gridded residential quarters | 55918 | 14731 | 6579 | 80570 | 9560 | 7671 | 7728 | 18096 |
Connected residential neighbourhoods | 119297 | 28384 | 3409 | 101829 | 15030 | 11771 | 9894 | 24907 |
Dense residential neighbourhoods | 98914 | 59058 | 12731 | 147608 | 37505 | 17095 | 24222 | 57099 |
Dense urban neighbourhoods | 13726 | 33810 | 13937 | 75548 | 14257 | 3575 | 13439 | 59140 |
Local urbanity | 815 | 10831 | 11178 | 11802 | 3613 | 823 | 4803 | 35536 |
Regional urbanity | 94 | 1870 | 4779 | 542 | 427 | 26 | 1603 | 8812 |
Metropolitan urbanity | 0 | 258 | 1699 | 6 | 0 | 3 | 303 | 1090 |
Concentrated urbanity | 0 | 10 | 1099 | 0 | 0 | 0 | 41 | 238 |
Hyper concentrated urbanity | 3 | 0 | 239 | 0 | 0 | 2 | 18 | 1 |
names = [
"Suburban Landscapes",
"Railway Buzz",
"The Old Town",
"Victorian Terraces",
"Waterside Settings",
"Countryside Sceneries",
"High Street and Promenades",
"Central Business District",
]
crosstab.columns = names
crosstab.columns.name = 'MODUM class'
crosstab.index.name = "Spatial Signature type"
Plot percentages.
fig, ax = plt.subplots(figsize=(8, 8))
sns.heatmap(crosstab.divide(crosstab.sum(axis=1), axis=0).astype('float') * 100, cmap=sns.light_palette(ugg.HEX[2], n_colors=256), annot=True, fmt='.0f', vmax=100)
plt.savefig("crosstab_modum.pdf", bbox_inches="tight")
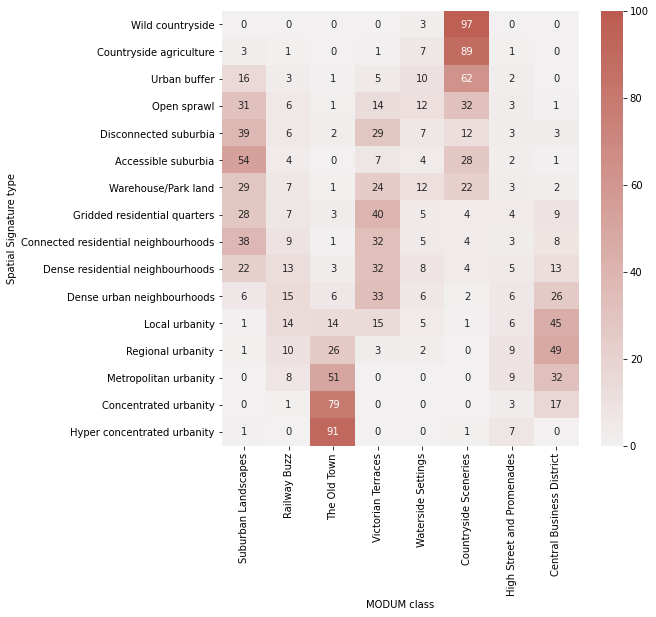
Compute chi-squared
chi = sp.stats.chi2_contingency(crosstab)
print(f"chi2: {chi[0]}, p: {chi[1]}, dof: {chi[2]}, N: {crosstab.sum().sum()}")
chi2: 13938867.969895832, p: 0.0, dof: 152, N: 13067584
/opt/conda/lib/python3.8/site-packages/ipykernel/ipkernel.py:283: DeprecationWarning: `should_run_async` will not call `transform_cell` automatically in the future. Please pass the result to `transformed_cell` argument and any exception that happen during thetransform in `preprocessing_exc_tuple` in IPython 7.17 and above.
and should_run_async(code)
Compute Cramer’s V.
cramers_v(crosstab)
/opt/conda/lib/python3.8/site-packages/ipykernel/ipkernel.py:283: DeprecationWarning: `should_run_async` will not call `transform_cell` automatically in the future. Please pass the result to `transformed_cell` argument and any exception that happen during thetransform in `preprocessing_exc_tuple` in IPython 7.17 and above.
and should_run_async(code)
0.3007137152411417
Results indicate moderate associtation.
Urban Atlas¶
Link is no longer valid and needs to be generated at https://land.copernicus.eu/local/urban-atlas/urban-atlas-2018/. The zip contains the full UK classification.
urban_atlas_path = download("https://land.copernicus.eu/land-files/f0dd642f9db5ca4de46b3cc955de135503446c43.zip",
'../../urbangrammar_samba/spatial_signatures/validation/urban_atlas/', kind="zip")
/opt/conda/lib/python3.8/site-packages/ipykernel/ipkernel.py:283: DeprecationWarning: `should_run_async` will not call `transform_cell` automatically in the future. Please pass the result to `transformed_cell` argument and any exception that happen during thetransform in `preprocessing_exc_tuple` in IPython 7.17 and above.
and should_run_async(code)
Creating data folder...
Downloading data from https://land.copernicus.eu/land-files/f0dd642f9db5ca4de46b3cc955de135503446c43.zip (2.50 GB)
file_sizes: 100%|██████████████████████████| 2.68G/2.68G [00:42<00:00, 63.5MB/s]
Extracting zip file...
Successfully downloaded / unzipped to ../../urbangrammar_samba/spatial_signatures/validation/urban_atlas/
urban_atlas_path = '../../urbangrammar_samba/spatial_signatures/validation/urban_atlas/'
/opt/conda/lib/python3.8/site-packages/ipykernel/ipkernel.py:283: DeprecationWarning: `should_run_async` will not call `transform_cell` automatically in the future. Please pass the result to `transformed_cell` argument and any exception that happen during thetransform in `preprocessing_exc_tuple` in IPython 7.17 and above.
and should_run_async(code)
import zipfile
from glob import glob
# zip file handler
a = zipfile.ZipFile(glob(urban_atlas_path + '*')[0])
/opt/conda/lib/python3.8/site-packages/ipykernel/ipkernel.py:283: DeprecationWarning: `should_run_async` will not call `transform_cell` automatically in the future. Please pass the result to `transformed_cell` argument and any exception that happen during thetransform in `preprocessing_exc_tuple` in IPython 7.17 and above.
and should_run_async(code)
a.namelist()
/opt/conda/lib/python3.8/site-packages/ipykernel/ipkernel.py:283: DeprecationWarning: `should_run_async` will not call `transform_cell` automatically in the future. Please pass the result to `transformed_cell` argument and any exception that happen during thetransform in `preprocessing_exc_tuple` in IPython 7.17 and above.
and should_run_async(code)
['UK566L1_NORWICH_UA2018_v012/Data/UK566L1_NORWICH_UA2018_v012.gpkg',
'UK566L1_NORWICH_UA2018_v012/Metadata/UK566L1_NORWICH_UA2018_v012.xml',
'UK566L1_NORWICH_UA2018_v012/Legend/Urban_Atlas_2018_Legend.lyr',
'UK566L1_NORWICH_UA2018_v012/Legend/Urban_Atlas_2018_Legend.qml',
'UK566L1_NORWICH_UA2018_v012/Legend/Urban_Atlas_2018_Legend.sld',
'UK566L1_NORWICH_UA2018_v012/Documents/UK566L1_NORWICH_UA2018_DELIVERY_REPORT.pdf',
'UK566L1_NORWICH_UA2018_v012/Documents/UK566L1_NORWICH_UA2018_MAP.pdf']
fuas = []
for file in glob(urban_atlas_path + '*'):
a = zipfile.ZipFile(file)
gdf = gpd.read_file(file + "!" + a.namelist()[0])
fuas.append(gdf)
print(file)
/opt/conda/lib/python3.8/site-packages/ipykernel/ipkernel.py:283: DeprecationWarning: `should_run_async` will not call `transform_cell` automatically in the future. Please pass the result to `transformed_cell` argument and any exception that happen during thetransform in `preprocessing_exc_tuple` in IPython 7.17 and above.
and should_run_async(code)
/opt/conda/lib/python3.8/site-packages/geopandas/geodataframe.py:577: RuntimeWarning: Sequential read of iterator was interrupted. Resetting iterator. This can negatively impact the performance.
for feature in features_lst:
../../urbangrammar_samba/spatial_signatures/validation/urban_atlas/UK566L1_NORWICH_UA2018_v012.zip
../../urbangrammar_samba/spatial_signatures/validation/urban_atlas/UK002L3_WEST_MIDLANDS_URBAN_AREA_UA2018_v012.zip
../../urbangrammar_samba/spatial_signatures/validation/urban_atlas/UK562L2_PRESTON_UA2018_v012.zip
../../urbangrammar_samba/spatial_signatures/validation/urban_atlas/UK009L1_CARDIFF_UA2018_v012.zip
../../urbangrammar_samba/spatial_signatures/validation/urban_atlas/UK517L1_SWANSEA_UA2018_v012.zip
../../urbangrammar_samba/spatial_signatures/validation/urban_atlas/UK026L1_KINGSTON_UPON_HULL_UA2018_v012.zip
../../urbangrammar_samba/spatial_signatures/validation/urban_atlas/UK056L1_HASTINGS_UA2018_v012.zip
../../urbangrammar_samba/spatial_signatures/validation/urban_atlas/UK539L1_BOURNEMOUTH_UA2018_v012.zip
../../urbangrammar_samba/spatial_signatures/validation/urban_atlas/UK033L1_GUILDFORD_UA2018_v012.zip
../../urbangrammar_samba/spatial_signatures/validation/urban_atlas/UK023L1_PORTSMOUTH_UA2018_v012.zip
../../urbangrammar_samba/spatial_signatures/validation/urban_atlas/UK013L2_NEWCASTLE_UPON_TYNE_UA2018_v012.zip
../../urbangrammar_samba/spatial_signatures/validation/urban_atlas/UK019L3_LINCOLN_UA2018_v012.zip
../../urbangrammar_samba/spatial_signatures/validation/urban_atlas/UK014L1_LEICESTER_UA2018_v012.zip
../../urbangrammar_samba/spatial_signatures/validation/urban_atlas/UK027L1_STOKE_ON_TRENT_UA2018_v012.zip
../../urbangrammar_samba/spatial_signatures/validation/urban_atlas/UK551L1_FALKIRK_UA2018_v012.zip
../../urbangrammar_samba/spatial_signatures/validation/urban_atlas/UK016L1_ABERDEEN_UA2018_v012.zip
../../urbangrammar_samba/spatial_signatures/validation/urban_atlas/UK011L2_BRISTOL_UA2018_v012.zip
../../urbangrammar_samba/spatial_signatures/validation/urban_atlas/UK568L1_CHESHIRE_WEST_AND_CHESTER_UA2018_v012.zip
../../urbangrammar_samba/spatial_signatures/validation/urban_atlas/UK546L1_COLCHESTER_UA2018_v012.zip
../../urbangrammar_samba/spatial_signatures/validation/urban_atlas/UK029L1_NOTTINGHAM_UA2018_v012.zip
../../urbangrammar_samba/spatial_signatures/validation/urban_atlas/UK516L1_PLYMOUTH_UA2018_v012.zip
../../urbangrammar_samba/spatial_signatures/validation/urban_atlas/UK006L3_LIVERPOOL_UA2018_v012.zip
../../urbangrammar_samba/spatial_signatures/validation/urban_atlas/UK012L2_BELFAST_UA2018_v012.zip
../../urbangrammar_samba/spatial_signatures/validation/urban_atlas/UK007L1_EDINBURGH_UA2018_v012.zip
../../urbangrammar_samba/spatial_signatures/validation/urban_atlas/UK558L1_NEWPORT_UA2018_v012.zip
../../urbangrammar_samba/spatial_signatures/validation/urban_atlas/UK550L0_DUNDEE_CITY_UA2018_v012.zip
../../urbangrammar_samba/spatial_signatures/validation/urban_atlas/UK050L1_BURNLEY_UA2018_v012.zip
../../urbangrammar_samba/spatial_signatures/validation/urban_atlas/UK024L1_WORCESTER_UA2018_v012.zip
../../urbangrammar_samba/spatial_signatures/validation/urban_atlas/UK553L1_BLACKPOOL_UA2018_v012.zip
../../urbangrammar_samba/spatial_signatures/validation/urban_atlas/UK001L3_LONDON_UA2018_v012.zip
../../urbangrammar_samba/spatial_signatures/validation/urban_atlas/UK520L2_SOUTHAMPTON_UA2018_v012.zip
../../urbangrammar_samba/spatial_signatures/validation/urban_atlas/UK008L3_GREATER_MANCHESTER_UA2018_v012.zip
../../urbangrammar_samba/spatial_signatures/validation/urban_atlas/UK515L1_BRIGHTON_AND_HOVE_UA2018_v012.zip
../../urbangrammar_samba/spatial_signatures/validation/urban_atlas/UK025L3_COVENTRY_UA2018_v012.zip
../../urbangrammar_samba/spatial_signatures/validation/urban_atlas/UK518L1_DERBY_UA2018_v012.zip
../../urbangrammar_samba/spatial_signatures/validation/urban_atlas/UK017L2_CAMBRIDGE_UA2018_v012.zip
../../urbangrammar_samba/spatial_signatures/validation/urban_atlas/UK557L1_BLACKBURN_WITH_DARWEN_UA2018_v012.zip
../../urbangrammar_samba/spatial_signatures/validation/urban_atlas/UK010L3_SHEFFIELD_UA2018_v012.zip
../../urbangrammar_samba/spatial_signatures/validation/urban_atlas/UK560L1_OXFORD_UA2018_v012.zip
../../urbangrammar_samba/spatial_signatures/validation/urban_atlas/UK018L3_EXETER_UA2018_v012.zip
../../urbangrammar_samba/spatial_signatures/validation/urban_atlas/UK569L2_IPSWICH_UA2018_v012.zip
../../urbangrammar_samba/spatial_signatures/validation/urban_atlas/UK528L1_NORTHAMPTON_UA2018_v012.zip
../../urbangrammar_samba/spatial_signatures/validation/urban_atlas/UK004L1_GLASGOW_UA2018_v012.zip
../../urbangrammar_samba/spatial_signatures/validation/urban_atlas/UK559L2_MIDDLESBROUGH_UA2018_v012.zip
../../urbangrammar_samba/spatial_signatures/validation/urban_atlas/UK571L1_CHELTENHAM_UA2018_v012.zip
../../urbangrammar_samba/spatial_signatures/validation/urban_atlas/UK003L2_LEEDS_UA2018_v012.zip
../../urbangrammar_samba/spatial_signatures/validation/urban_atlas/UK552L0_READING_UA2018_v012.zip
urban_atlas = pd.concat(fuas)
/opt/conda/lib/python3.8/site-packages/ipykernel/ipkernel.py:283: DeprecationWarning: `should_run_async` will not call `transform_cell` automatically in the future. Please pass the result to `transformed_cell` argument and any exception that happen during thetransform in `preprocessing_exc_tuple` in IPython 7.17 and above.
and should_run_async(code)
urban_atlas.crs
/opt/conda/lib/python3.8/site-packages/ipykernel/ipkernel.py:283: DeprecationWarning: `should_run_async` will not call `transform_cell` automatically in the future. Please pass the result to `transformed_cell` argument and any exception that happen during thetransform in `preprocessing_exc_tuple` in IPython 7.17 and above.
and should_run_async(code)
<Projected CRS: EPSG:3035>
Name: ETRS89-extended / LAEA Europe
Axis Info [cartesian]:
- Y[north]: Northing (metre)
- X[east]: Easting (metre)
Area of Use:
- name: Europe - European Union (EU) countries and candidates. Europe - onshore and offshore: Albania; Andorra; Austria; Belgium; Bosnia and Herzegovina; Bulgaria; Croatia; Cyprus; Czechia; Denmark; Estonia; Faroe Islands; Finland; France; Germany; Gibraltar; Greece; Hungary; Iceland; Ireland; Italy; Kosovo; Latvia; Liechtenstein; Lithuania; Luxembourg; Malta; Monaco; Montenegro; Netherlands; North Macedonia; Norway including Svalbard and Jan Mayen; Poland; Portugal including Madeira and Azores; Romania; San Marino; Serbia; Slovakia; Slovenia; Spain including Canary Islands; Sweden; Switzerland; Turkey; United Kingdom (UK) including Channel Islands and Isle of Man; Vatican City State.
- bounds: (-35.58, 24.6, 44.83, 84.17)
Coordinate Operation:
- name: Europe Equal Area 2001
- method: Lambert Azimuthal Equal Area
Datum: European Terrestrial Reference System 1989
- Ellipsoid: GRS 1980
- Prime Meridian: Greenwich
urban_atlas = urban_atlas.to_crs(27700)
/opt/conda/lib/python3.8/site-packages/ipykernel/ipkernel.py:283: DeprecationWarning: `should_run_async` will not call `transform_cell` automatically in the future. Please pass the result to `transformed_cell` argument and any exception that happen during thetransform in `preprocessing_exc_tuple` in IPython 7.17 and above.
and should_run_async(code)
urban_atlas = urban_atlas[urban_atlas.class_2018 != "Other roads and associated land"]
/opt/conda/lib/python3.8/site-packages/ipykernel/ipkernel.py:283: DeprecationWarning: `should_run_async` will not call `transform_cell` automatically in the future. Please pass the result to `transformed_cell` argument and any exception that happen during thetransform in `preprocessing_exc_tuple` in IPython 7.17 and above.
and should_run_async(code)
urban_atlas
country | fua_name | fua_code | code_2018 | class_2018 | prod_date | identifier | perimeter | area | comment | geometry | |
---|---|---|---|---|---|---|---|---|---|---|---|
0 | UK | Norwich | UK566L1 | 12100 | Industrial, commercial, public, military and p... | 2020-02 | 6191-UK566L1 | 395.405063 | 9578.604245 | None | MULTIPOLYGON (((620644.028 307123.158, 620624.... |
1 | UK | Norwich | UK566L1 | 12100 | Industrial, commercial, public, military and p... | 2020-02 | 6276-UK566L1 | 344.502008 | 5999.177060 | None | MULTIPOLYGON (((625449.063 309572.394, 625436.... |
2 | UK | Norwich | UK566L1 | 12100 | Industrial, commercial, public, military and p... | 2020-02 | 6319-UK566L1 | 408.686684 | 11324.997591 | None | MULTIPOLYGON (((627325.583 310096.486, 627334.... |
3 | UK | Norwich | UK566L1 | 12100 | Industrial, commercial, public, military and p... | 2020-02 | 6346-UK566L1 | 219.549474 | 2990.227266 | None | MULTIPOLYGON (((618204.340 310427.862, 618222.... |
4 | UK | Norwich | UK566L1 | 12100 | Industrial, commercial, public, military and p... | 2020-02 | 6365-UK566L1 | 486.728439 | 12997.641845 | None | MULTIPOLYGON (((620576.811 312663.606, 620626.... |
... | ... | ... | ... | ... | ... | ... | ... | ... | ... | ... | ... |
4197 | UK | Reading | UK552L0 | 11210 | Discontinuous dense urban fabric (S.L. : 50% -... | 2020-02 | 305-UK552L0 | 558.446702 | 14641.882260 | None | MULTIPOLYGON (((468779.192 173658.431, 468676.... |
4198 | UK | Reading | UK552L0 | 11220 | Discontinuous medium density urban fabric (S.L... | 2020-02 | 1044-UK552L0 | 870.186192 | 29291.038780 | None | MULTIPOLYGON (((467566.392 174849.590, 467553.... |
4199 | UK | Reading | UK552L0 | 11230 | Discontinuous low density urban fabric (S.L. :... | 2020-02 | 1415-UK552L0 | 1459.462751 | 58927.793763 | None | MULTIPOLYGON (((467100.219 175109.903, 467097.... |
4200 | UK | Reading | UK552L0 | 11230 | Discontinuous low density urban fabric (S.L. :... | 2020-02 | 1416-UK552L0 | 918.929836 | 40518.633162 | None | MULTIPOLYGON (((467413.737 175161.847, 467424.... |
4201 | UK | Reading | UK552L0 | 13300 | Construction sites | 2020-02 | 2616-UK552L0 | 507.509481 | 16769.773281 | None | MULTIPOLYGON (((481889.558 169963.353, 481799.... |
949178 rows × 11 columns
%%time
ddf_urban_atlas = dask_geopandas.from_geopandas(urban_atlas, npartitions=16)
ddf_urban_atlas.geometry = ddf_urban_atlas.geometry.simplify(5)
simplified_urban_atlas = ddf_urban_atlas.compute()
/opt/conda/lib/python3.8/site-packages/ipykernel/ipkernel.py:283: DeprecationWarning: `should_run_async` will not call `transform_cell` automatically in the future. Please pass the result to `transformed_cell` argument and any exception that happen during thetransform in `preprocessing_exc_tuple` in IPython 7.17 and above.
and should_run_async(code)
CPU times: user 3min 11s, sys: 483 ms, total: 3min 12s
Wall time: 15 s
%%time
def _join_ua(ch):
tb = ch.total_bounds
urban_atlas_ch = simplified_urban_atlas.cx[tb[0]:tb[2], tb[1]:tb[3]]
return tobler.area_weighted.area_join(urban_atlas_ch, ch, ["class_2018"])
meta = tobler.area_weighted.area_join(simplified_urban_atlas, cells._meta, ["class_2018"])
ua_joined = cells.map_partitions(_join_ua, meta=meta)
/opt/conda/lib/python3.8/site-packages/ipykernel/ipkernel.py:283: DeprecationWarning: `should_run_async` will not call `transform_cell` automatically in the future. Please pass the result to `transformed_cell` argument and any exception that happen during thetransform in `preprocessing_exc_tuple` in IPython 7.17 and above.
and should_run_async(code)
/opt/conda/lib/python3.8/site-packages/IPython/core/magic.py:187: UserWarning: CRS mismatch between the CRS of left geometries and the CRS of right geometries.
Use `to_crs()` to reproject one of the input geometries to match the CRS of the other.
Left CRS: None
Right CRS: EPSG:27700
call = lambda f, *a, **k: f(*a, **k)
CPU times: user 13.1 s, sys: 1.31 s, total: 14.4 s
Wall time: 14.4 s
ua_data = ua_joined[["signature_type", "class_2018"]]
%%time
ua_data = ua_data.compute()
CPU times: user 1h 55min 52s, sys: 14min 55s, total: 2h 10min 48s
Wall time: 29min 41s
ua_covered = ua_data.dropna()
/opt/conda/lib/python3.8/site-packages/ipykernel/ipkernel.py:283: DeprecationWarning: `should_run_async` will not call `transform_cell` automatically in the future. Please pass the result to `transformed_cell` argument and any exception that happen during thetransform in `preprocessing_exc_tuple` in IPython 7.17 and above.
and should_run_async(code)
ua_covered
/opt/conda/lib/python3.8/site-packages/ipykernel/ipkernel.py:283: DeprecationWarning: `should_run_async` will not call `transform_cell` automatically in the future. Please pass the result to `transformed_cell` argument and any exception that happen during thetransform in `preprocessing_exc_tuple` in IPython 7.17 and above.
and should_run_async(code)
signature_type | class_2018 | |
---|---|---|
0 | 6 | Discontinuous dense urban fabric (S.L. : 50% -... |
1 | 6 | Discontinuous dense urban fabric (S.L. : 50% -... |
2 | 6 | Discontinuous dense urban fabric (S.L. : 50% -... |
3 | 6 | Discontinuous dense urban fabric (S.L. : 50% -... |
4 | 6 | Discontinuous dense urban fabric (S.L. : 50% -... |
... | ... | ... |
139162 | 0 | Sports and leisure facilities |
139168 | 0 | Arable land (annual crops) |
139178 | 0 | Arable land (annual crops) |
139205 | 4 | Discontinuous very low density urban fabric (S... |
139206 | 4 | Discontinuous very low density urban fabric (S... |
8396642 rows × 2 columns
crosstab = pd.crosstab(ua_covered.signature_type, ua_covered.class_2018)
/opt/conda/lib/python3.8/site-packages/ipykernel/ipkernel.py:283: DeprecationWarning: `should_run_async` will not call `transform_cell` automatically in the future. Please pass the result to `transformed_cell` argument and any exception that happen during thetransform in `preprocessing_exc_tuple` in IPython 7.17 and above.
and should_run_async(code)
crosstab
/opt/conda/lib/python3.8/site-packages/ipykernel/ipkernel.py:283: DeprecationWarning: `should_run_async` will not call `transform_cell` automatically in the future. Please pass the result to `transformed_cell` argument and any exception that happen during thetransform in `preprocessing_exc_tuple` in IPython 7.17 and above.
and should_run_async(code)
class_2018 | Airports | Arable land (annual crops) | Complex and mixed cultivation patterns | Construction sites | Continuous urban fabric (S.L. : > 80%) | Discontinuous dense urban fabric (S.L. : 50% - 80%) | Discontinuous low density urban fabric (S.L. : 10% - 30%) | Discontinuous medium density urban fabric (S.L. : 30% - 50%) | Discontinuous very low density urban fabric (S.L. : < 10%) | Fast transit roads and associated land | ... | Mineral extraction and dump sites | Open spaces with little or no vegetation (beaches, dunes, bare rocks, glaciers) | Orchards at the fringe of urban classes | Pastures | Permanent crops (vineyards, fruit trees, olive groves) | Port areas | Railways and associated land | Sports and leisure facilities | Water | Wetlands |
---|---|---|---|---|---|---|---|---|---|---|---|---|---|---|---|---|---|---|---|---|---|
signature_type | |||||||||||||||||||||
0 | 1970 | 162715 | 35 | 2728 | 716 | 30725 | 180334 | 139190 | 70543 | 865 | ... | 2044 | 124 | 1 | 308524 | 108 | 403 | 772 | 19612 | 3055 | 570 |
1 | 116 | 1728 | 12 | 2160 | 7246 | 773300 | 55316 | 506555 | 2701 | 83 | ... | 130 | 149 | 0 | 4242 | 0 | 132 | 1362 | 19054 | 340 | 59 |
3 | 574 | 10599 | 3 | 10465 | 12418 | 753983 | 67639 | 559736 | 6299 | 1211 | ... | 1888 | 78 | 0 | 33717 | 6 | 521 | 2986 | 27642 | 1899 | 172 |
4 | 47 | 23891 | 3 | 49 | 27 | 1656 | 6652 | 5936 | 2711 | 32 | ... | 265 | 51 | 0 | 50629 | 0 | 7 | 67 | 938 | 416 | 64 |
5 | 1361 | 3938 | 0 | 2084 | 4644 | 217845 | 17828 | 149909 | 1657 | 795 | ... | 717 | 25 | 0 | 9753 | 0 | 6058 | 1284 | 11024 | 1377 | 79 |
6 | 0 | 10 | 0 | 125 | 23544 | 98085 | 1240 | 15678 | 56 | 23 | ... | 27 | 34 | 0 | 149 | 0 | 69 | 307 | 2141 | 119 | 3 |
7 | 1756 | 96530 | 25 | 31212 | 3894 | 381255 | 255892 | 670328 | 36046 | 2435 | ... | 3281 | 238 | 0 | 215022 | 64 | 739 | 2791 | 40338 | 4123 | 714 |
8 | 0 | 227 | 0 | 471 | 2069 | 197699 | 7840 | 121443 | 393 | 2 | ... | 34 | 4 | 0 | 863 | 0 | 0 | 477 | 4760 | 91 | 0 |
20 | 0 | 260 | 1 | 795 | 19834 | 242743 | 5128 | 70591 | 451 | 199 | ... | 101 | 62 | 0 | 990 | 0 | 1120 | 2115 | 8012 | 597 | 2 |
21 | 0 | 152 | 0 | 329 | 9477 | 203970 | 4842 | 69128 | 283 | 58 | ... | 72 | 36 | 0 | 577 | 0 | 61 | 916 | 4698 | 124 | 6 |
22 | 27 | 89 | 0 | 224 | 24064 | 121552 | 979 | 16398 | 35 | 148 | ... | 35 | 20 | 0 | 153 | 0 | 1373 | 2348 | 2651 | 608 | 0 |
90 | 0 | 9 | 0 | 130 | 12066 | 42757 | 154 | 3169 | 151 | 72 | ... | 0 | 16 | 0 | 2 | 0 | 437 | 1364 | 565 | 328 | 0 |
91 | 0 | 0 | 0 | 0 | 779 | 79 | 0 | 1 | 0 | 0 | ... | 0 | 0 | 0 | 0 | 0 | 0 | 9 | 1 | 5 | 0 |
92 | 0 | 0 | 0 | 87 | 6145 | 8267 | 33 | 238 | 0 | 59 | ... | 0 | 14 | 0 | 0 | 0 | 55 | 555 | 190 | 138 | 0 |
93 | 0 | 0 | 0 | 0 | 0 | 0 | 0 | 0 | 0 | 0 | ... | 0 | 0 | 0 | 0 | 0 | 0 | 0 | 1 | 0 | 0 |
94 | 0 | 0 | 0 | 2 | 1807 | 555 | 0 | 18 | 4 | 21 | ... | 0 | 1 | 0 | 0 | 0 | 0 | 192 | 9 | 16 | 0 |
95 | 0 | 0 | 0 | 0 | 238 | 8 | 0 | 0 | 0 | 0 | ... | 0 | 0 | 0 | 4 | 0 | 0 | 0 | 0 | 0 | 1 |
97 | 0 | 0 | 0 | 0 | 0 | 0 | 0 | 0 | 0 | 0 | ... | 0 | 0 | 0 | 0 | 0 | 91 | 0 | 0 | 5 | 0 |
98 | 0 | 0 | 0 | 0 | 0 | 0 | 0 | 0 | 0 | 0 | ... | 0 | 0 | 0 | 0 | 0 | 0 | 0 | 1 | 0 | 0 |
19 rows × 26 columns
crosstab.to_parquet("../../urbangrammar_samba/spatial_signatures/validation/ctab_ua.pq")
/opt/conda/lib/python3.8/site-packages/ipykernel/ipkernel.py:283: DeprecationWarning: `should_run_async` will not call `transform_cell` automatically in the future. Please pass the result to `transformed_cell` argument and any exception that happen during thetransform in `preprocessing_exc_tuple` in IPython 7.17 and above.
and should_run_async(code)
crosstab = pd.read_parquet("../../urbangrammar_samba/spatial_signatures/validation/ctab_ua.pq")
crosstab = crosstab.drop([x for x in crosstab.index if int(x) not in types.keys()])
crosstab.index = [types[int(x)] for x in crosstab.index]
crosstab
class_2018 | Airports | Arable land (annual crops) | Complex and mixed cultivation patterns | Construction sites | Continuous urban fabric (S.L. : > 80%) | Discontinuous dense urban fabric (S.L. : 50% - 80%) | Discontinuous low density urban fabric (S.L. : 10% - 30%) | Discontinuous medium density urban fabric (S.L. : 30% - 50%) | Discontinuous very low density urban fabric (S.L. : < 10%) | Fast transit roads and associated land | ... | Mineral extraction and dump sites | Open spaces with little or no vegetation (beaches, dunes, bare rocks, glaciers) | Orchards at the fringe of urban classes | Pastures | Permanent crops (vineyards, fruit trees, olive groves) | Port areas | Railways and associated land | Sports and leisure facilities | Water | Wetlands |
---|---|---|---|---|---|---|---|---|---|---|---|---|---|---|---|---|---|---|---|---|---|
Countryside agriculture | 1970 | 162715 | 35 | 2728 | 716 | 30725 | 180334 | 139190 | 70543 | 865 | ... | 2044 | 124 | 1 | 308524 | 108 | 403 | 772 | 19612 | 3055 | 570 |
Accessible suburbia | 116 | 1728 | 12 | 2160 | 7246 | 773300 | 55316 | 506555 | 2701 | 83 | ... | 130 | 149 | 0 | 4242 | 0 | 132 | 1362 | 19054 | 340 | 59 |
Open sprawl | 574 | 10599 | 3 | 10465 | 12418 | 753983 | 67639 | 559736 | 6299 | 1211 | ... | 1888 | 78 | 0 | 33717 | 6 | 521 | 2986 | 27642 | 1899 | 172 |
Wild countryside | 47 | 23891 | 3 | 49 | 27 | 1656 | 6652 | 5936 | 2711 | 32 | ... | 265 | 51 | 0 | 50629 | 0 | 7 | 67 | 938 | 416 | 64 |
Warehouse/Park land | 1361 | 3938 | 0 | 2084 | 4644 | 217845 | 17828 | 149909 | 1657 | 795 | ... | 717 | 25 | 0 | 9753 | 0 | 6058 | 1284 | 11024 | 1377 | 79 |
Gridded residential quarters | 0 | 10 | 0 | 125 | 23544 | 98085 | 1240 | 15678 | 56 | 23 | ... | 27 | 34 | 0 | 149 | 0 | 69 | 307 | 2141 | 119 | 3 |
Urban buffer | 1756 | 96530 | 25 | 31212 | 3894 | 381255 | 255892 | 670328 | 36046 | 2435 | ... | 3281 | 238 | 0 | 215022 | 64 | 739 | 2791 | 40338 | 4123 | 714 |
Disconnected suburbia | 0 | 227 | 0 | 471 | 2069 | 197699 | 7840 | 121443 | 393 | 2 | ... | 34 | 4 | 0 | 863 | 0 | 0 | 477 | 4760 | 91 | 0 |
Dense residential neighbourhoods | 0 | 260 | 1 | 795 | 19834 | 242743 | 5128 | 70591 | 451 | 199 | ... | 101 | 62 | 0 | 990 | 0 | 1120 | 2115 | 8012 | 597 | 2 |
Connected residential neighbourhoods | 0 | 152 | 0 | 329 | 9477 | 203970 | 4842 | 69128 | 283 | 58 | ... | 72 | 36 | 0 | 577 | 0 | 61 | 916 | 4698 | 124 | 6 |
Dense urban neighbourhoods | 27 | 89 | 0 | 224 | 24064 | 121552 | 979 | 16398 | 35 | 148 | ... | 35 | 20 | 0 | 153 | 0 | 1373 | 2348 | 2651 | 608 | 0 |
Local urbanity | 0 | 9 | 0 | 130 | 12066 | 42757 | 154 | 3169 | 151 | 72 | ... | 0 | 16 | 0 | 2 | 0 | 437 | 1364 | 565 | 328 | 0 |
Concentrated urbanity | 0 | 0 | 0 | 0 | 779 | 79 | 0 | 1 | 0 | 0 | ... | 0 | 0 | 0 | 0 | 0 | 0 | 9 | 1 | 5 | 0 |
Regional urbanity | 0 | 0 | 0 | 87 | 6145 | 8267 | 33 | 238 | 0 | 59 | ... | 0 | 14 | 0 | 0 | 0 | 55 | 555 | 190 | 138 | 0 |
Metropolitan urbanity | 0 | 0 | 0 | 2 | 1807 | 555 | 0 | 18 | 4 | 21 | ... | 0 | 1 | 0 | 0 | 0 | 0 | 192 | 9 | 16 | 0 |
Hyper concentrated urbanity | 0 | 0 | 0 | 0 | 238 | 8 | 0 | 0 | 0 | 0 | ... | 0 | 0 | 0 | 4 | 0 | 0 | 0 | 0 | 0 | 1 |
16 rows × 26 columns
crosstab.columns.name = "Urban Atlas class"
crosstab.index.name = "Spatial Signature type"
fig, ax = plt.subplots(figsize=(12, 8))
sns.heatmap(crosstab.divide(crosstab.sum(axis=1), axis=0).astype('float') * 100, cmap=sns.light_palette(ugg.HEX[2], n_colors=256), annot=True, fmt='.0f', vmax=100)
plt.savefig("crosstab_ua.pdf", bbox_inches="tight")
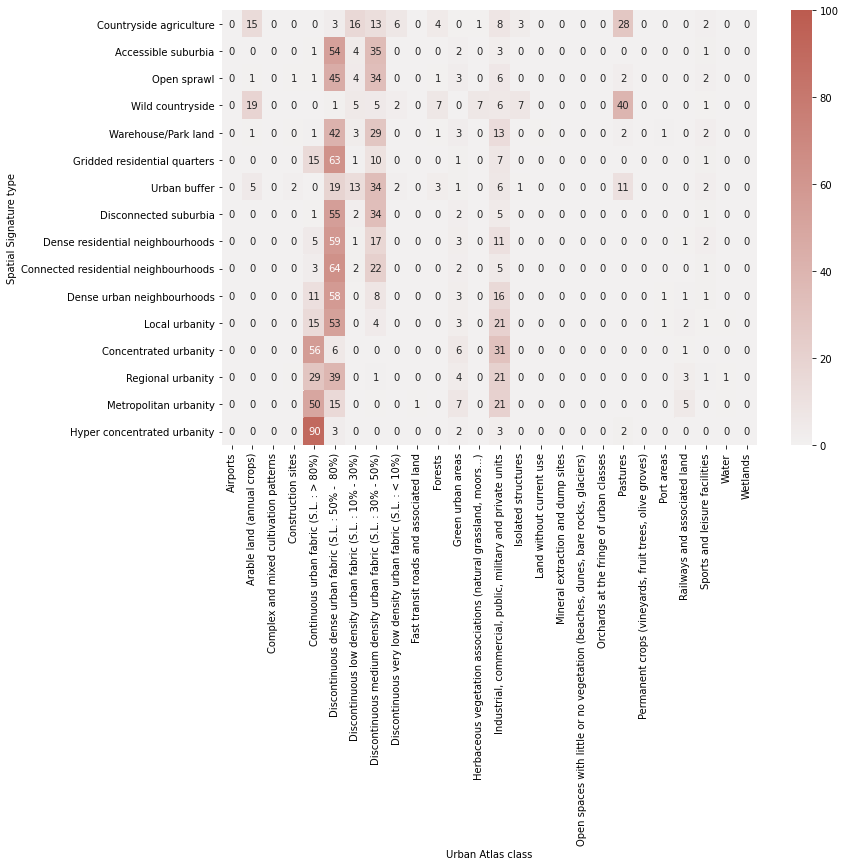
Compute chi-squared
chi = sp.stats.chi2_contingency(crosstab)
print(f"chi2: {chi[0]}, p: {chi[1]}, dof: {chi[2]}, N: {crosstab.sum().sum()}")
chi2: 5229900.007979867, p: 0.0, dof: 450, N: 8396642
/opt/conda/lib/python3.8/site-packages/ipykernel/ipkernel.py:283: DeprecationWarning: `should_run_async` will not call `transform_cell` automatically in the future. Please pass the result to `transformed_cell` argument and any exception that happen during thetransform in `preprocessing_exc_tuple` in IPython 7.17 and above.
and should_run_async(code)
cramers_v(crosstab)
0.18601133182588733
Local Climate Zones¶
Download and read data. https://journals.plos.org/plosone/article?id=10.1371/journal.pone.0214474, https://figshare.com/articles/dataset/European_LCZ_map/13322450
path = download("https://figshare.com/ndownloader/files/28352715",
'../../urbangrammar_samba/spatial_signatures/validation/EU_LCZ_map_epsg4326.tif')
Downloading data from https://s3-eu-west-1.amazonaws.com/pfigshare-u-files/28352715/EU_LCZ_map_epsg4326.tif?X-Amz-Algorithm=AWS4-HMAC-SHA256&X-Amz-Credential=AKIAIYCQYOYV5JSSROOA/20220223/eu-west-1/s3/aws4_request&X-Amz-Date=20220223T104658Z&X-Amz-Expires=10&X-Amz-SignedHeaders=host&X-Amz-Signature=4ab98243608f600c64065a763f57b99cbb32def65f74e32e9975f7b5c341ba99 (113.8 MB)
file_sizes: 100%|████████████████████████████| 119M/119M [00:03<00:00, 38.1MB/s]
Successfully downloaded file to ../../urbangrammar_samba/spatial_signatures/validation/EU_LCZ_map_epsg4326.tif
lcz = rioxarray.open_rasterio(path)
lcz
<xarray.DataArray (band: 1, y: 33718, x: 97031)> [3271691258 values with dtype=int16] Coordinates: * band (band) int64 1 * x (x) float64 -55.24 -55.24 -55.24 -55.24 ... 75.5 75.5 75.5 75.5 * y (y) float64 74.07 74.07 74.07 74.07 ... 28.64 28.64 28.64 28.64 spatial_ref int64 0 Attributes: scale_factor: 1.0 add_offset: 0.0
- band: 1
- y: 33718
- x: 97031
- ...
[3271691258 values with dtype=int16]
- band(band)int641
array([1])
- x(x)float64-55.24 -55.24 -55.24 ... 75.5 75.5
array([-55.244489, -55.243142, -55.241794, ..., 75.49916 , 75.500507, 75.501855])
- y(y)float6474.07 74.07 74.07 ... 28.64 28.64
array([74.073758, 74.07241 , 74.071063, ..., 28.643344, 28.641997, 28.640649])
- spatial_ref()int640
- crs_wkt :
- GEOGCS["WGS 84",DATUM["WGS_1984",SPHEROID["WGS 84",6378137,298.257223563,AUTHORITY["EPSG","7030"]],AUTHORITY["EPSG","6326"]],PRIMEM["Greenwich",0],UNIT["degree",0.0174532925199433,AUTHORITY["EPSG","9122"]],AXIS["Latitude",NORTH],AXIS["Longitude",EAST],AUTHORITY["EPSG","4326"]]
- semi_major_axis :
- 6378137.0
- semi_minor_axis :
- 6356752.314245179
- inverse_flattening :
- 298.257223563
- reference_ellipsoid_name :
- WGS 84
- longitude_of_prime_meridian :
- 0.0
- prime_meridian_name :
- Greenwich
- geographic_crs_name :
- WGS 84
- grid_mapping_name :
- latitude_longitude
- spatial_ref :
- GEOGCS["WGS 84",DATUM["WGS_1984",SPHEROID["WGS 84",6378137,298.257223563,AUTHORITY["EPSG","7030"]],AUTHORITY["EPSG","6326"]],PRIMEM["Greenwich",0],UNIT["degree",0.0174532925199433,AUTHORITY["EPSG","9122"]],AXIS["Latitude",NORTH],AXIS["Longitude",EAST],AUTHORITY["EPSG","4326"]]
- GeoTransform :
- -55.245163095931595 0.0013474837091883192 0.0 74.07443129379674 0.0 -0.0013474837091883192
array(0)
- scale_factor :
- 1.0
- add_offset :
- 0.0
signatures = gpd.read_parquet("../../urbangrammar_samba/spatial_signatures/signatures/signatures_combined_levels_simplified.pq")
Reproject.
box = gpd.GeoSeries([box(*signatures.total_bounds)], crs=signatures.crs).to_crs(lcz.rio.crs)
lcz_osgb = lcz.rio.clip_box(*box.total_bounds).rio.reproject("EPSG:27700")
Get signature type as float.
signatures.signature_type = (signatures.kmeans10gb * 10) + signatures.level2
signatures
kmeans10gb | geometry | level2 | signature_type | |
---|---|---|---|---|
0 | 0 | POLYGON ((62219.999 798499.999, 62109.999 7985... | 0.0 | 0.0 |
1 | 0 | POLYGON ((63507.682 796515.168, 63471.096 7965... | 0.0 | 0.0 |
2 | 0 | POLYGON ((65953.174 802246.171, 65523.864 8023... | 0.0 | 0.0 |
3 | 0 | POLYGON ((67297.740 803435.799, 67220.290 8034... | 0.0 | 0.0 |
4 | 0 | POLYGON ((75760.000 852669.999, 75699.999 8527... | 0.0 | 0.0 |
... | ... | ... | ... | ... |
96699 | 9 | POLYGON ((323321.005 463795.415, 323236.741 46... | 8.0 | 98.0 |
96700 | 9 | POLYGON ((325929.840 1008792.060, 325890.989 1... | 8.0 | 98.0 |
96701 | 9 | POLYGON ((337804.769 1013422.582, 337547.855 1... | 8.0 | 98.0 |
96702 | 9 | POLYGON ((422304.270 1147826.989, 422296.000 1... | 8.0 | 98.0 |
96703 | 9 | POLYGON ((525396.260 439215.479, 525360.919 43... | 8.0 | 98.0 |
96704 rows × 4 columns
Rasterise signatures.
%%time
signatures_raster = make_geocube(signatures, measurements=["signature_type"], like=lcz_osgb)
CPU times: user 17.6 s, sys: 1.1 s, total: 18.7 s
Wall time: 18.6 s
Save raster to file.
signatures_raster.signature_type.rio.to_raster("../../urbangrammar_samba/spatial_signatures/signatures/signatures_raster_lcz.tif")
Create cross tabulation. There may be a smarter way but this one works.
spsig_vals = signatures.signature_type.unique()
lcz_vals = np.unique(lcz_osgb)[1:] # ignoring null
crosstab = pd.DataFrame(columns=lcz_vals, index=spsig_vals)
for sps, so in product(spsig_vals, lcz_vals):
crosstab.loc[sps, so] = np.logical_and(signatures_raster.signature_type == sps, lcz_osgb[0] == so).data.sum()
crosstab
0 | 2 | 3 | 4 | 5 | 6 | 8 | 9 | 10 | 11 | 12 | 13 | 14 | 15 | 16 | 17 | |
---|---|---|---|---|---|---|---|---|---|---|---|---|---|---|---|---|
0.0 | 25077 | 39 | 195 | 0 | 159 | 30504 | 4819 | 101054 | 0 | 618768 | 460846 | 1518 | 5338608 | 4160 | 1167 | 28298 |
10.0 | 765 | 0 | 27 | 0 | 43 | 135527 | 1393 | 1292 | 0 | 1770 | 8372 | 0 | 8474 | 244 | 47 | 384 |
30.0 | 1756 | 6 | 224 | 0 | 733 | 218317 | 12039 | 5126 | 0 | 15052 | 49288 | 4 | 51549 | 368 | 162 | 3391 |
40.0 | 67832 | 25 | 117 | 0 | 101 | 10253 | 628 | 151456 | 0 | 1201136 | 623736 | 57865 | 4146693 | 54835 | 2814 | 115009 |
50.0 | 5314 | 138 | 291 | 36 | 1222 | 76593 | 23508 | 1363 | 43 | 6176 | 26242 | 1 | 27913 | 742 | 89 | 3935 |
60.0 | 329 | 34 | 1130 | 0 | 264 | 13452 | 2284 | 52 | 0 | 64 | 466 | 0 | 196 | 78 | 2 | 110 |
70.0 | 28392 | 84 | 645 | 5 | 899 | 215584 | 18073 | 57667 | 14 | 206451 | 285617 | 417 | 1390348 | 2579 | 1532 | 17996 |
80.0 | 50 | 0 | 24 | 0 | 34 | 47355 | 243 | 182 | 0 | 269 | 1477 | 0 | 334 | 6 | 1 | 36 |
20.0 | 782 | 58 | 1519 | 4 | 1300 | 50885 | 6465 | 233 | 4 | 727 | 3643 | 0 | 869 | 114 | 10 | 779 |
21.0 | 380 | 5 | 438 | 0 | 208 | 33992 | 1180 | 167 | 0 | 304 | 2313 | 0 | 500 | 24 | 20 | 188 |
22.0 | 657 | 271 | 3298 | 5 | 3451 | 21764 | 7107 | 93 | 0 | 179 | 2192 | 0 | 261 | 47 | 8 | 826 |
90.0 | 393 | 566 | 1392 | 0 | 5524 | 4409 | 3278 | 31 | 2 | 20 | 383 | 0 | 35 | 13 | 2 | 298 |
91.0 | 0 | 467 | 0 | 0 | 15 | 0 | 23 | 0 | 0 | 0 | 28 | 0 | 0 | 0 | 0 | 19 |
92.0 | 0 | 920 | 765 | 2 | 2129 | 424 | 825 | 9 | 0 | 12 | 135 | 0 | 1 | 3 | 0 | 169 |
93.0 | 10 | 0 | 0 | 0 | 0 | 0 | 0 | 0 | 0 | 0 | 0 | 0 | 0 | 0 | 0 | 0 |
94.0 | 2 | 552 | 78 | 0 | 332 | 17 | 21 | 0 | 0 | 1 | 117 | 0 | 15 | 1 | 0 | 38 |
95.0 | 9 | 87 | 0 | 0 | 0 | 0 | 0 | 0 | 0 | 0 | 0 | 0 | 59 | 0 | 0 | 1 |
96.0 | 29 | 0 | 0 | 0 | 0 | 0 | 0 | 0 | 0 | 0 | 0 | 0 | 0 | 0 | 0 | 0 |
97.0 | 5 | 0 | 0 | 0 | 0 | 0 | 17 | 0 | 0 | 0 | 0 | 0 | 0 | 0 | 0 | 4 |
98.0 | 27 | 0 | 0 | 0 | 0 | 0 | 0 | 1 | 0 | 0 | 0 | 0 | 0 | 0 | 0 | 1 |
crosstab.columns = [str(c) for c in crosstab.columns]
crosstab.to_parquet("../../urbangrammar_samba/spatial_signatures/validation/ctab_lcz.pq")
crosstab = pd.read_parquet("../../urbangrammar_samba/spatial_signatures/validation/ctab_lcz.pq")
Plot percentages.
types = {
0: "Countryside agriculture",
10: "Accessible suburbia",
30: "Open sprawl",
40: "Wild countryside",
50: "Warehouse/Park land",
60: "Gridded residential quarters",
70: "Urban buffer",
80: "Disconnected suburbia",
20: "Dense residential neighbourhoods",
21: "Connected residential neighbourhoods",
22: "Dense urban neighbourhoods",
90: "Local urbanity",
91: "Concentrated urbanity",
92: "Regional urbanity",
94: "Metropolitan urbanity",
95: "Hyper concentrated urbanity",
}
lczs = {
0:"NA",
1:"Compact highrise",
2:"Compact midrise",
3:"Compact lowrise",
4:"Open highrise",
5:"Open midrise",
6:"Open lowrise",
7:"Lightweight low-rise",
8:"Large lowrise",
9:"Sparsely built",
10:"Heavy Industry",
11 :"Dense trees",
12 :"Scattered trees",
13 :"Bush, scrub",
14 :"Low plants",
15 :"Bare rock or paved",
16 :"Bare soil or sand",
17 :"Water"
}
crosstab = crosstab.drop([x for x in crosstab.index if x not in types.keys()])
crosstab.index = [types[x] for x in crosstab.index]
crosstab.columns = [lczs[int(x)] for x in crosstab.columns]
crosstab
NA | Compact midrise | Compact lowrise | Open highrise | Open midrise | Open lowrise | Large lowrise | Sparsely built | Heavy Industry | Dense trees | Scattered trees | Bush, scrub | Low plants | Bare rock or paved | Bare soil or sand | Water | |
---|---|---|---|---|---|---|---|---|---|---|---|---|---|---|---|---|
Countryside agriculture | 25077 | 39 | 195 | 0 | 159 | 30504 | 4819 | 101054 | 0 | 618768 | 460846 | 1518 | 5338608 | 4160 | 1167 | 28298 |
Accessible suburbia | 765 | 0 | 27 | 0 | 43 | 135527 | 1393 | 1292 | 0 | 1770 | 8372 | 0 | 8474 | 244 | 47 | 384 |
Open sprawl | 1756 | 6 | 224 | 0 | 733 | 218317 | 12039 | 5126 | 0 | 15052 | 49288 | 4 | 51549 | 368 | 162 | 3391 |
Wild countryside | 67832 | 25 | 117 | 0 | 101 | 10253 | 628 | 151456 | 0 | 1201136 | 623736 | 57865 | 4146693 | 54835 | 2814 | 115009 |
Warehouse/Park land | 5314 | 138 | 291 | 36 | 1222 | 76593 | 23508 | 1363 | 43 | 6176 | 26242 | 1 | 27913 | 742 | 89 | 3935 |
Gridded residential quarters | 329 | 34 | 1130 | 0 | 264 | 13452 | 2284 | 52 | 0 | 64 | 466 | 0 | 196 | 78 | 2 | 110 |
Urban buffer | 28392 | 84 | 645 | 5 | 899 | 215584 | 18073 | 57667 | 14 | 206451 | 285617 | 417 | 1390348 | 2579 | 1532 | 17996 |
Disconnected suburbia | 50 | 0 | 24 | 0 | 34 | 47355 | 243 | 182 | 0 | 269 | 1477 | 0 | 334 | 6 | 1 | 36 |
Dense residential neighbourhoods | 782 | 58 | 1519 | 4 | 1300 | 50885 | 6465 | 233 | 4 | 727 | 3643 | 0 | 869 | 114 | 10 | 779 |
Connected residential neighbourhoods | 380 | 5 | 438 | 0 | 208 | 33992 | 1180 | 167 | 0 | 304 | 2313 | 0 | 500 | 24 | 20 | 188 |
Dense urban neighbourhoods | 657 | 271 | 3298 | 5 | 3451 | 21764 | 7107 | 93 | 0 | 179 | 2192 | 0 | 261 | 47 | 8 | 826 |
Local urbanity | 393 | 566 | 1392 | 0 | 5524 | 4409 | 3278 | 31 | 2 | 20 | 383 | 0 | 35 | 13 | 2 | 298 |
Concentrated urbanity | 0 | 467 | 0 | 0 | 15 | 0 | 23 | 0 | 0 | 0 | 28 | 0 | 0 | 0 | 0 | 19 |
Regional urbanity | 0 | 920 | 765 | 2 | 2129 | 424 | 825 | 9 | 0 | 12 | 135 | 0 | 1 | 3 | 0 | 169 |
Metropolitan urbanity | 2 | 552 | 78 | 0 | 332 | 17 | 21 | 0 | 0 | 1 | 117 | 0 | 15 | 1 | 0 | 38 |
Hyper concentrated urbanity | 9 | 87 | 0 | 0 | 0 | 0 | 0 | 0 | 0 | 0 | 0 | 0 | 59 | 0 | 0 | 1 |
order = [
"Wild countryside",
"Countryside agriculture",
"Urban buffer",
"Open sprawl",
"Disconnected suburbia",
"Accessible suburbia",
"Warehouse/Park land",
"Gridded residential quarters",
"Connected residential neighbourhoods",
"Dense residential neighbourhoods",
"Dense urban neighbourhoods",
"Local urbanity",
"Regional urbanity",
"Metropolitan urbanity",
"Concentrated urbanity",
"Hyper concentrated urbanity",
]
crosstab = crosstab.loc[order]
crosstab.columns.name = "LCZ class"
crosstab.index.name = "Spatial Signature type"
fig, ax = plt.subplots(figsize=(8, 8))
sns.heatmap(crosstab.divide(crosstab.sum(axis=1), axis=0).astype('float') * 100, cmap=sns.light_palette(ugg.HEX[2], n_colors=256), annot=True, fmt='.0f', vmax=100)
plt.savefig("crosstab_lcz.pdf", bbox_inches="tight")
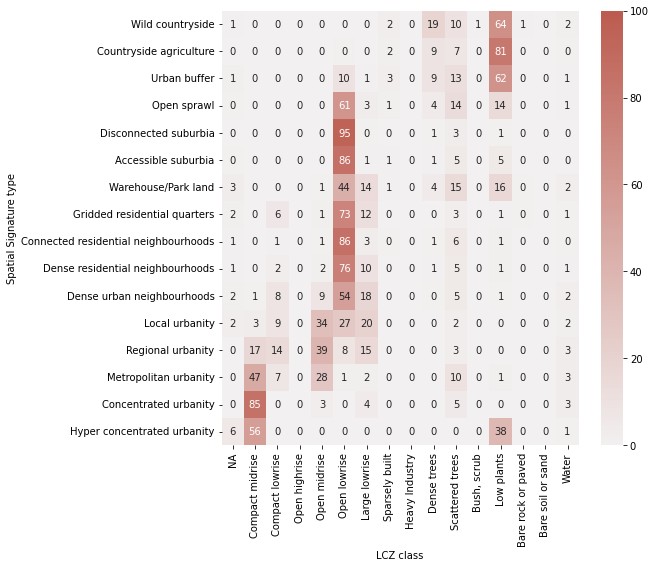
Compute chi-squared
chi = sp.stats.chi2_contingency(crosstab)
print(f"chi2: {chi[0]}, p: {chi[1]}, dof: {chi[2]}, N: {crosstab.sum().sum()}")
chi2: 18467242.8837895, p: 0.0, dof: 225, N: 16203338
Compute Cramer’s V.
cramers_v(crosstab)
0.2756453754603744
Results indicate moderate associtation.